Phase Identification in Synchrotron X-ray Diffraction Patterns of Ti–6Al–4V Using Computer Vision and Deep Learning
Integrating Materials and Manufacturing Innovation(2024)
摘要
X-ray diffraction patterns contain information about the atomistic structure and microstructure (defect population) of materials, extracting detailed information from diffraction patterns is complex, demanding and relies on prior knowledge. We hypothesize that deep-learning techniques can help to perform an effective and accurate analysis with high throughput rates. To demonstrate this concept, we applied a novel deep learning framework to determine the evolution of the -phase volume fraction in a Ti–6Al–4V alloy during heat-treatment from video sequences of 2D diffraction patterns recorded in transmission and with highly monochromatic radiation in a synchrotron beamline. In particular, we studied the impact of network design on prediction reliability and computational performance. Networks of different architectures were trained using 3008 experimental 2D patterns. A well-tuned model was found to reproduce the phase fractions of another experimental data set, consisting of 1100 diffraction patterns, with a mean-square error as small as 2.6 × 10^-4 . The average prediction error of -phase volume fraction was within 1.6 × 10^-2 (in each diffraction pattern) of the values obtained by conventional methods. Our work demonstrates that convolutional neural networks can evaluate high energy X-ray diffraction patterns with a remarkable level of reliability. Furthermore, it demonstrates the significance of network design on the reliability of predictions and computational performance. The most complex models do not necessarily result in highest accuracy and may even fail to learn from the data.
更多查看译文
关键词
XRD,CNN,Deep learning,Ti–6Al–4V,Phase identification
AI 理解论文
溯源树
样例
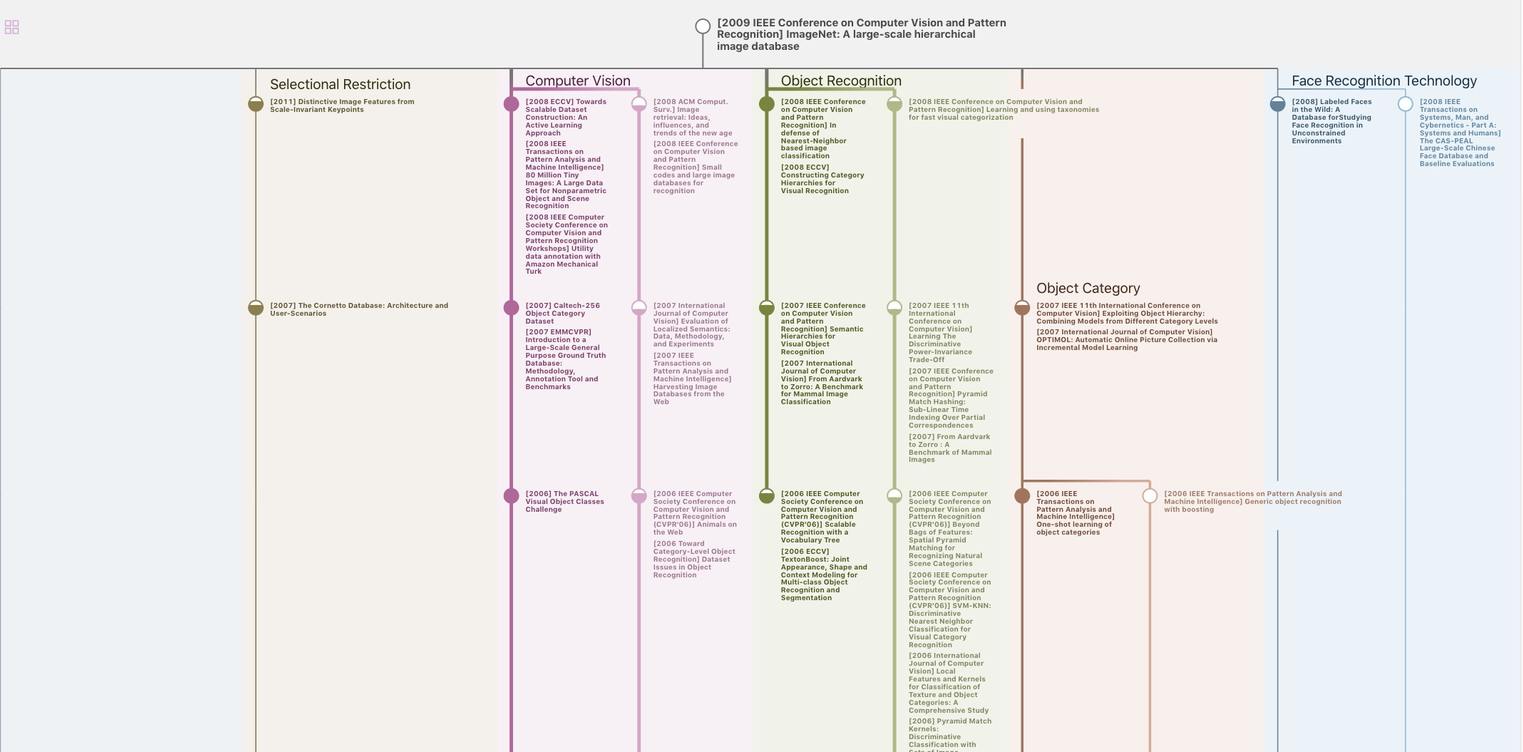
生成溯源树,研究论文发展脉络
Chat Paper
正在生成论文摘要