Gas/Odor Qualitative and Quantitative Estimation Methodology for a Bionic Electronic Nose.
2023 IEEE Biomedical Circuits and Systems Conference (BioCAS)(2023)
摘要
The electronic nose (E-nose) efficiently detects the composition and concentration of gases within a remarkably short span, enabling the identification of volatile metabolites emitted by the human body. However, Traditional methods for both qualitative and quantitative gas estimation face challenges in efficiently employing real-time signals from electronic noses, thereby resulting in imprecise online gas qualitative and quantitative estimation. To address this limitation, our research introduces a cascaded approach that integrates two models: the Muti-scale Wavelet Coefficient Image-Capsule Network (MWCI-CapsNet) for gas identification and the weighted real-time signal-cosformer (weighted-cosformer) for concentration estimation. This approach directly utilizes the real-time signal data from the E-nose as input, eliminating the need for signal pre-processing. By conducting experiments aimed at identifying CO, H
2
, and mixed gas compositions of CO and H
2
using our custom-developed bionic electronic nose, our proposed MWCI-CapsNet model achieves a remarkable accuracy approaching 100% in gas recognition while employing a reduced number of annotated data samples. Furthermore, the weighted-cosformer model demonstrates superior performance when contrasted with the conventional gas quantitative estimation model.
更多查看译文
关键词
electronic nose,gas identification,Capsule Network,wavelet coefficient
AI 理解论文
溯源树
样例
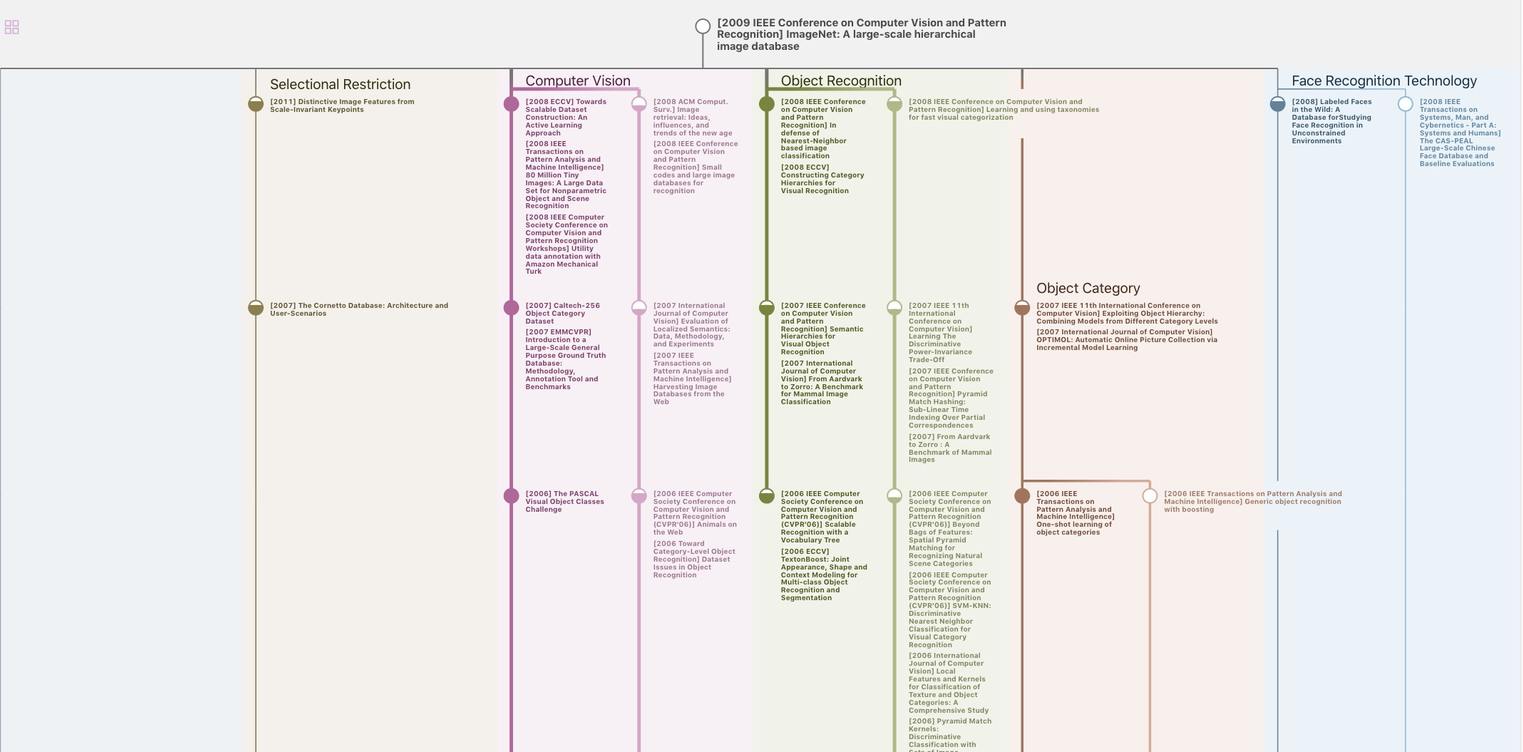
生成溯源树,研究论文发展脉络
Chat Paper
正在生成论文摘要