Meta-learning for efficient unsupervised domain adaptation
NEUROCOMPUTING(2024)
摘要
The standard machine learning assumption that training and test data are drawn from the same probability distribution does not hold in many real -world applications due to the inability to reproduce testing conditions at training time. Existing unsupervised domain adaption (UDA) methods address this problem by learning a domain -invariant feature space that performs well on available source domain(s) (labeled training data) and the specific target domain (unlabeled test data). In contrast, instead of simply adapting to domains, this paper aims for an approach that learns to adapt effectively to new unlabeled domains. To do so, we leverage meta -learning to optimize a neural network such that an unlabeled adaptation of its parameters to any domain would yield a good generalization on this latter. The experimental evaluation shows that the proposed approach outperforms standard approaches even when a small amount of unlabeled test data is used for adaptation, demonstrating the benefit of meta -learning prior knowledge from various domains to solve UDA problems.
更多查看译文
关键词
Domain adaptation,Meta-learning,Unsupervised learning,Distribution shift
AI 理解论文
溯源树
样例
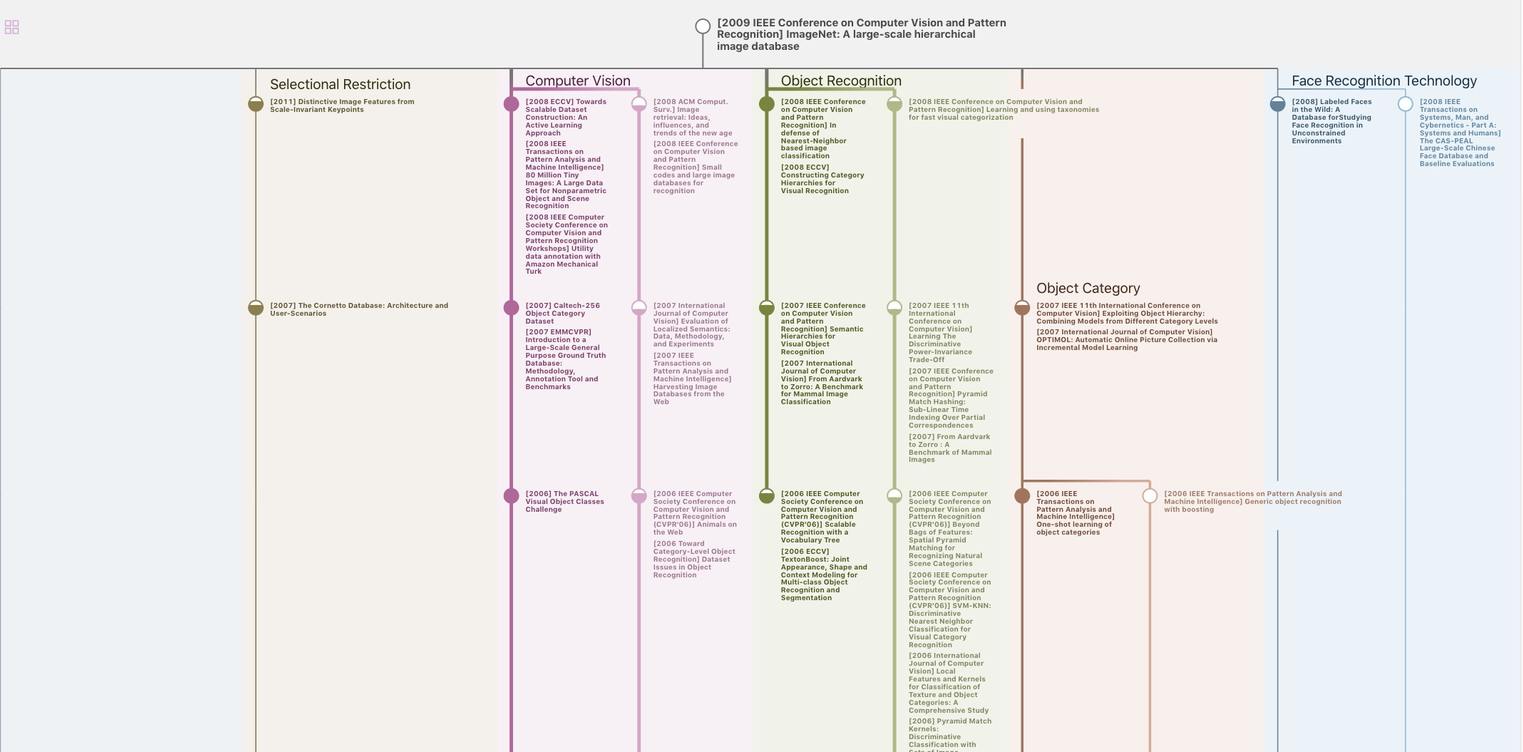
生成溯源树,研究论文发展脉络
Chat Paper
正在生成论文摘要