Adaptable and Interpretable Framework for Anomaly Detection in SCADA-based industrial systems
EXPERT SYSTEMS WITH APPLICATIONS(2024)
摘要
In this paper, we introduce an Adaptable and Interpretable Framework for Anomaly Detection (AID) designed for industrial systems utilizing IoT data streams on top of well -established SCADA systems. AID leverages dynamic conditional probability distribution modeling to capture the normal operation of dynamic systems and isolate the root causes of anomalies at the level of individual inputs. The self -supervised framework dynamically updates parameters of underlying model, allowing it to adapt to non-stationarity. AID interprets anomalies as significant deviations from conditional probability, encompassing interactions as well as both spatial and temporal irregularities by exposing them as features. Crucially, AID provides dynamic operating limits to integrate with existing alarm handling mechanisms in SCADA-based IoT systems. Two industrial -scale case studies demonstrate AID's capabilities. The first study showcases AID's effectiveness on energy storage system, adapting to changes, setting context -aware limits for SCADA, and ability to leverage a physics -based model. The second study monitors battery module temperatures, where AID identifies hardware faults, emphasizing its relevance to energy storage safety. A benchmark evaluation on real data shows that AID delivers comparable performance to other self -learning adaptable anomaly detection methods, with the significant advancement in diagnostic capabilities for improved system reliability and performance.
更多查看译文
关键词
Anomaly detection,Root cause isolation,Iterative learning,Statistical learning,Self-supervised learning
AI 理解论文
溯源树
样例
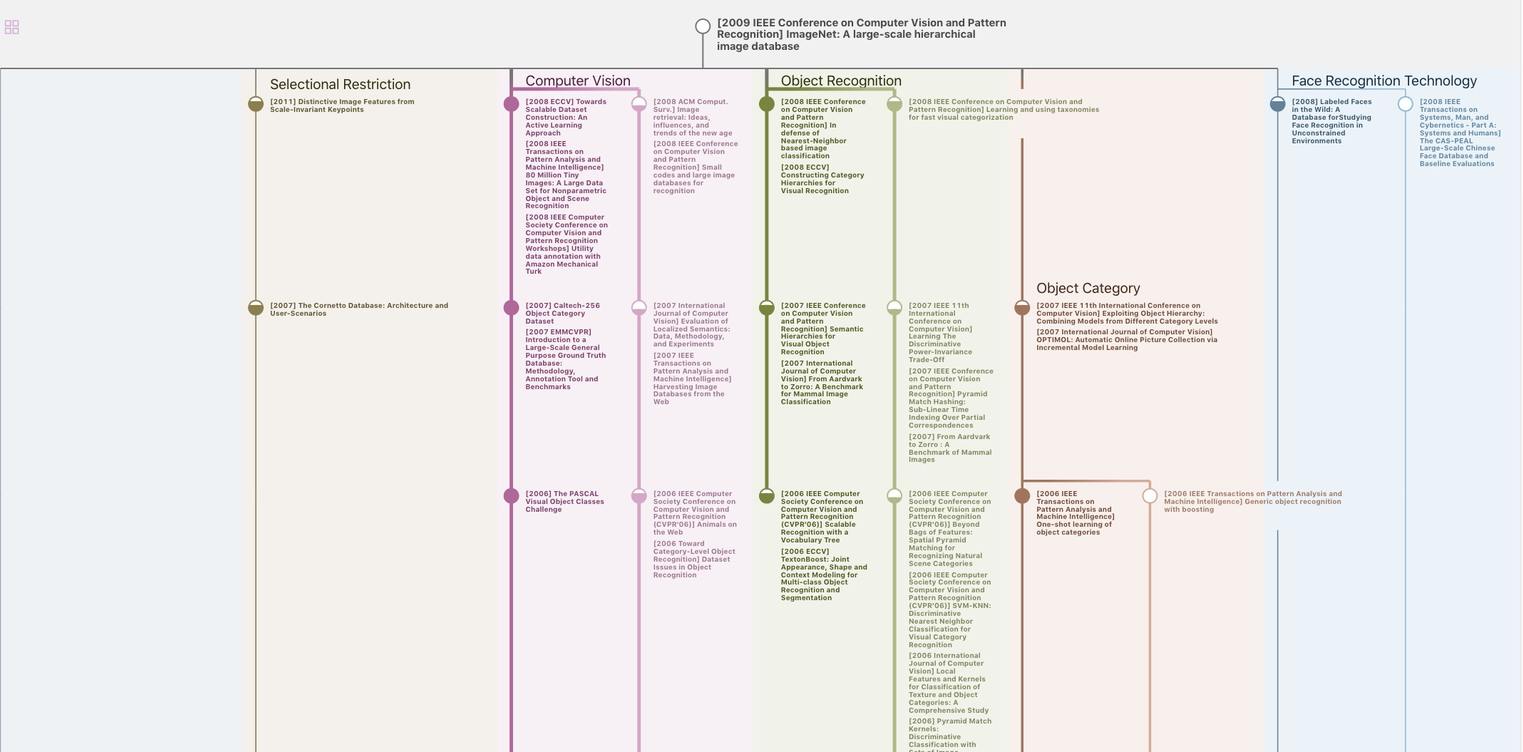
生成溯源树,研究论文发展脉络
Chat Paper
正在生成论文摘要