Contribution of CEEMDAN Decomposition in Enhancing the Forecast of Short-Term Global Solar Irradiation
2023 14th International Renewable Energy Congress (IREC)(2023)
摘要
Given the rapid growth and development of solar energy in recent years, accurate forecasting of solar output has become one of the most critical and formidable challenges in the modern power system. This paper introduces an approach for short-term global solar irradiance forecasting, combining Complete Ensemble Empirical Mode Decomposition with Adaptive Noise (CEEMDAN) with Artificial Neural Network (ANN) and Multiple Linear Regression (MLR) models. The CEEMDAN decomposition is employed to decompose original data series, extracting crucial features for forecasting. The model's performance is evaluated on two distinct sites in Algeria, characterized by Mediterranean and desert climates. Statistical tests reveal a significant enhancement in nRMSE values, with approximately 14.09% and 7.86% improvements for the ANN_CEEMDAN model and about 17.52% and 8.97% enhancements for the MLR_CEEMDAN model, specifically for the first hour of forecast.
更多查看译文
关键词
forecast,solar irradiance,short-term
AI 理解论文
溯源树
样例
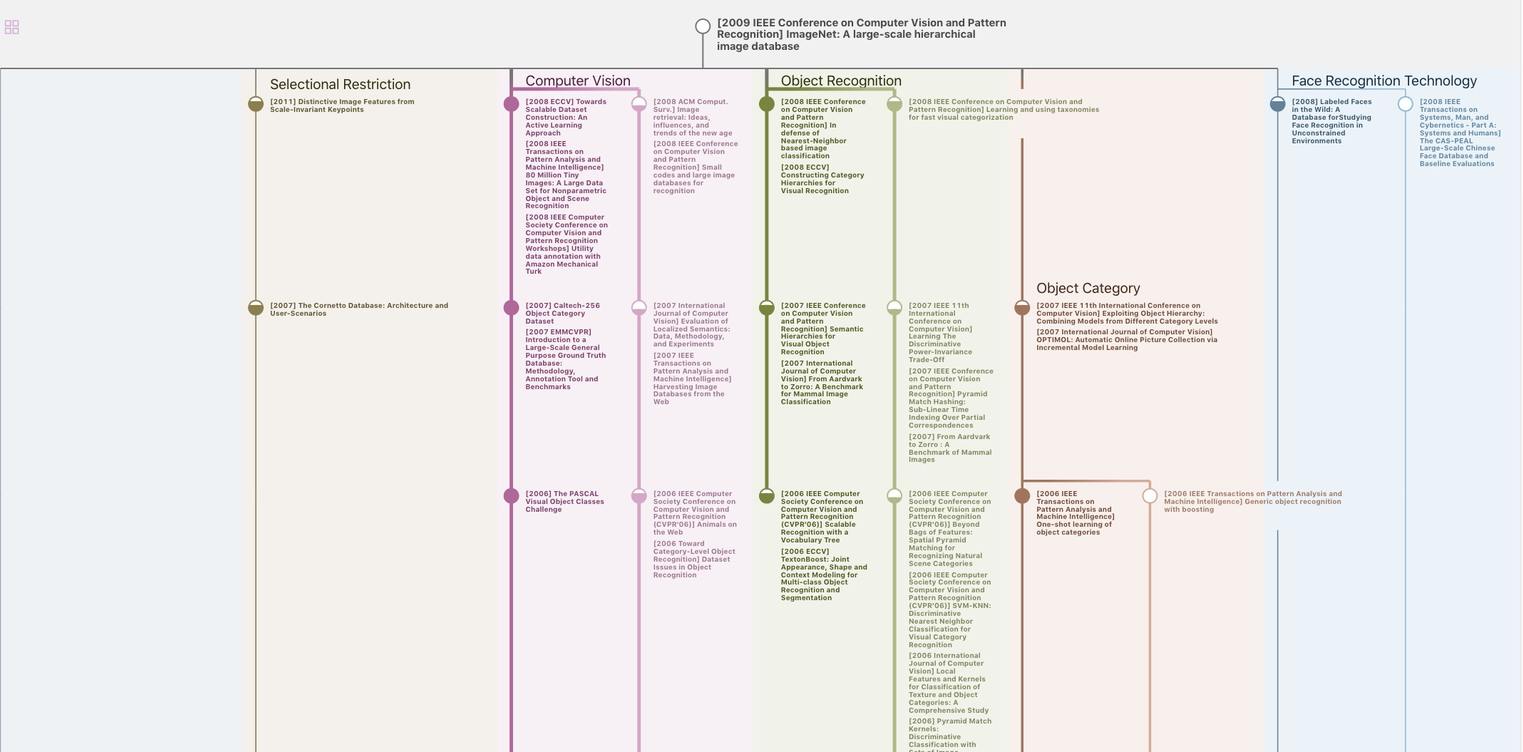
生成溯源树,研究论文发展脉络
Chat Paper
正在生成论文摘要