Sample Relationship from Learning Dynamics Matters for Generalisation
CoRR(2024)
摘要
Although much research has been done on proposing new models or loss
functions to improve the generalisation of artificial neural networks (ANNs),
less attention has been directed to the impact of the training data on
generalisation. In this work, we start from approximating the interaction
between samples, i.e. how learning one sample would modify the model's
prediction on other samples. Through analysing the terms involved in weight
updates in supervised learning, we find that labels influence the interaction
between samples. Therefore, we propose the labelled pseudo Neural Tangent
Kernel (lpNTK) which takes label information into consideration when measuring
the interactions between samples. We first prove that lpNTK asymptotically
converges to the empirical neural tangent kernel in terms of the Frobenius norm
under certain assumptions. Secondly, we illustrate how lpNTK helps to
understand learning phenomena identified in previous work, specifically the
learning difficulty of samples and forgetting events during learning. Moreover,
we also show that using lpNTK to identify and remove poisoning training samples
does not hurt the generalisation performance of ANNs.
更多查看译文
关键词
generalisation,data selection,neural tangent kernel,sample interaction,learning dynamics
AI 理解论文
溯源树
样例
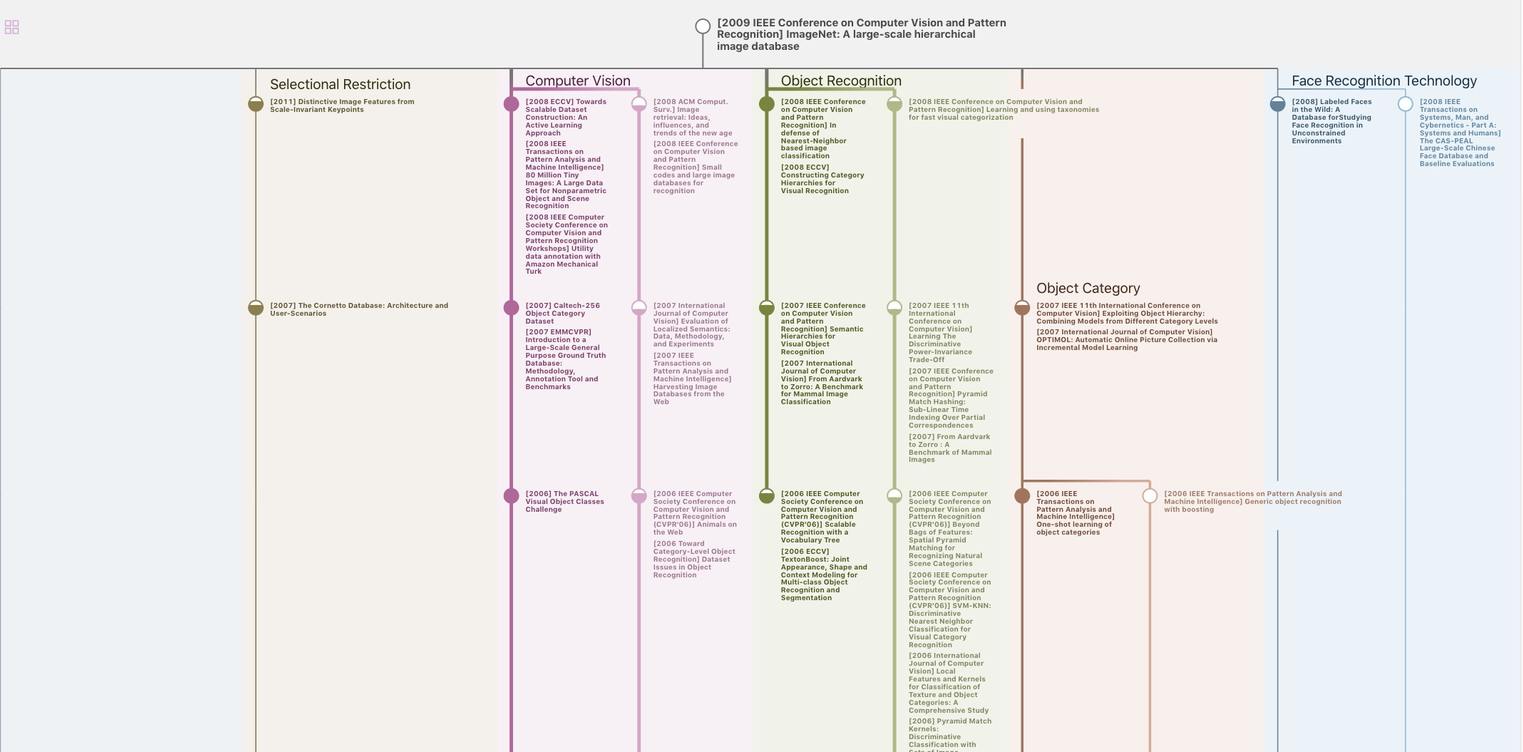
生成溯源树,研究论文发展脉络
Chat Paper
正在生成论文摘要