Accelerating Graph-Based SLAM through Data Parallelism and Mixed Precision on FPGAs
2023 IEEE 16TH INTERNATIONAL SYMPOSIUM ON EMBEDDED MULTICORE/MANY-CORE SYSTEMS-ON-CHIP, MCSOC(2023)
摘要
Simultaneous localization and mapping (SLAM) is a very important application employed in many realistic scenarios, where a mobile robot builds a map of the environment while also using it to locate itself. Within many existing SLAM implementations, graph-based SLAM (G-SLAM) is an intuitive one as graphs are used to represent robot poses, landmarks, and sensor measurements. Obviously, estimating the whole environment and all trajectories through solving such graph problems can incur a large amount of computation and energy consumption. Therefore, in order to speed up G-SLAM within a tight power envelope, we have employed FPGA devices to make use of its energy efficiency and vast data parallelism when inverting the information matrix. In addition, we have also lowered the precision of the information matrix for further reductions in the execution time. With the above attempts, speed-ups of up to 4.5x over general-purpose CPUs can be realized under much smaller power consumption, which has also dramatically improved energy efficiency.
更多查看译文
关键词
G-SLAM,acceleration,parallelism,precision,FPGA
AI 理解论文
溯源树
样例
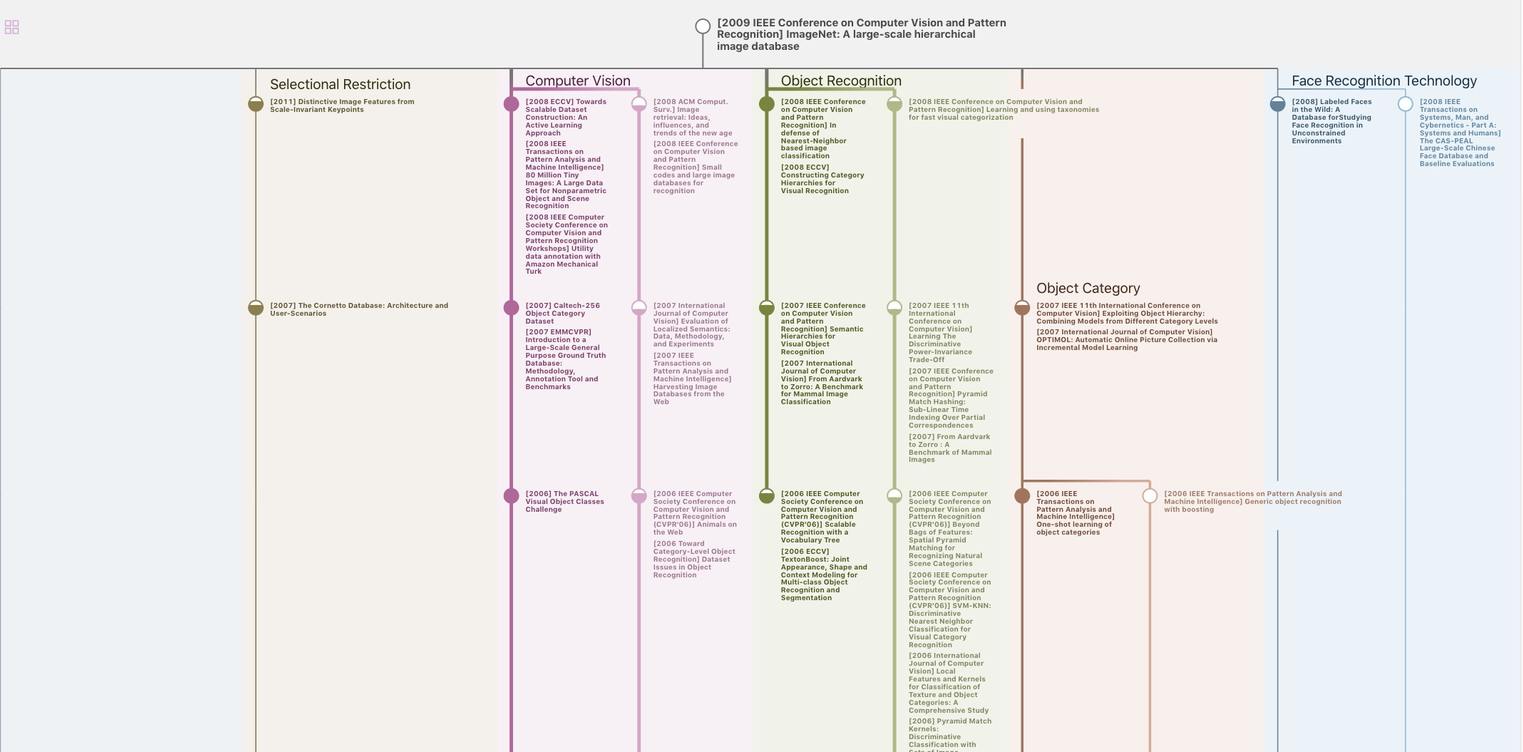
生成溯源树,研究论文发展脉络
Chat Paper
正在生成论文摘要