Selecting Subsets of Source Data for Transfer Learning with Applications in Metal Additive Manufacturing
Journal of Intelligent Manufacturing(2024)
Abstract
Considering data insufficiency in metal additive manufacturing (AM), transfer learning (TL) has been adopted to extract knowledge from source domains (e.g., completed printings) to improve the modeling performance in target domains (e.g., new printings). Current applications use all accessible source data directly in TL with no regard to the similarity between source and target data. This paper proposes a systematic method to find appropriate subsets of source data based on similarities between the source and limited target datasets. Such similarity is characterized by the spatial and model distance metrics. A Pareto frontier-based source data selection method is developed, where the source data located on the Pareto frontier defined by two similarity distance metrics are selected iteratively. This method is integrated into an instance-based TL method (decision tree regression model) and a model-based TL method (fine-tuned artificial neural network). Both models are then tested on several regression tasks in metal AM. Comparison results demonstrate that (1) the source data selection method is general and supports integration with various TL methods and distance metrics, (2) compared with using all source data, the proposed method can find a subset of source data from the same domain with better TL performance in metal AM regression tasks involving different processes and machines, and (3) when multiple source domains exist, the source data selection method could find the subset from one source domain to obtain comparable or better TL performance than the model constructed using data from all source domains.
MoreTranslated text
Key words
Metal additive manufacturing,Transfer learning,Source data selection,Pareto frontier
PDF
View via Publisher
AI Read Science
AI Summary
AI Summary is the key point extracted automatically understanding the full text of the paper, including the background, methods, results, conclusions, icons and other key content, so that you can get the outline of the paper at a glance.
Example
Background
Key content
Introduction
Methods
Results
Related work
Fund
Key content
- Pretraining has recently greatly promoted the development of natural language processing (NLP)
- We show that M6 outperforms the baselines in multimodal downstream tasks, and the large M6 with 10 parameters can reach a better performance
- We propose a method called M6 that is able to process information of multiple modalities and perform both single-modal and cross-modal understanding and generation
- The model is scaled to large model with 10 billion parameters with sophisticated deployment, and the 10 -parameter M6-large is the largest pretrained model in Chinese
- Experimental results show that our proposed M6 outperforms the baseline in a number of downstream tasks concerning both single modality and multiple modalities We will continue the pretraining of extremely large models by increasing data to explore the limit of its performance
Try using models to generate summary,it takes about 60s
Must-Reading Tree
Example
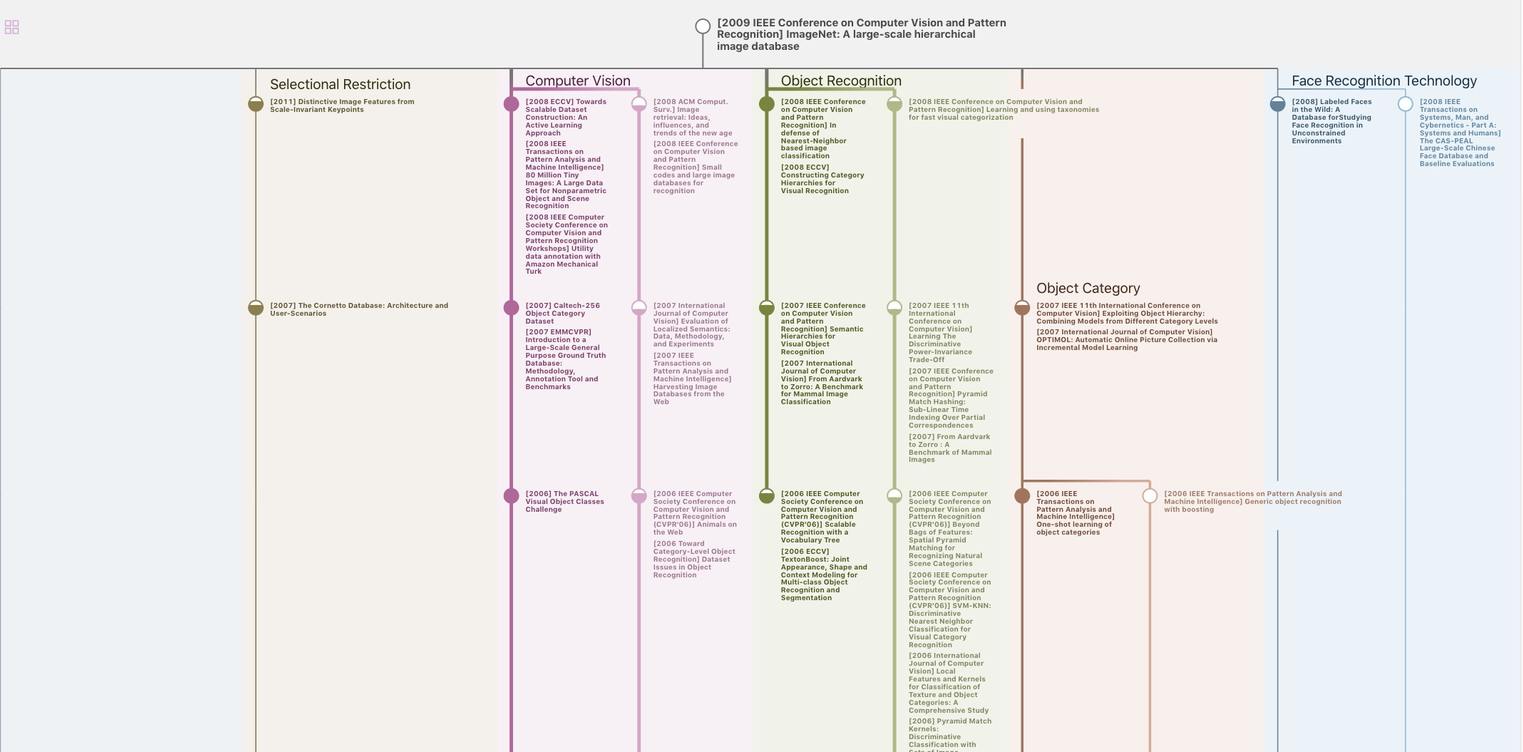
Generate MRT to find the research sequence of this paper
Related Papers
1992
被引用12081 | 浏览
2017
被引用42 | 浏览
2017
被引用31 | 浏览
2018
被引用32 | 浏览
2020
被引用89 | 浏览
2021
被引用69 | 浏览
2021
被引用19 | 浏览
2021
被引用5 | 浏览
2022
被引用76 | 浏览
2022
被引用54 | 浏览
2022
被引用33 | 浏览
2021
被引用13 | 浏览
2022
被引用16 | 浏览
2022
被引用47 | 浏览
2022
被引用42 | 浏览
2024
被引用3 | 浏览
2023
被引用17 | 浏览
2023
被引用6 | 浏览
Data Disclaimer
The page data are from open Internet sources, cooperative publishers and automatic analysis results through AI technology. We do not make any commitments and guarantees for the validity, accuracy, correctness, reliability, completeness and timeliness of the page data. If you have any questions, please contact us by email: report@aminer.cn
Chat Paper
去 AI 文献库 对话