True benefits of multiple nanoparticle sizes in radiative cooling paints identified with machine learning
INTERNATIONAL JOURNAL OF HEAT AND MASS TRANSFER(2024)
摘要
Previous works find that multiple nanoparticle sizes in radiative cooling paints increase solar reflectance compared to a single particle size. In this study, we assess this finding by combining Mie theory, Monte Carlo simulations, and machine learning methods to identify the optimum particle size combinations in BaSO4 and TiO2-acrylic radiative cooling paints. We have found that the optimal multiple particle sizes indeed outperform the optimal single size in TiO2 paint, but surprisingly underperform compared to the optimal single size in BaSO4 paint. This is due to the near constant refractive index of BaSO4 across the solar spectrum. Also, different particle size distributions yield similarly high solar reflectance as long as the average particle size is in the neighborhood of 300-600 nm. Considering that it is unfeasible to precisely manufacture a single particle size, we conclude that the true benefits of multiple particle sizes is that they enable cost effective manufacturing while preserving robust high performance.
更多查看译文
关键词
Radiative cooling,Machine learning,Optimization
AI 理解论文
溯源树
样例
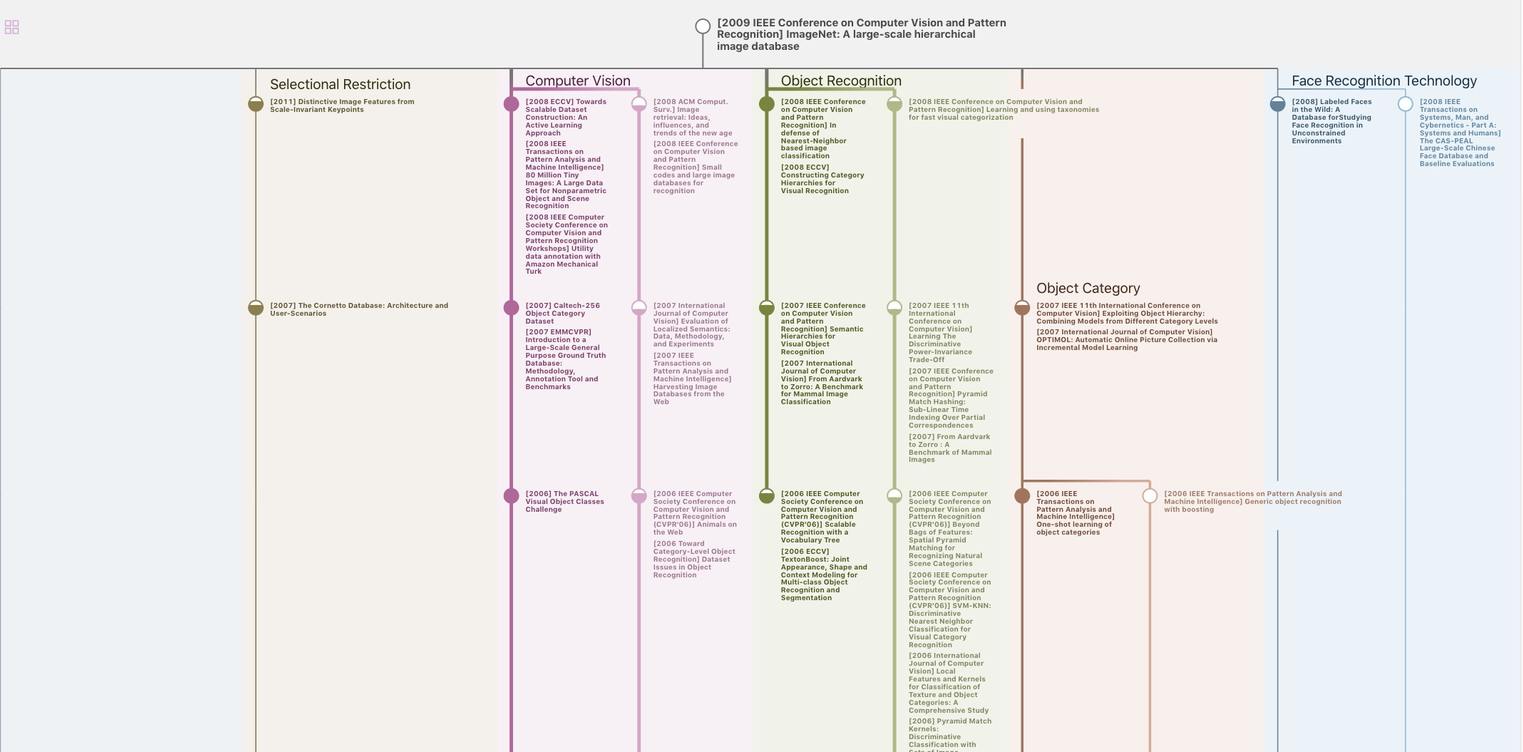
生成溯源树,研究论文发展脉络
Chat Paper
正在生成论文摘要