Censored broken adaptive ridge regression in high-dimension
Computational Statistics(2024)
摘要
Broken adaptive ridge (BAR) is a penalized regression method that performs variable selection via a computationally scalable surrogate to L_0 regularization. The BAR regression has many appealing features; it converges to selection with L_0 penalties as a result of reweighting L_2 penalties, and satisfies the oracle property with grouping effect for highly correlated covariates. In this paper, we investigate the BAR procedure for variable selection in a semiparametric accelerated failure time model with complex high-dimensional censored data. Coupled with Buckley-James-type responses, BAR-based variable selection procedures can be performed when event times are censored in complex ways, such as right-censored, left-censored, or double-censored. Our approach utilizes a two-stage cyclic coordinate descent algorithm to minimize the objective function by iteratively estimating the pseudo survival response and regression coefficients along the direction of coordinates. Under some weak regularity conditions, we establish both the oracle property and the grouping effect of the proposed BAR estimator. Numerical studies are conducted to investigate the finite-sample performance of the proposed algorithm and an application to real data is provided as a data example.
更多查看译文
关键词
Broken adaptive ridge regression,Buckley-James estimator,Accelerated failure time model,Double censoring,Variable selection,Coordinate descent
AI 理解论文
溯源树
样例
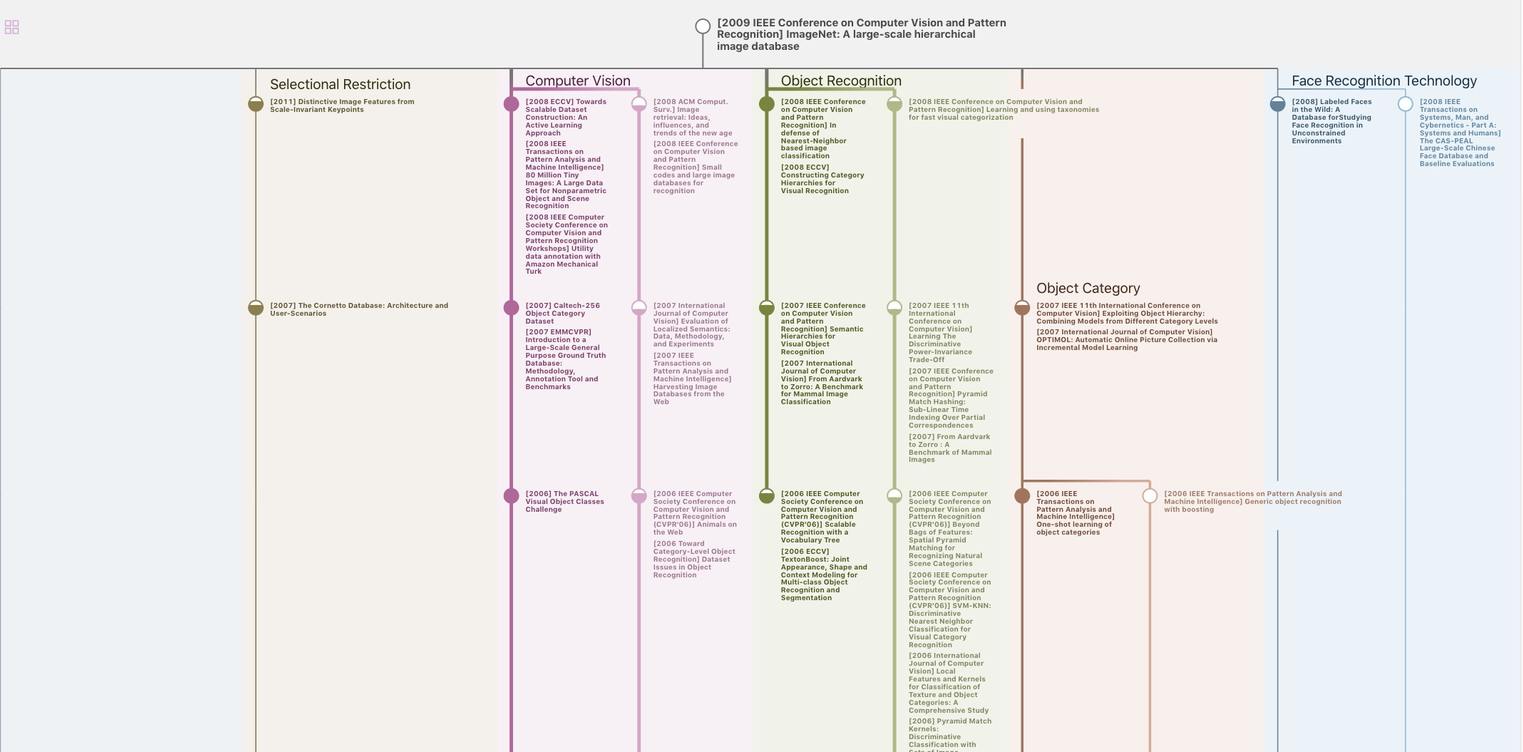
生成溯源树,研究论文发展脉络
Chat Paper
正在生成论文摘要