Machine Learning for Diagnostics of Space Weather Effects for the Arctic Region
Moscow University physics bulletin(2023)
摘要
Despite the variety of existing approaches to monitoring space weather and geophysical parameters in the auroral oval region, the problem of effective prediction and diagnostics of auroras as a special state of the upper ionosphere at high latitudes remains practically open. And another significant problem here is also concerned with diagnostics of geomagnetically induced currents (GICs) in extended grounded technological systems, which are driven by telluric electric fields induced by the rapid changes of the geomagnetic field. The paper is concerned with research on and analysis of possibility of local diagnostics of aurora presence based on the intellectual analysis of geomagnetic data from ground-based sources. The significance of the indicative variables and their statistical relationships are assessed. So, for example, the application of Bayesian inference to the data of the Lovozero geophysical station for 2012–2020 show that the dependence of aposteriori probability of observing auroras in the optical range on the state of geomagnetic parameters is logarithmic, and the degree of significance is inversely proportional to the discrepancy between the empirical data and the approximating function. The accuracy of the approach to diagnostics of aurora presence based on the random forest method is at least 86 % when using several local predictors and 80 % when using several global indices of geomagnetic activity characterizing the disturbance of the geomagnetic field in the auroral zone. In conclusion, promising ways to improve the quality metrics of diagnostic models are considered and the areas of their possible application are discussed. Also the paper is concerned with research on the approach to diagnostics of GIC in the power transmission lines in northwestern Russia based on data from IMAGE magnetometers. Based on the results of the statistical and correlation analysis of the objective function (the level of the GIC recorded at the Vykhodnoy transformer station) and geomagnetic data recorded by the nearby IMAGE magnetometers, the features that best characterize the target variable in a given region are distinguished. Using machine learning (ML) methods, the defined number of feature objects is used to develop the relationship for the GIC diagnostics. Evaluation of the coefficient of determination for a stack of various ML methods revealed that the regression approach and artificial neural networks (ANN) are the best solution for the problem under consideration. Verification tests have shown that ANN-based approach and regression methods provide nearly the same diagnostic accuracy for GIC (the mean square error 0.12 A ^2 ). However, ANN-based methods are less interpretable and require more computer resources.
更多查看译文
关键词
geoinduced currents,aurora,extreme geophysical events diagnostics,machine learning,statistical analysis
AI 理解论文
溯源树
样例
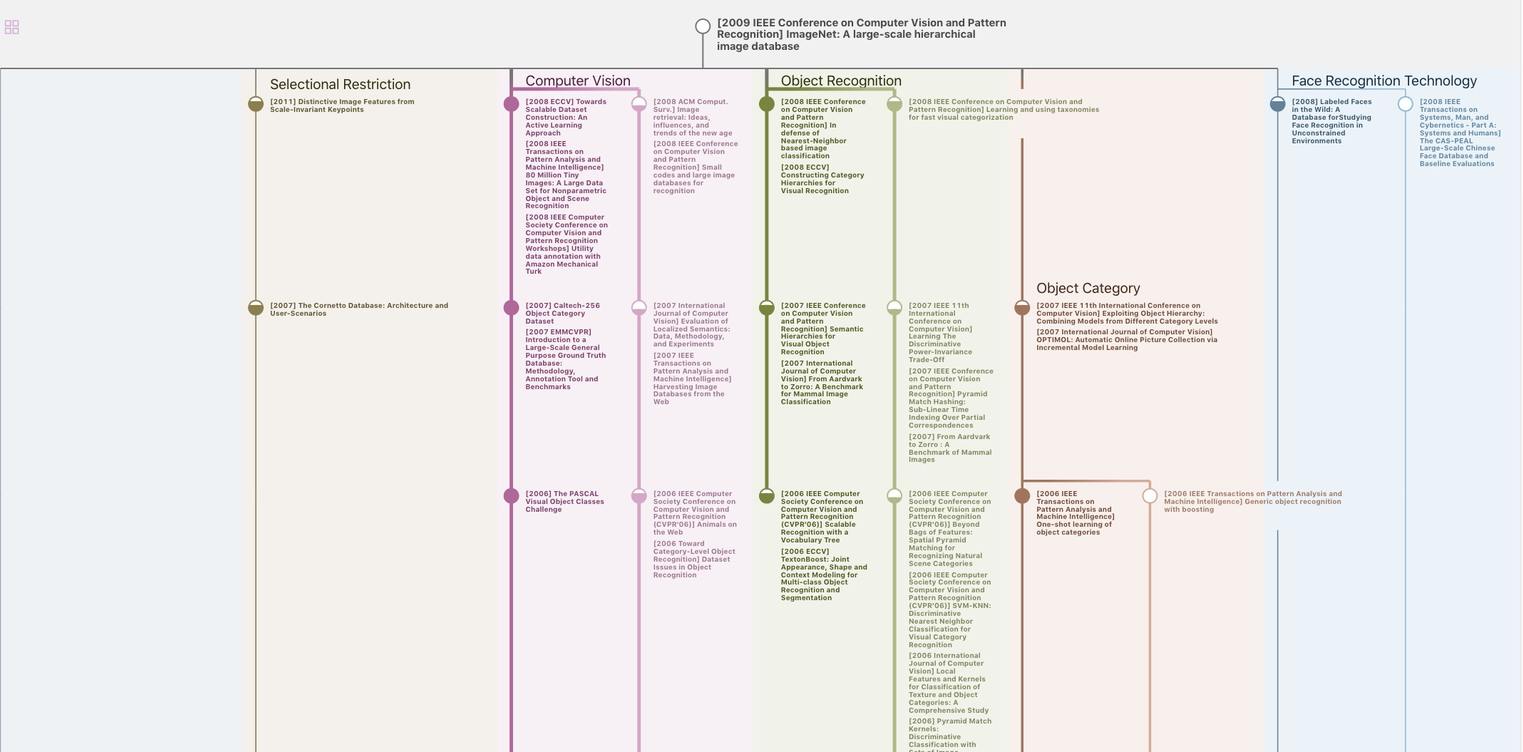
生成溯源树,研究论文发展脉络
Chat Paper
正在生成论文摘要