Predicting photovoltaic efficiency in Cs-based perovskite solar cells: A comprehensive study integrating SCAPS simulation and machine learning models
SOLID STATE COMMUNICATIONS(2024)
摘要
Conventional perovskite-based solar cells (PSCs) have emerged as promising candidates for next -generation solar energy due to their remarkable features, including a high absorption coefficient, tunable bandgaps, high mobility, low maintenance cost, and high power conversion efficiency (PCE). However, the major bottleneck in commercialization of conventional PSCs is their poor stability (of few days), and toxicity concerns (due to lead content). To address these challenges cesium -based perovskites are widely adopted by researchers. However, detailed understanding of these devices considering several device parameters and their connection with overall PCE is not comprehensively disclosed in previous findings. Therefore, in this study, the PV performance of six (6) different PSCs with Cs -based absorber layer (CAL) viz. CsPbI3, CsPbBr3, CsSnCl3, CsSnI3, Cs2AgBiBr6 and CsSn0.5Ge0.5I3 has been investigated through SCAPS simulator, followed by developing few machine learning models to forecast the efficiency. Total 2160 dataset has been obtained by varying the absorber layer, thickness, and doping and defect density for training and testing the five different machine learning algorithms such as linear regression (LR), support vector regression (SVR), neural network (NN), random forest (RF), and XGBoost (XGB). The XGB algorithm outperforms other approaches, achieving an impressive R2 of 99.99 % and low MSE of 0.0006. Impact of each input variable on the efficiency is also obtained by generating SHAP plot for each model which revealed that absorber layer and it thickness variation greatly affected the PCE and least impact of doping is observed on PCE. Among all the absorbers, CsPbI3 shows promising performance by delivering a maximum PCE of 14.00 %. Results reported in this work along with developed ML models may pave the way in the development of Cs based PSCs without the need of complex device simulations.
更多查看译文
关键词
Metal halide perovskite,Simulations,Solar cell,Machine learning,Photovoltaic
AI 理解论文
溯源树
样例
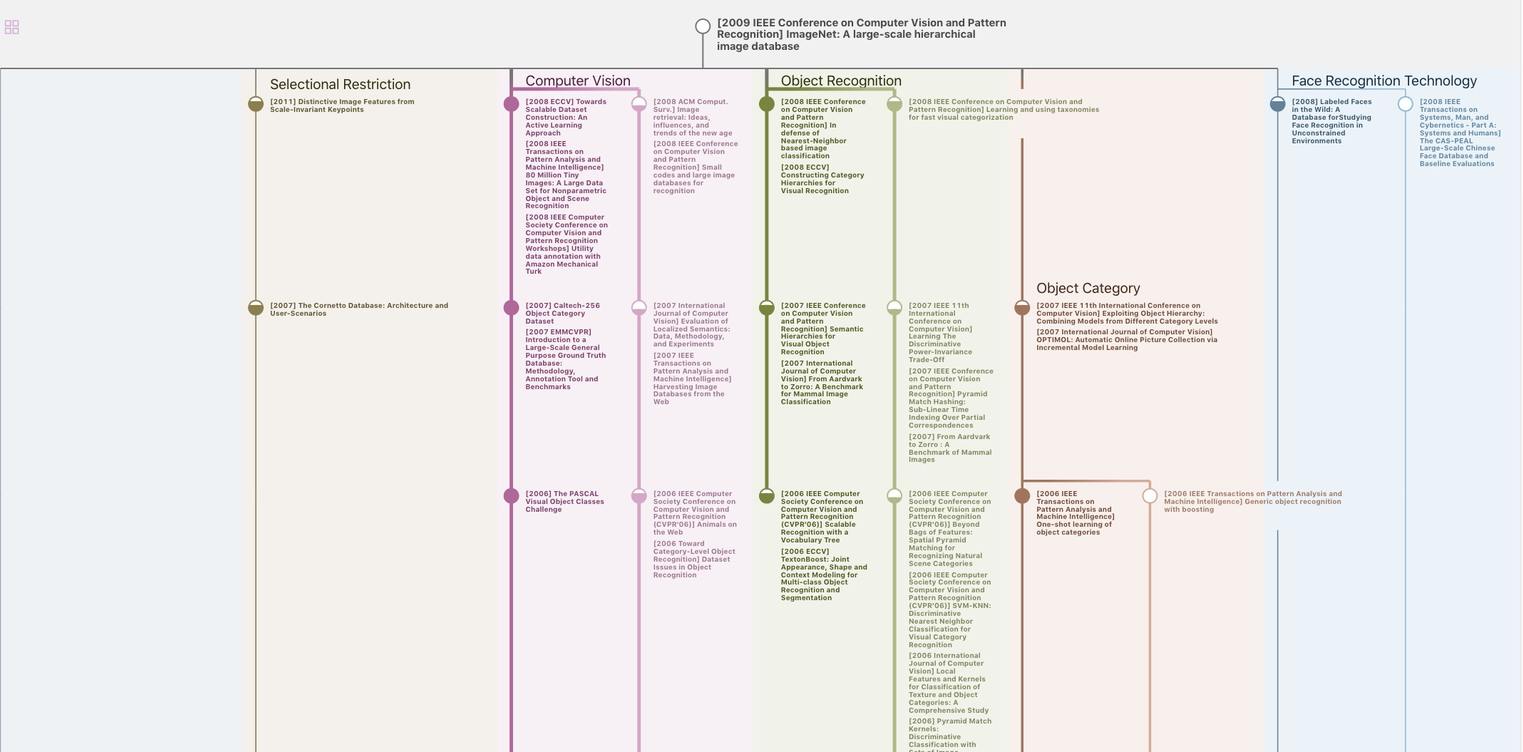
生成溯源树,研究论文发展脉络
Chat Paper
正在生成论文摘要