Fast graph-based denoising for point cloud color information
CoRR(2024)
摘要
Point clouds are utilized in various 3D applications such as cross-reality
(XR) and realistic 3D displays. In some applications, e.g., for live streaming
using a 3D point cloud, real-time point cloud denoising methods are required to
enhance the visual quality. However, conventional high-precision denoising
methods cannot be executed in real time for large-scale point clouds owing to
the complexity of graph constructions with K nearest neighbors and noise level
estimation. This paper proposes a fast graph-based denoising (FGBD) for a
large-scale point cloud. First, high-speed graph construction is achieved by
scanning a point cloud in various directions and searching adjacent
neighborhoods on the scanning lines. Second, we propose a fast noise level
estimation method using eigenvalues of the covariance matrix on a graph.
Finally, we also propose a new low-cost filter selection method to enhance
denoising accuracy to compensate for the degradation caused by the acceleration
algorithms. In our experiments, we succeeded in reducing the processing time
dramatically while maintaining accuracy relative to conventional denoising
methods. Denoising was performed at 30fps, with frames containing approximately
1 million points.
更多查看译文
关键词
point cloud denoising,fast graph construction,real-time denoising,noise estimation,graph signal processing
AI 理解论文
溯源树
样例
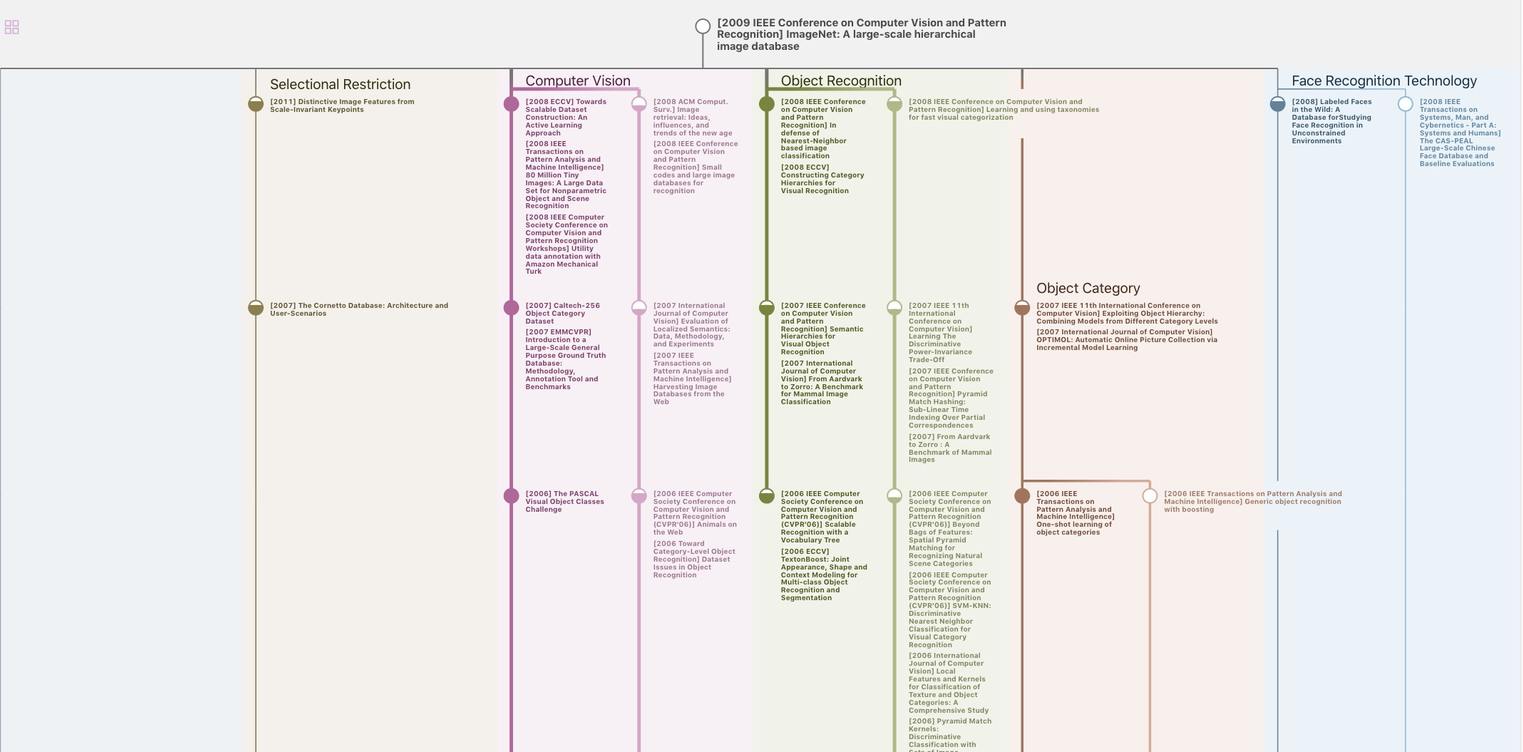
生成溯源树,研究论文发展脉络
Chat Paper
正在生成论文摘要