Ensembling Multi-View Discriminative Semantic Feature for Few-Shot Classification
ENGINEERING APPLICATIONS OF ARTIFICIAL INTELLIGENCE(2024)
摘要
Few-Shot Classification (FSC) is an innovative application in machine learning. FSC consists of two main components: (1) Pre-training, where a feature extraction model (FEM) is trained using base data, and (2) Meta-testing, where the FEM is utilized to extract features from novel data (with categories different from the base data) for classification. Implementing FSC presents several challenges. For example, due to the cross-domain limitation (base data -> novel data), the FEM may generate inappropriate features for new classes, leading to a Sample-Feature-Mismatch problem, and the corresponding feature is dubbed as Original-Shift-Feature (OSF). This paper proposes a generative method to construct Multi-View Discriminative Semantic Feature (MVDSF) to address the fundamental problem from the perspective of improving the discriminability of OSF. Typically, a linear projection is designed to transform OSF into a semantic space, generating Discriminative-Semantic-Features (DSF). By incorporating a reconstructive representation that is not solely reliant on the FEM, the influence of the Sample-Feature-Mismatch problem is reduced. Furthermore, considering that descriptions based on a single DSF tend to be one-sided, an attention mechanism is devised to fuse multi-view DSF, thereby improving the robustness of the proposed method. The effectiveness of MVDSF is evaluated on five benchmark few-shot learning datasets, where it achieves outstanding performance. This evaluation demonstrates the efficiency and performance of the proposed approach.
更多查看译文
关键词
Few-Shot Classification (FSC),Feature-Mismatch-Sample (FMS) problem,Multi-View Discriminative Semantic Feature (MVDSF),Original-Shift-Feature (OSF),Discriminative-Semantic-Feature (DSF)
AI 理解论文
溯源树
样例
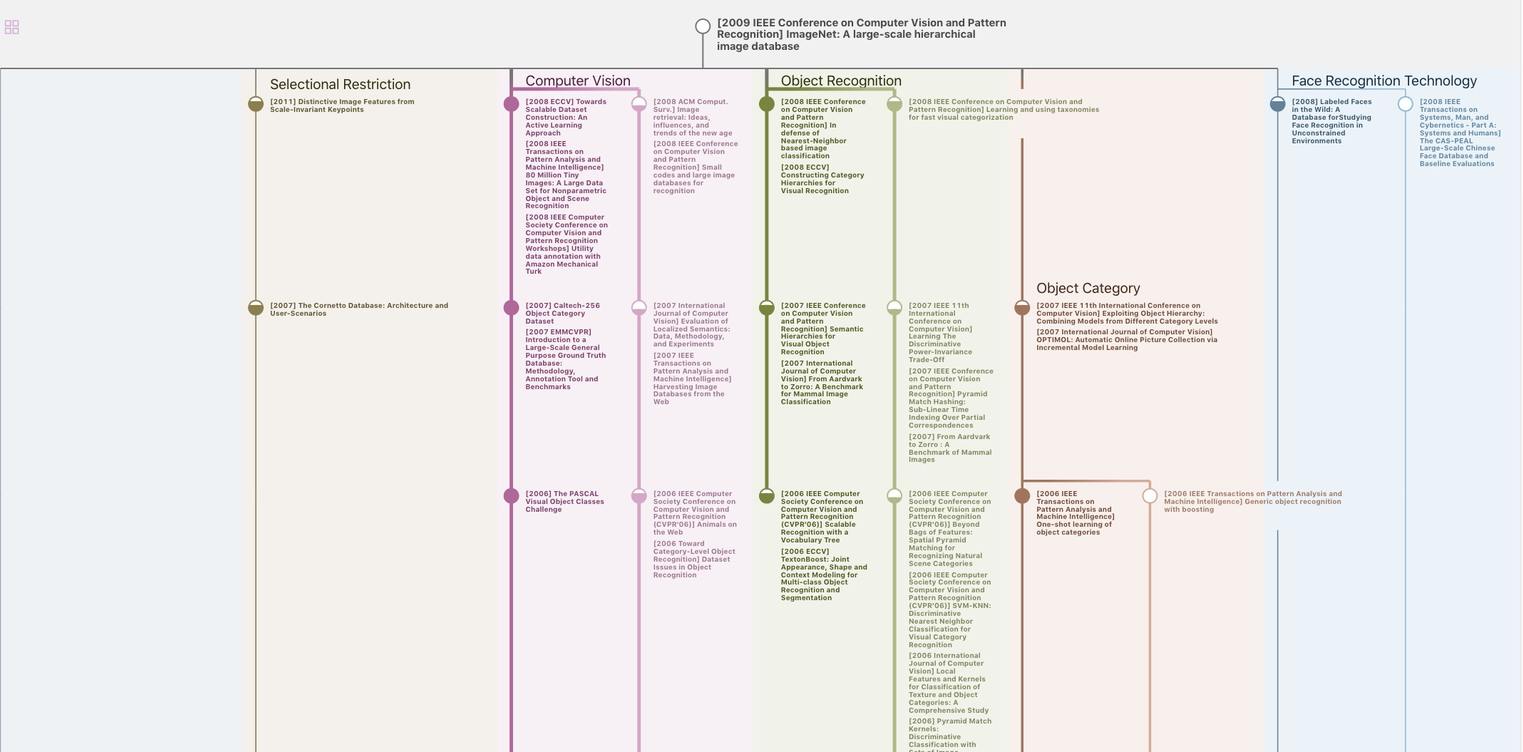
生成溯源树,研究论文发展脉络
Chat Paper
正在生成论文摘要