FARP-Net: Local-Global Feature Aggregation and Relation-Aware Proposals for 3D Object Detection
IEEE TRANSACTIONS ON MULTIMEDIA(2024)
摘要
In this work, we introduce FARP-Net, an adaptive local-global feature aggregation and relation-aware proposal network for high-quality 3D object detection from pure point clouds. Our key insight is that learning adaptive local-global feature aggregation from an irregular yet sparse point cloud and generating superb proposals are both pivotal for detection. Technically, we propose a novel local-global feature aggregation layer (LGFAL) that fully exploits the complementary correlation between local features and global features, and fuses their strengths adaptively via an attention-based fusion module. Furthermore, we incorporate a lightweight feature affine module (LFAM) into LGFAL to map the local features into a normal distribution, thus acquiring fine-grained features of each local region in a weight-sharing manner. During object proposal generation, we propose a weighted relation-aware proposal module (WRPM) that uses an objectness-aware formalism to weigh the relation importance among object candidates for a clear and principal context, thereby facilitating the generation of high-quality proposals. The WRPM challenges the traditional practice of extracting contextual information among all object candidates, which is inefficient as object candidates are always noisy and redundant. Experimentally, FARP-Net delivers superior performance on two widely used benchmarks with fewer parameters, 64.0% mAP@0.25 on the SUN RGB-D dataset and 70.9% mAP@0.25 on the ScanNet V2 dataset. We further validate that the proposed LGFAL and WRPM can be integrated into both indoor and outdoor detectors to boost performance.
更多查看译文
关键词
3D object detection,deep learning,point cloud
AI 理解论文
溯源树
样例
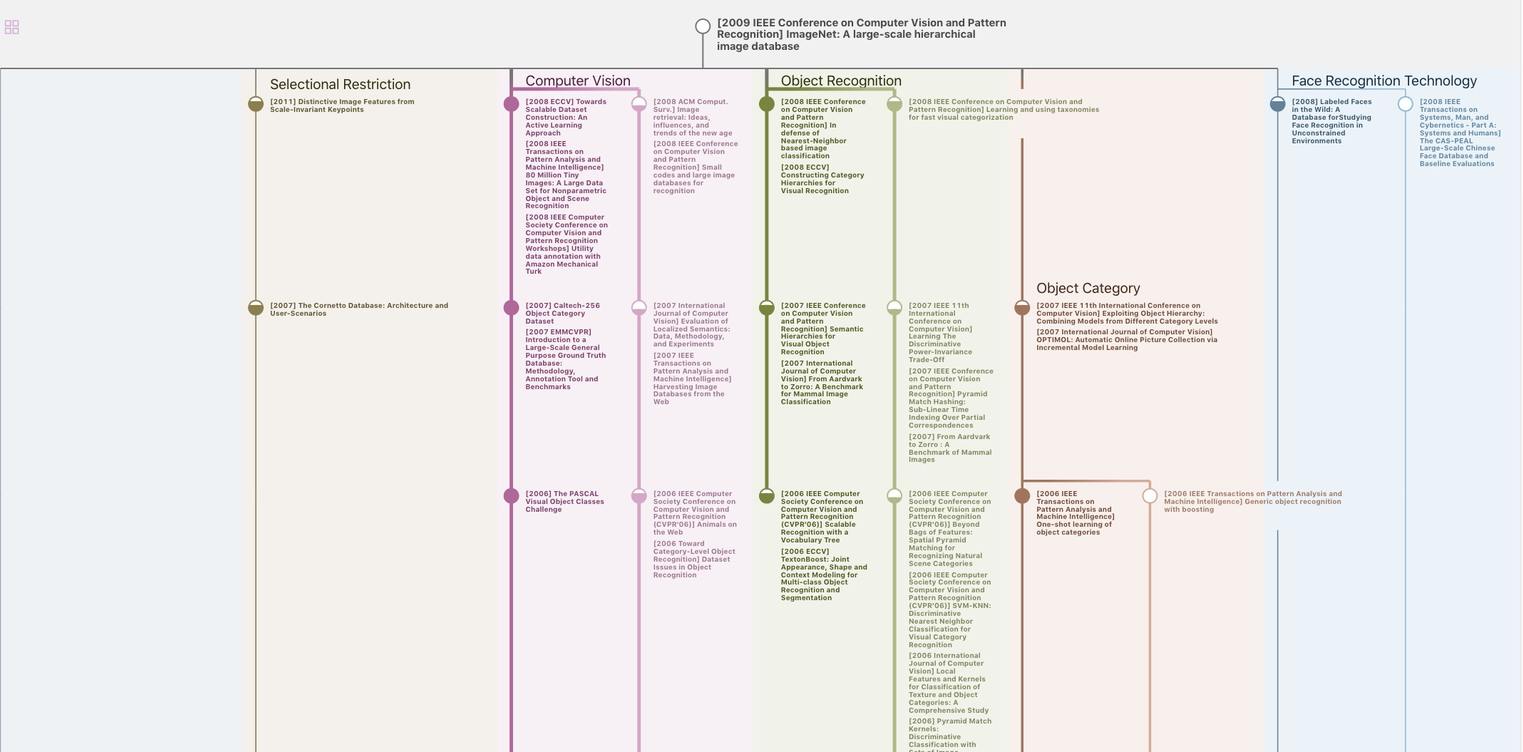
生成溯源树,研究论文发展脉络
Chat Paper
正在生成论文摘要