Unveiling the Black Box: Enhancing Trust and Accuracy in Social Media Rumour Detection Through Explainable Machine Learning
2023 20th International Computer Conference on Wavelet Active Media Technology and Information Processing (ICCWAMTIP)(2023)
摘要
This study evaluates machine learning classifiers; Logistic Regression, Random Forest, SVM, Passive Aggressive Classifiers, Decision Trees, KNN, Naïve Bayes, and Gradient Boosting Machines for detecting rumors on social media, utilizing datasets from FakeNewsNet and Politifact. While these traditional models are effective, their lack of transparency hinders user trust. To address this, we integrate Explainable AI (XAI) techniques; LIME and SHAP, to enhance interpretability and reliability. Our findings, based on rigorous evaluation metrics, reveal the tradeoffs between accuracy and transparency. The study demonstrates how XAI techniques uncover feature impacts on model decisions, contributing significantly to user trust in AI systems. This balanced approach, combining robust machine learning with XAI, advances our understanding of rumour detection in the dynamic social media landscape. It suggests a trajectory towards more transparent, adaptive, and user-trusted AI solutions, highlighting the need for continuous model updating and ethical considerations in future research.
更多查看译文
关键词
Rumour Detection Algorithms,Explainable AI (XAI) Techniques,Social Media Analytics,Machine Learning Evaluation,AI Transparency and Trust
AI 理解论文
溯源树
样例
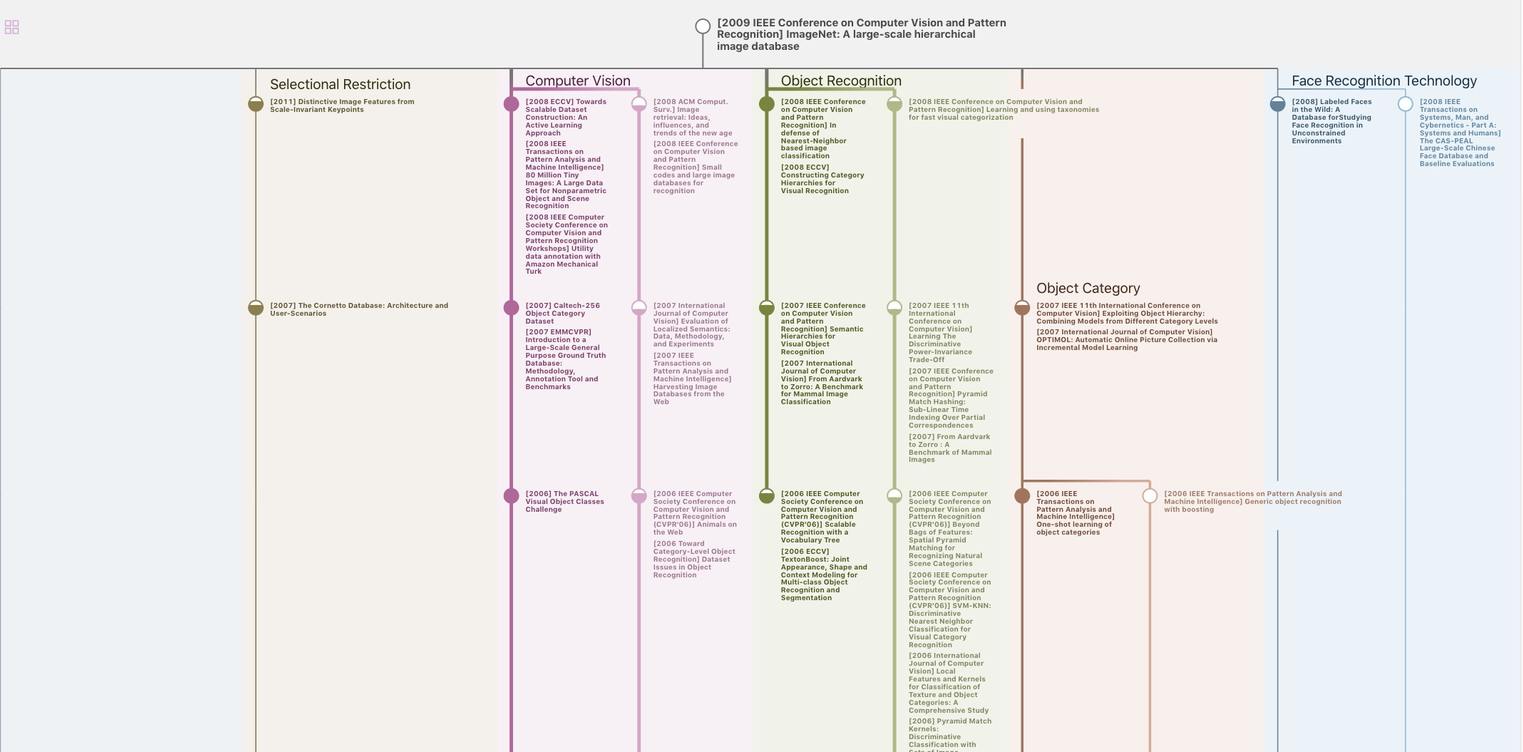
生成溯源树,研究论文发展脉络
Chat Paper
正在生成论文摘要