Cardiac Digital Twin Pipeline for Virtual Therapy Evaluation
CoRR(2024)
摘要
Cardiac digital twins are computational tools capturing key functional and
anatomical characteristics of patient hearts for investigating disease
phenotypes and predicting responses to therapy. When paired with large-scale
computational resources and large clinical datasets, digital twin technology
can enable virtual clinical trials on virtual cohorts to fast-track therapy
development. Here, we present an automated pipeline for personalising
ventricular anatomy and electrophysiological function based on routinely
acquired cardiac magnetic resonance (CMR) imaging data and the standard 12-lead
electrocardiogram (ECG). Using CMR-based anatomical models, a sequential
Monte-Carlo approximate Bayesian computational inference method is extended to
infer electrical activation and repolarisation characteristics from the ECG.
Fast simulations are conducted with a reaction-Eikonal model, including the
Purkinje network and biophysically-detailed subcellular ionic current dynamics
for repolarisation. For each patient, parameter uncertainty is represented by
inferring a population of ventricular models rather than a single one, which
means that parameter uncertainty can be propagated to therapy evaluation.
Furthermore, we have developed techniques for translating from reaction-Eikonal
to monodomain simulations, which allows more realistic simulations of cardiac
electrophysiology. The pipeline is demonstrated in a healthy female subject,
where our inferred reaction-Eikonal models reproduced the patient's ECG with a
Pearson's correlation coefficient of 0.93, and the translated monodomain
simulations have a correlation coefficient of 0.89. We then apply the effect of
Dofetilide to the monodomain population of models for this subject and show
dose-dependent QT and T-peak to T-end prolongations that are in keeping with
large population drug response data.
更多查看译文
AI 理解论文
溯源树
样例
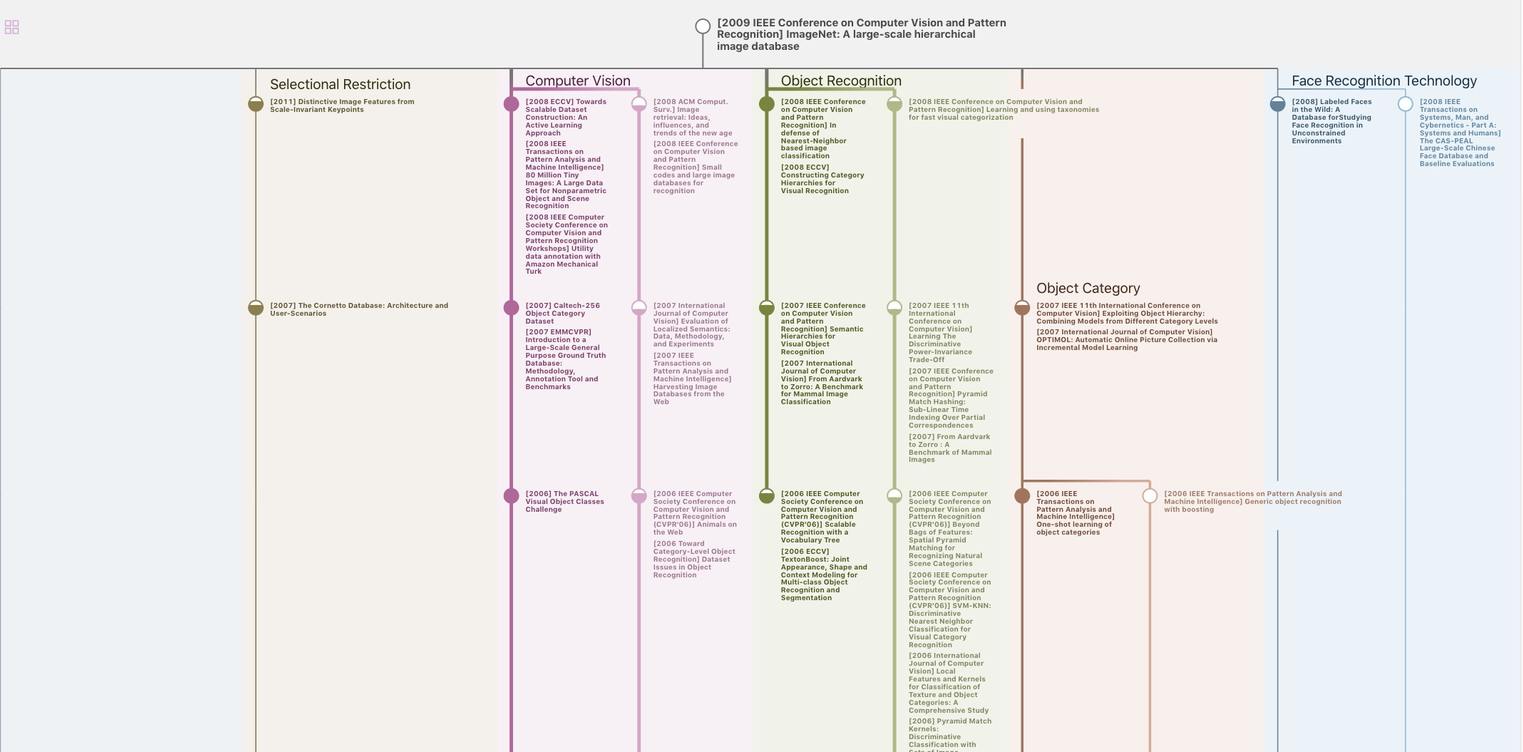
生成溯源树,研究论文发展脉络
Chat Paper
正在生成论文摘要