GPU Acceleration of a Conjugate Exponential Model for Cancer Tissue Heterogeneity
ACM Transactions on Computing for Healthcare(2024)
摘要
Heterogeneity in the cell population of cancer tissues poses many challenges
in cancer diagnosis and treatment. Studying the heterogeneity in cell
populations from gene expression measurement data in the context of cancer
research is a problem of paramount importance. In addition, reducing the
computation time of the algorithms that deal with high volumes of data has its
obvious merits. Parallelizable models using Markov chain Monte Carlo methods
are typically slow. This paper shows a novel, computationally efficient, and
parallelizable model to analyze heterogeneity in cancer tissues using GPUs.
Because our model is parallelizable, the input data size does not affect the
computation time much, provided the hardware resources are not exhausted. Our
model uses qPCR (quantitative polymerase chain reaction) gene expression
measurements to study heterogeneity in cancer tissue. We compute the cell
proportion breakup by accelerating variational methods on a GPU. We test this
model on synthetic and real-world gene expression data collected from
fibroblasts and compare the performance of our algorithm with those of MCMC and
Expectation Maximization. Our new model is computationally less complex and
faster than existing Bayesian models for cancer tissue heterogeneity.
更多查看译文
关键词
CUDA,graphics processing unit,hierarchical model and heterogeneity
AI 理解论文
溯源树
样例
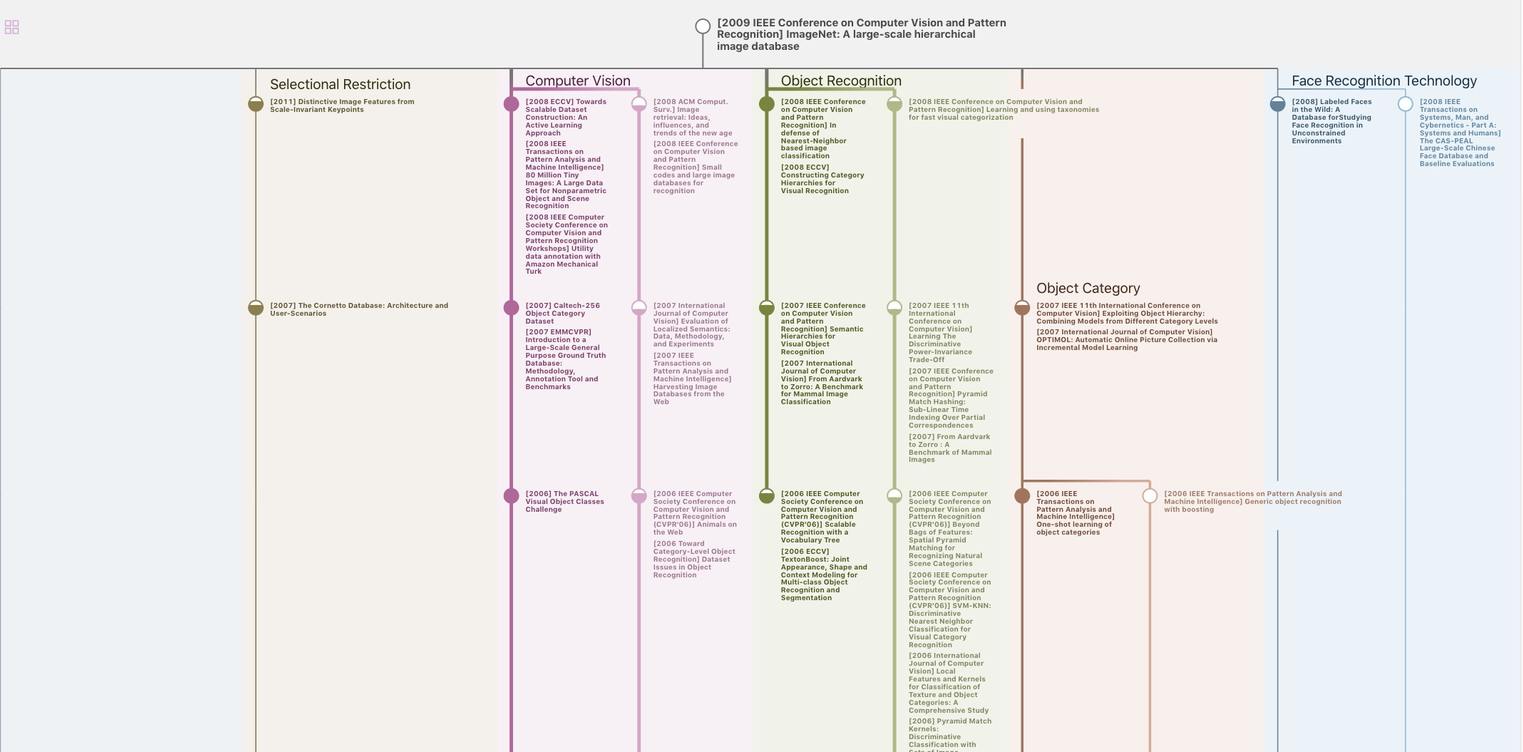
生成溯源树,研究论文发展脉络
Chat Paper
正在生成论文摘要