Toward Diverse Polymer Property Prediction Using Transfer Learning
arxiv(2024)
摘要
The prediction of mechanical and thermal properties of polymers is a critical
aspect for polymer development. Herein, we discuss the use of transfer learning
approach to predict multiple properties of linear polymers. The neural network
model is initially trained to predict the heat capacity in constant pressure
(Cp) of linear polymers. Once, the pre-trained model is transferred to predict
four additional properties of polymers: specific heat capacity (Cv), shear
modulus, flexural stress strength at yield, and tensile creep compliance. They
represent a diverse set of mechanical, thermal, and rheological properties. We
demonstrate the effectiveness of the approach by achieving high accuracy in
predicting the four additional properties using relatively small datasets of 13
to 18 samples. Also, the performance of the base model is examined using five
different loss functions. Our results suggest that the combined loss function
had better performance compared to the individual loss functions.
更多查看译文
AI 理解论文
溯源树
样例
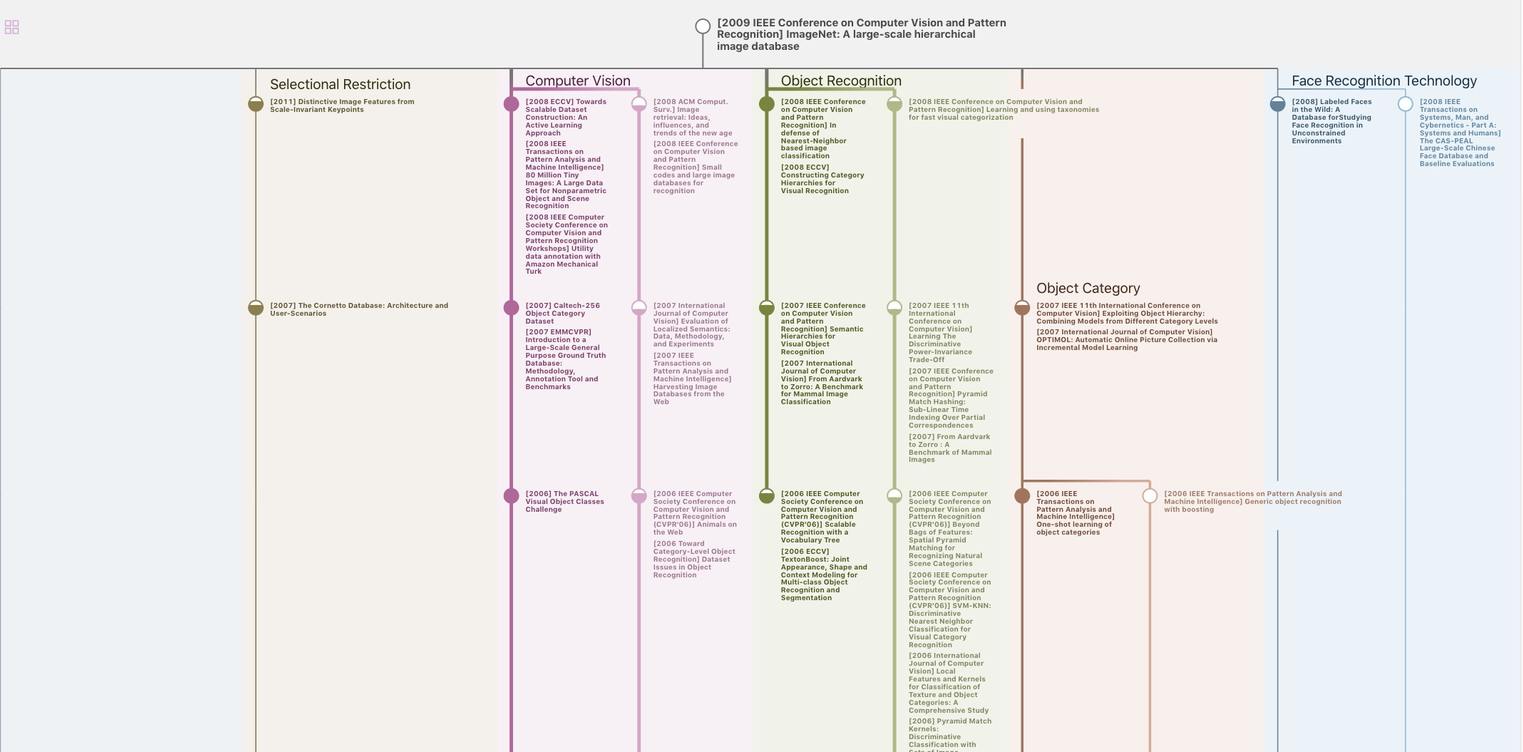
生成溯源树,研究论文发展脉络
Chat Paper
正在生成论文摘要