Estimating Cloud Base Height From All-Sky Imagery Using Artificial Neural Networks
Moscow University Physics Bulletin(2023)
摘要
Cloud Base Height (CBH) is one of the most important meteorological parameters. CBH strongly correlates with the planetary boundary layer depth. Existing methods for assessing CBH in practice either involve the use of complex and expensive equipment, such as lidars, airplanes, and meteorological pilot balloons, or have high uncertainty introduced by an expert in the process of visual assessment. In addition, most of the instrumental methods designed for a stable platform for installing equipment are difficult to apply under conditions of waving. In this study, we propose a new way to estimate CBH using two optical wide-angle cameras mounted at a distance which may vary from 15 m to 30 m. In our approach, we use the phenomenon of parallax, namely, the property of observing a point of a cloud at angles that differ due to the displacement of an observation device. Optical images of the visible sky hemisphere are acquired synchronously during field observations from two cameras of the SAIL Cloud v.2 cloud characteristics assessment device, developed in the Sea-Atmosphere Interaction Laboratory (SAIL) of the Shirshov Institute of Oceanology of the Russian Academy of Sciences. To exploit the parallax phenomenon for closely located objects (clouds), we adjust the transformation of one of the images of each pair to compensate for inaccurate camera installation. To do this, we calculate the correction of the position and orientation of the cameras using distant objects, such as the Sun or the Moon. To find the key points of clouds that are observed from cameras at different angles, the graph-based artificial neural network SuperGlue is used. With the help of SuperGlue, we identify key points and extract their geometric features, according to which we further match key points on synchronous images. Based on the location of key points on synchronously aligned images, we calculate the angle at which they are visible in two cameras, which allows for estimating the distance from the camera installation base to the clouds. As a result of this study, we developed a new algorithm for calculating CBH. We compared the results of CBH estimates with the values of the ERA—5 reanalysis for marine expeditions AI57, AI58, and AI61 of the Shirshov Institute of Oceanology of the Russian Academy of Sciences. The closest consistency with the reanalysis data is observed for cirrus and cumulus clouds.
更多查看译文
关键词
cloud base height,machine learning,deep learning,computer vision,cloud camera,planetary boundary layer
AI 理解论文
溯源树
样例
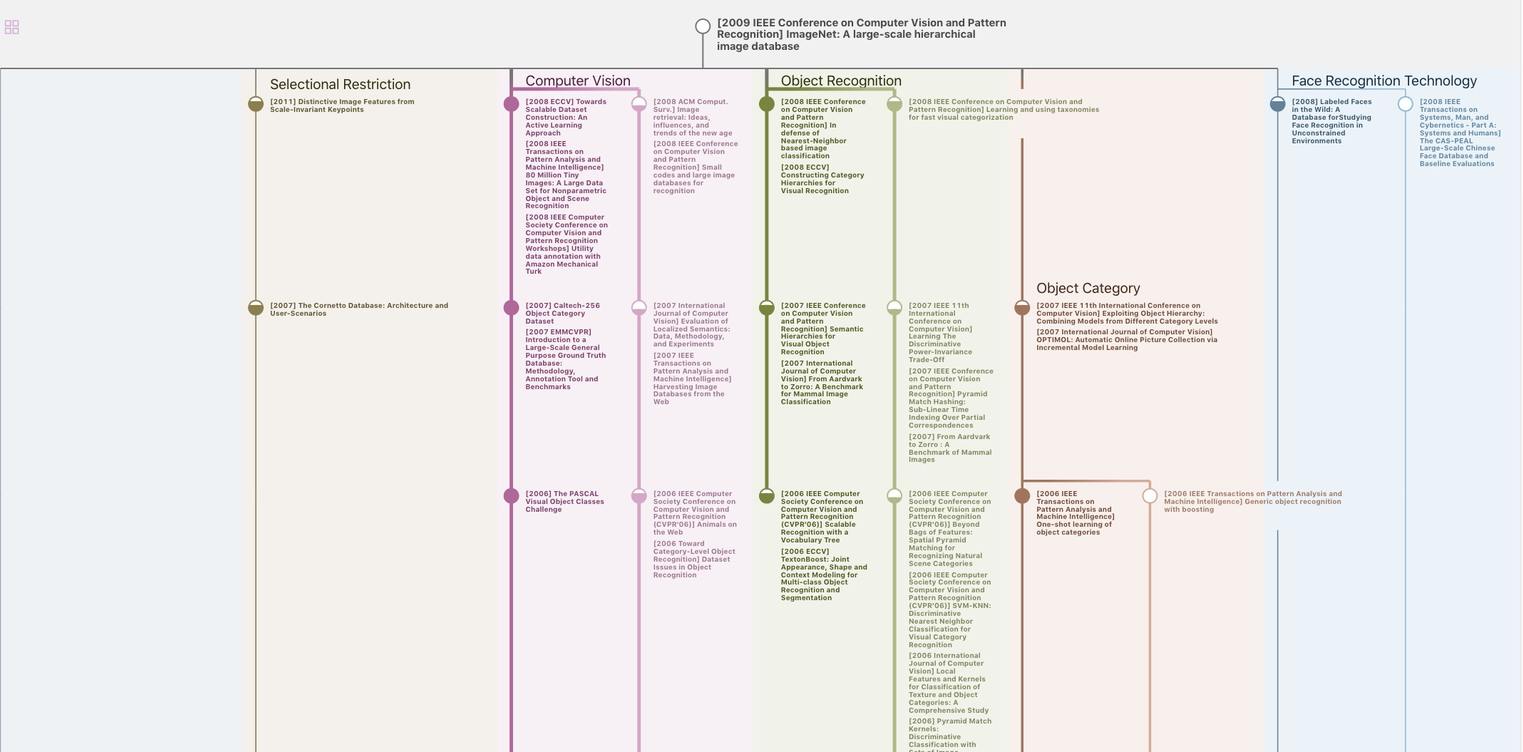
生成溯源树,研究论文发展脉络
Chat Paper
正在生成论文摘要