Mutual Graph Learning Network and Diffusion Probabilistic Model-based Medical Image Segmentation.
2023 IEEE International Conference on Bioinformatics and Biomedicine (BIBM)(2023)
摘要
Diffusion probabilistic models (DPM) can generate semantically valuable pixel-level representations and are widely used in medical image segmentation tasks. However, DPM faces challenges when dealing with medical image segmentation problems due to the irregular structure of medical images and the similarity between lesions and their surrounding environments. Therefore, this paper proposes a dual-branch Diff-UNet architecture to solve the medical image segmentation problem. Specifically, this architecture introduces the Transformer internal network on top of the standard UNet architecture based on DPM and realizes the interaction of UNet and Transformer branch features through bidirectional connection units to capture local features and remote dependencies better. In addition, through the feature fusion module (FFM), the global context information extracted by DPM is combined with the local detail features captured by the segmentation network. Simultaneously, this paper introduces a mutual graph learning (MGL) network to decompose the image into two task-specific feature maps, which are used to roughly locate the object position and capture the fine details of the object boundary. Finally, the cross attention (CA) module combines the edge information of the diffusion model with the features of the segmentation network to enhance the network’s ability to perceive images. Experiments demonstrate the effectiveness of our Diff-UNet on challenging datasets, including self-collected databases and LUNA16.
更多查看译文
关键词
Diffusion probability model,medical segmentation,mutual graph learning network,feature fusion module
AI 理解论文
溯源树
样例
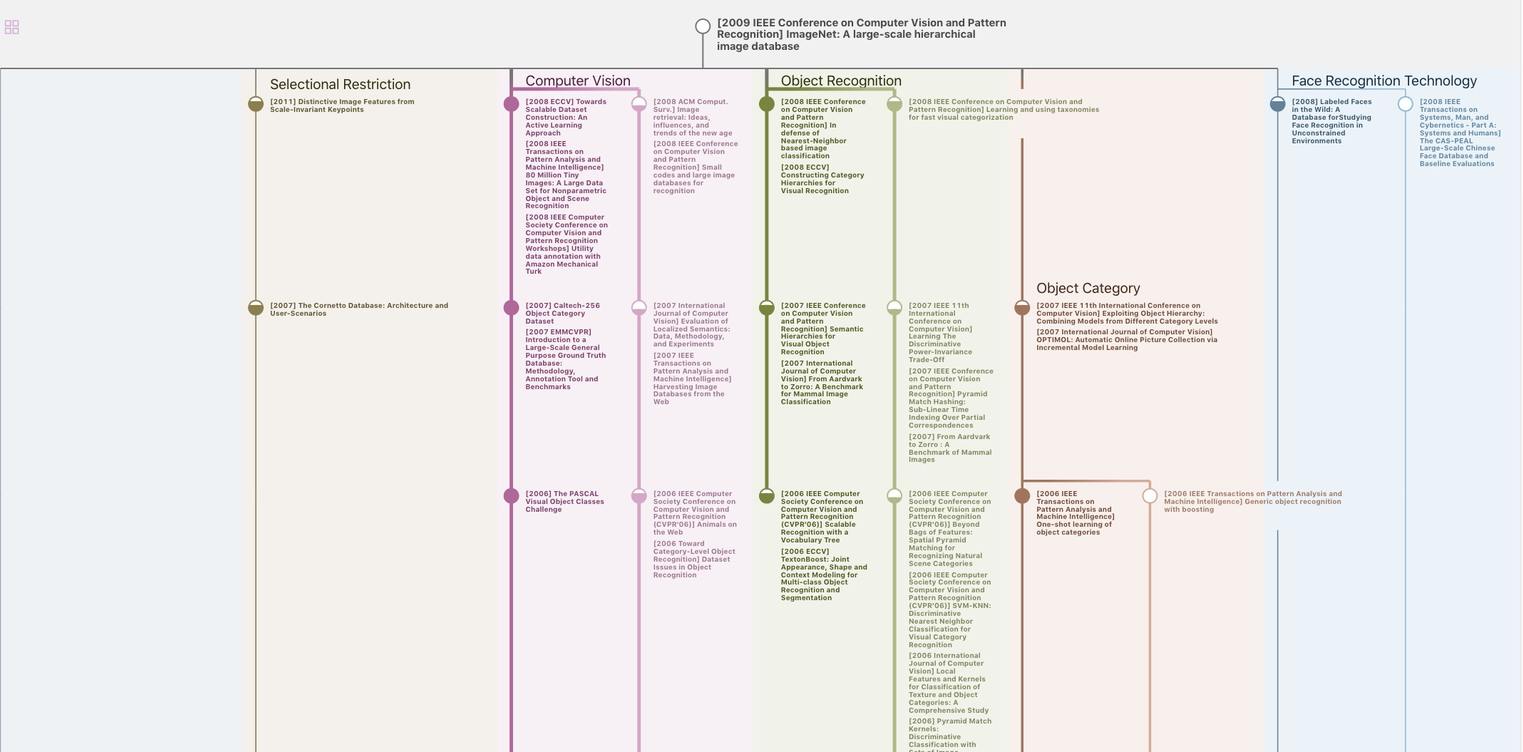
生成溯源树,研究论文发展脉络
Chat Paper
正在生成论文摘要