Approximate Optimal Robust Tracking Control Based on State Error and Derivative Without Initial Admissible Input
IEEE TRANSACTIONS ON SYSTEMS MAN CYBERNETICS-SYSTEMS(2024)
摘要
In this article, a robust tracking control (RTC) problem based on adaptive dynamic programming (ADP) is studied. A cost function based on the state error and derivative is used. A policy iteration (PI) algorithm that can converge is proposed to approximate the solution of the Hamilton-Jacobi- Bellman (HJB) gradually, and the feasibility of the cost function is verified. Since there is no steady-state quadratic term uTRu in the integrated part of the cost function, the boundedness of the cost function can be guaranteed without the discount factor. Unlike traditional ADP based on the discount cost function, it is theoretically proven that the error system can be asymptotically stable if the control input and cost function are iterated to optimal values. Because the error derivative is directly optimized, the excessive change of the error is suppressed. A critic neural network (NN) is used to approximate the optimal cost function. Considering the optimal gradient descent direction of the approximation error, an approximate optimal RTC algorithm without initial admissible input is derived. The difficulties of stability analysis caused by using the error derivative cost function are solved. The PI algorithm is proved to be converged and the error signal is proved to be uniformly ultimately bounded (UUB). Finally, the algorithm is verified to be effective via simulation.
更多查看译文
关键词
Adaptive dynamic programming (ADP),neural network (NN),optimal tracking control (OTC),policy iteration (PI),robust tracking control (RTC)
AI 理解论文
溯源树
样例
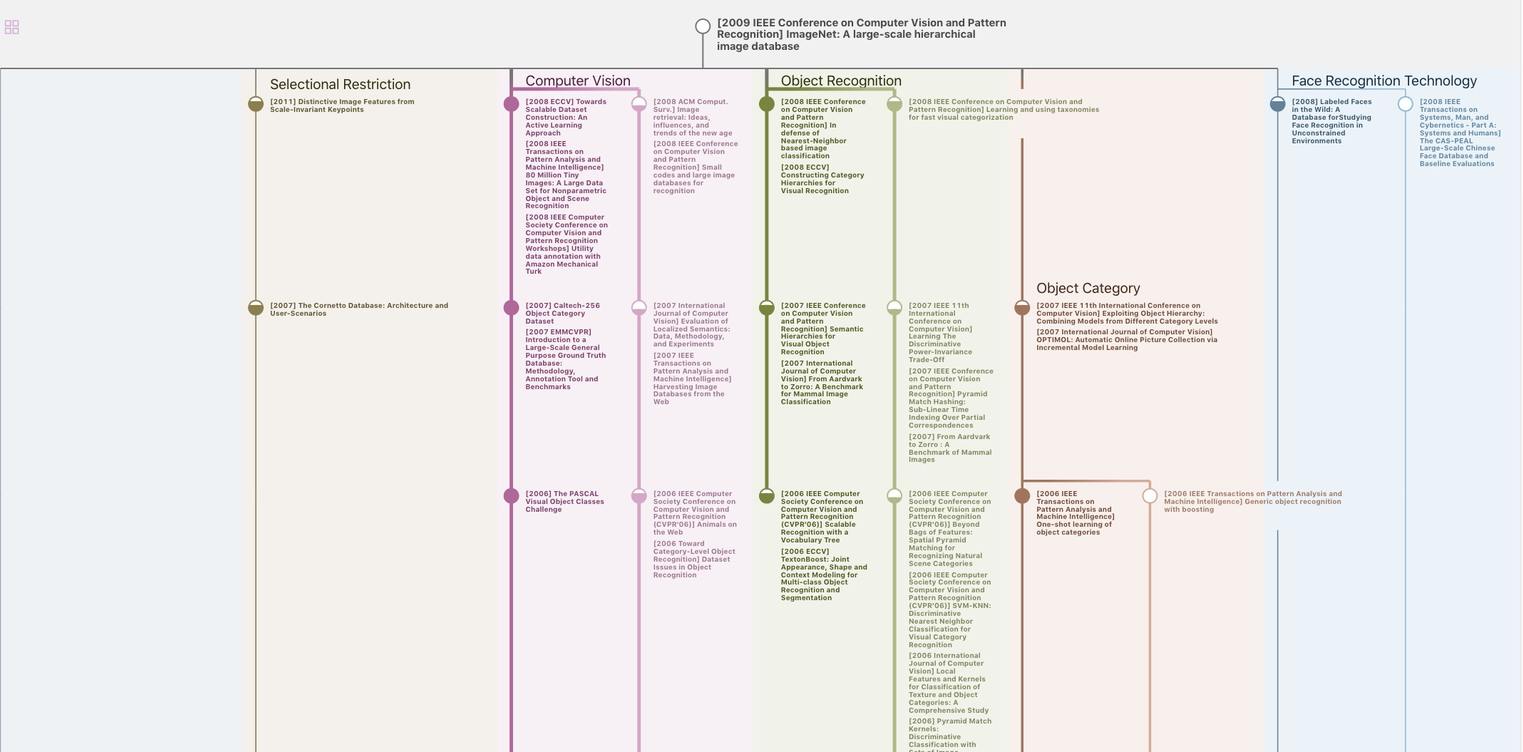
生成溯源树,研究论文发展脉络
Chat Paper
正在生成论文摘要