Spatial-Temporal Federated Transfer Learning with multi-sensor data fusion for cooperative positioning
INFORMATION FUSION(2024)
摘要
With the development of advanced embedded and communication systems, location information has become crucial factor in supporting context -aware or location -aware intelligent services. Among these services, modern Intelligent Transportation System (ITS) has the strictest requirements for real-time, accurate, and private location data. In this study, a Spatial -Temporal Federated Transfer Learning (ST-FTL) framework is designed and introduced to achieve more precise cooperative positioning with multi -sensor data fusion while protecting the location data privacy in urban ITS. Specifically, a three -layer FTL architecture is constructed to enhance the prediction accuracy on GPS positioning errors for vehicles in different regions especially when facing missing local data in some specific scenarios (e.g., urban canyons), in which Transfer Learning (TL) is incorporated to optimize the initialization of global model with faster convergence but less communication cost in Federated Learning (FL). A multi -attribute based spatial -temporal clustering algorithm is developed to facilitate the finding of more appropriate source domains similar to the target domain in a density -based scheme, while convolutional -gated unit is newly designed to further filter out the useless features and add new features based on the convolution operations and gating mechanism, resulting in more effective global model initialization and weight aggregation from cross -region selection. A multi -sensor data fusion model is built in local to improve the prediction accuracy on positioning errors, in which an improved time -aware asymmetric attention mechanism is involved to selectively adjust the weight importance of the incorporated GPS and Inertial Measurement Unit (IMU) data in a more targeted fusion process. Additionally, an improved Siamese network structure, which breaks the traditional dual network structure and only adopts one single network structure, is leveraged to realize the lightweight data augmentation based on the better utilization of historical similar data from local vehicles themselves, when suffering from insufficient training data. Experiments and evaluations based on two different public datasets demonstrate the outstanding performance of our proposed model and method in achieving superior prediction accuracy and faster convergence for cooperative positioning compared with other state-of-the-art positioning methods in urban ITS.
更多查看译文
关键词
Federated Transfer Learning,Siamese Network,Spatial-Temporal Clustering,Data Fusion,Cooperative Positioning
AI 理解论文
溯源树
样例
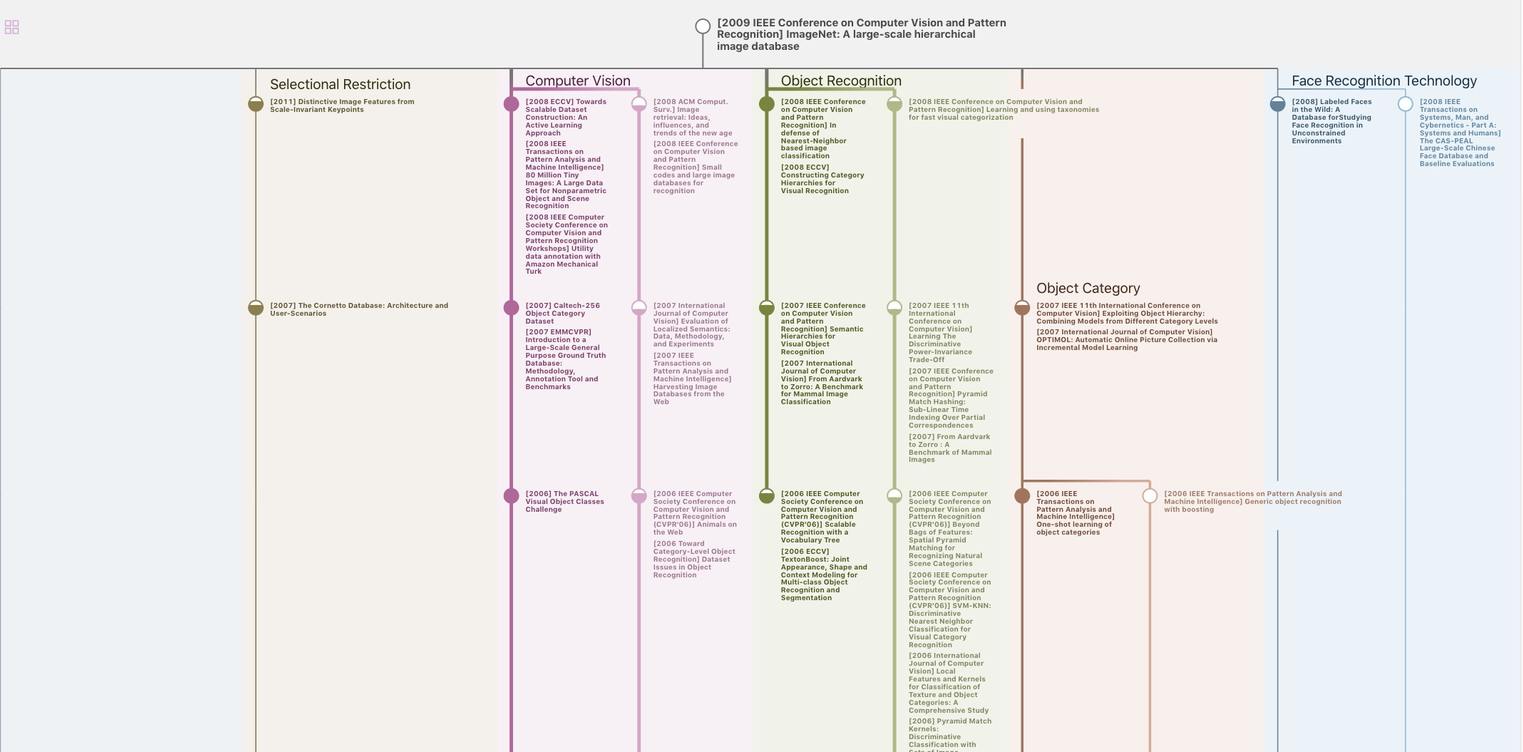
生成溯源树,研究论文发展脉络
Chat Paper
正在生成论文摘要