A Closer Look at Weak Supervision's Limitations in WSI Recurrence Score Prediction.
2023 IEEE International Conference on Bioinformatics and Biomedicine (BIBM)(2023)
摘要
Histological examination remains the gold standard for breast cancer diagnosis, prognosis assessment and treatment guidance. Commercial molecular signature test, ONCOTYPEDX
®
is routinely used for luminal breast cancers to predict the probabilities of response to chemotherapy and disease recurrence. We attempted to predict RS using digital pathology and Weakly Supervised (WS) attention-based models like CLAM (Clustering-constrained Attention Multiple Instance Learning) [1] and TransMIL (Transformer based Correlated Multiple Instance Learning) [2] on our in-house dataset. In tissue samples, the malignant component is haphazardly admixed with the nonmalignant component in variable proportions. This represents a challenge for the WS attention-based models to identify high-valued diagnostic/prognostic areas within whole slide images (WSIs). To address this, we propose an interactive approach with a human in the middle (supervised) by creating a user-friendly Graphical User Interface (GUI) that allows a pathologist to provide feedback to heatmaps generated by any WS attention-based model. We incorporate the feedback from the GUI as expected scores and penalize its difference with the attention scores in the successive training process (current scores). We observe an improvement in RS prediction after the pathologist’s feedback - a 5% rise in AUC and 4% in accuracy for CLAM and a 4.5% increase in AUC and 3% in accuracy for TransMIL. We analyze the generated heatmaps and notice an improvement in cosine similarity between the expected scores and attention scores before and after the feedback - 5% and 10% increase for CLAM and TransMIL, respectively. The implementation of the proposed approach and the dataset is available for download
1
. Our adaptive, interactive feedback system harmonizes attention scores with expert intuition and instills higher confidence in the system’s predictions. This study establishes a potent synergy between AI and pathologists.
更多查看译文
关键词
WS Attention models,heatmap feedback,interactive GUI,clinician’s correction,clinical diagnosis
AI 理解论文
溯源树
样例
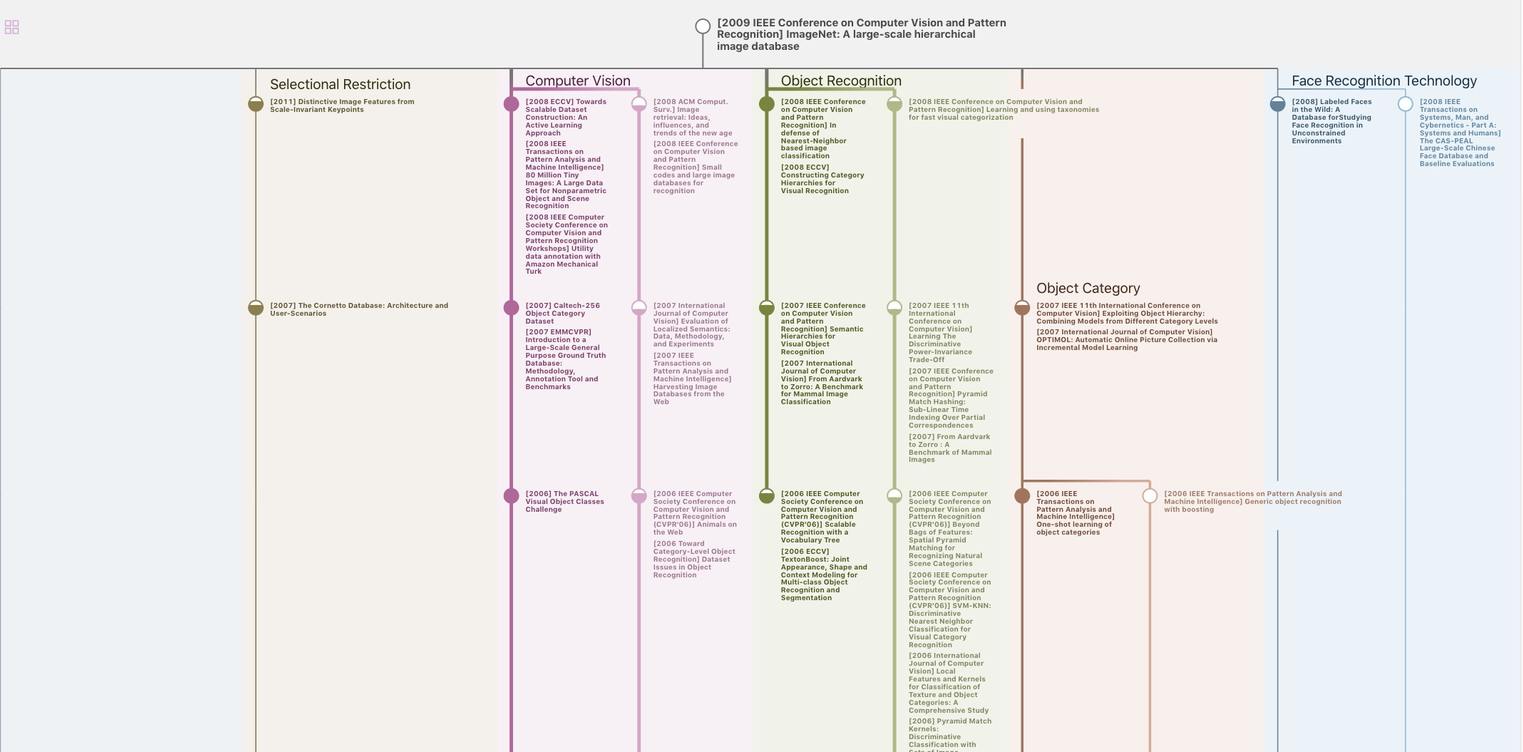
生成溯源树,研究论文发展脉络
Chat Paper
正在生成论文摘要