Tailored Learning Rates for Reinforcement Learning: A Visual Exploration and Guideline Formulation
2023 7th International Symposium on Innovative Approaches in Smart Technologies (ISAS)(2023)
摘要
Reinforcement learning (RL) continues to advance, but the selection of an appropriate learning rate (LR) remains a fundamental challenge. In this study, we conduct a comprehensive exploration and guideline formulation for LR selection in RL. We leverage a diverse set of RL simulations, each featuring a 128-dimensional input vector and a complex 18-dimensional action space, to examine the efficacy of LR adaptation. These simulations encompass a range of RL tasks, emphasizing the relevance of LR selection in various contexts. To gain insights into LR strategies, we utilize two distinct artificial neural networks (ANNs), one with 27,000 hidden layer connections and another with 44,000 connections. We employ a range of tailored LR values, ranging from an initial high of 0.25 to a minuscule 0.000000025. Our experiments focus on the cumulative agent scores across multiple training episodes and the stability of training, where episodes consistently achieve scores close to the average. Our results shed light on LR strategies that optimize both performance and stability across different RL scenarios, offering valuable guidance to researchers and practitioners.
更多查看译文
关键词
Deep Reinforcement Learning,Learning Rates,Neural Network Architecture,Parameter Tuning
AI 理解论文
溯源树
样例
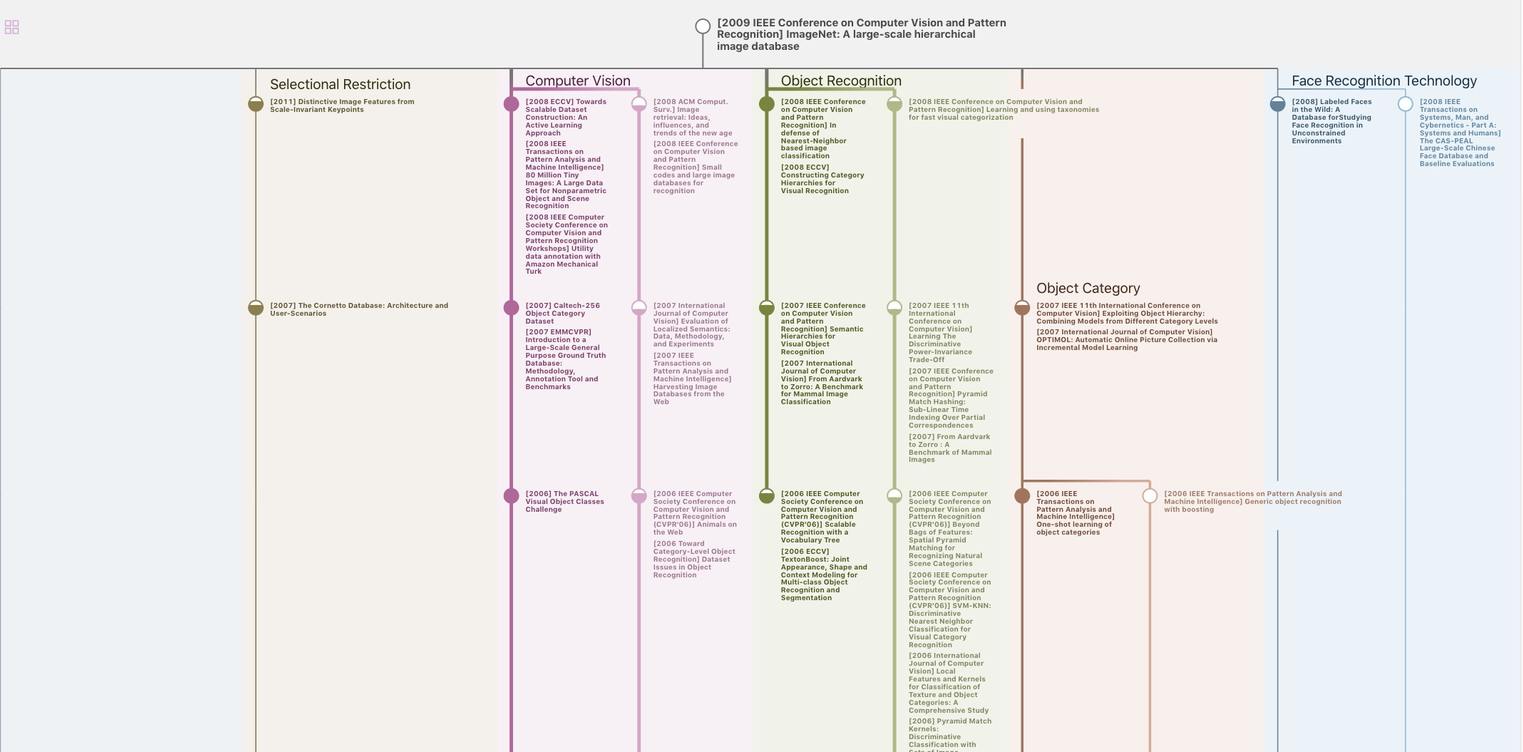
生成溯源树,研究论文发展脉络
Chat Paper
正在生成论文摘要