FedAVE: Adaptive data value evaluation framework for collaborative fairness in federated learning
Neurocomputing(2024)
摘要
Collaborative fairness in federated learning rewards high-contribution clients with high-performance models when multiple clients train a machine learning model cooperatively. Existing approaches ignore the information on data distribution when evaluating the clients’ data quality, resulting in a mismatch between the reward allocation and the real data quality of clients under different data heterogeneity settings. To address this problem, we propose a novel Federated learning framework with Adaptive data Value Evaluation mechanism (FedAVE) to ensure collaborative fairness without affecting the predictive performance of models. First, an adaptive reputation calculation module is designed to generate reputations that match the clients’ contributions based on the information of their data distribution, respectively. Second, a dynamic gradient reward distribution module is devised to allocate a certain number of aggregated model parameter updates/gradients as the rewards corresponding to the reputations and the data distribution information. Extensive experiments on three public benchmarks show that the proposed FedAVE outperforms all baseline methods in terms of fairness, and achieves comparable performance to the state-of-the-art methods in terms of accuracy. Code available at https://github.com/wangzihuixmu/FedAVE.
更多查看译文
关键词
Federated learning,Collaborative fairness,Reputation
AI 理解论文
溯源树
样例
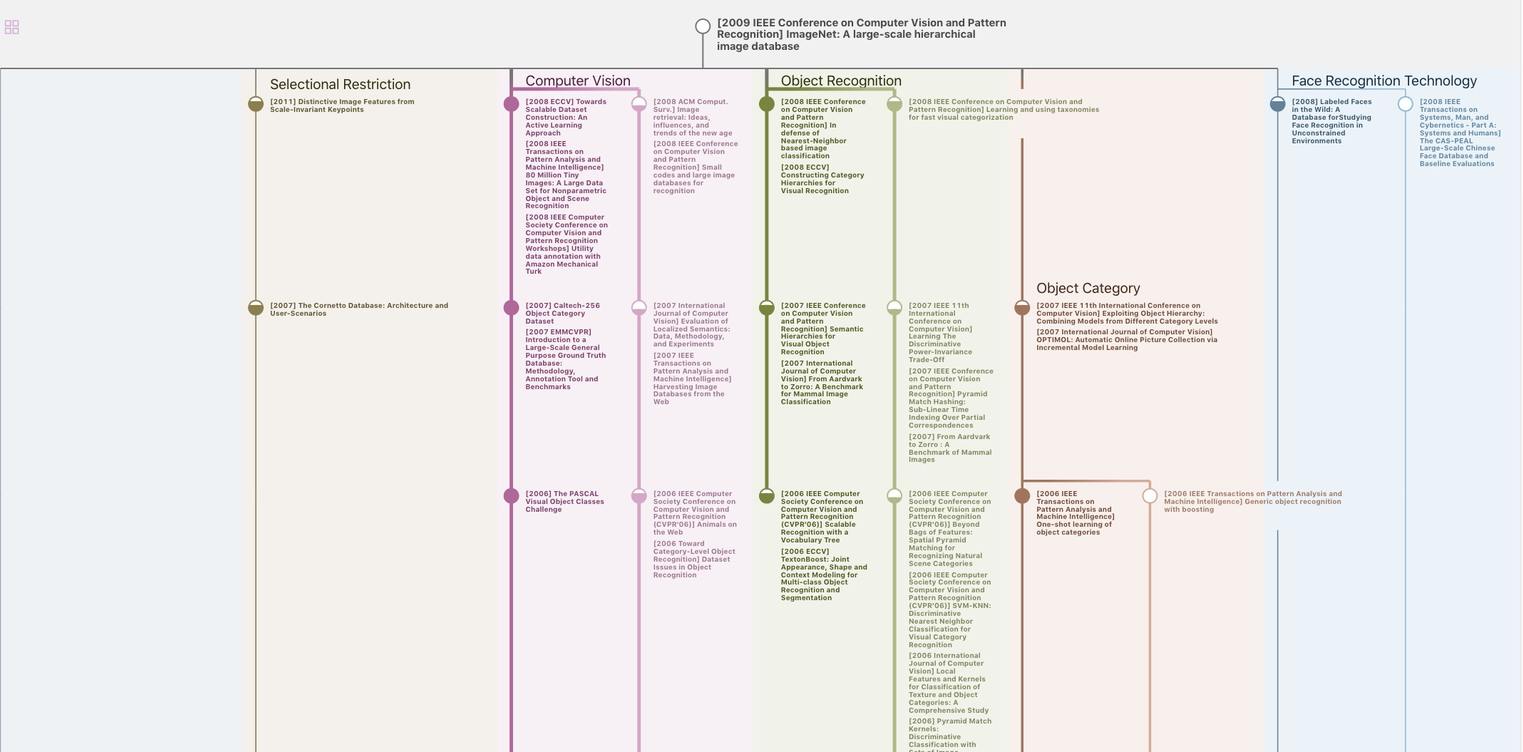
生成溯源树,研究论文发展脉络
Chat Paper
正在生成论文摘要