Predicting CKD progression using time-series clustering and light gradient boosting machines
Scientific Reports(2024)
摘要
Predicting the transition of kidney function in chronic kidney disease is difficult as specific symptoms are lacking and often overlooked, and progress occurs due to complicating factors. In this study, we applied time-series cluster analysis and a light gradient boosting machine to predict the trajectories of kidney function in non-dialysis dependent chronic kidney disease patients with baseline estimated glomerular filtration rate (GFR) ≥ 45 mL/min/1.73 m 2 . Based on 5-year changes in estimated GFR, participants were stratified into groups with similar trajectories by cluster analysis. Next, we applied the light gradient boosting machine algorithm and Shapley addictive explanation to develop a prediction model for clusters and identify important parameters for prediction. Data from 780 participants were available for analysis. Participants were classified into five classes (Class 1: n = 78, mean [± standard deviation] estimated GFR 100 ± 19.3 mL/min/1.73 m 2 ; Class 2: n = 176, 76.0 ± 9.3 mL/min/1.73 m 2 ; Class 3: n = 191, 59.8 ± 5.9 mL/min/1.73 m 2 ; Class 4: n = 261, 52.7 ± 4.6 mL/min/1.73 m 2 ; and Class 5: n = 74, 53.5 ± 12.0 mL/min/1.73 m 2 ). Declines in estimated GFR were 8.9% in Class 1, 12.2% in Class 2, 4.9% in Class 3, 12.0% in Class 4, and 45.1% in Class 5 during the 5-year period. The accuracy of prediction was 0.675, and the top three most important Shapley addictive explanation values were 1.61 for baseline estimated GFR, 0.12 for hemoglobin, and 0.11 for body mass index. The estimated GFR transition of patients with preserved chronic kidney disease mostly depended on baseline estimated GFR, and the borderline for estimated GFR trajectory was nearly 50 mL/min/1.73 m 2 .
更多查看译文
AI 理解论文
溯源树
样例
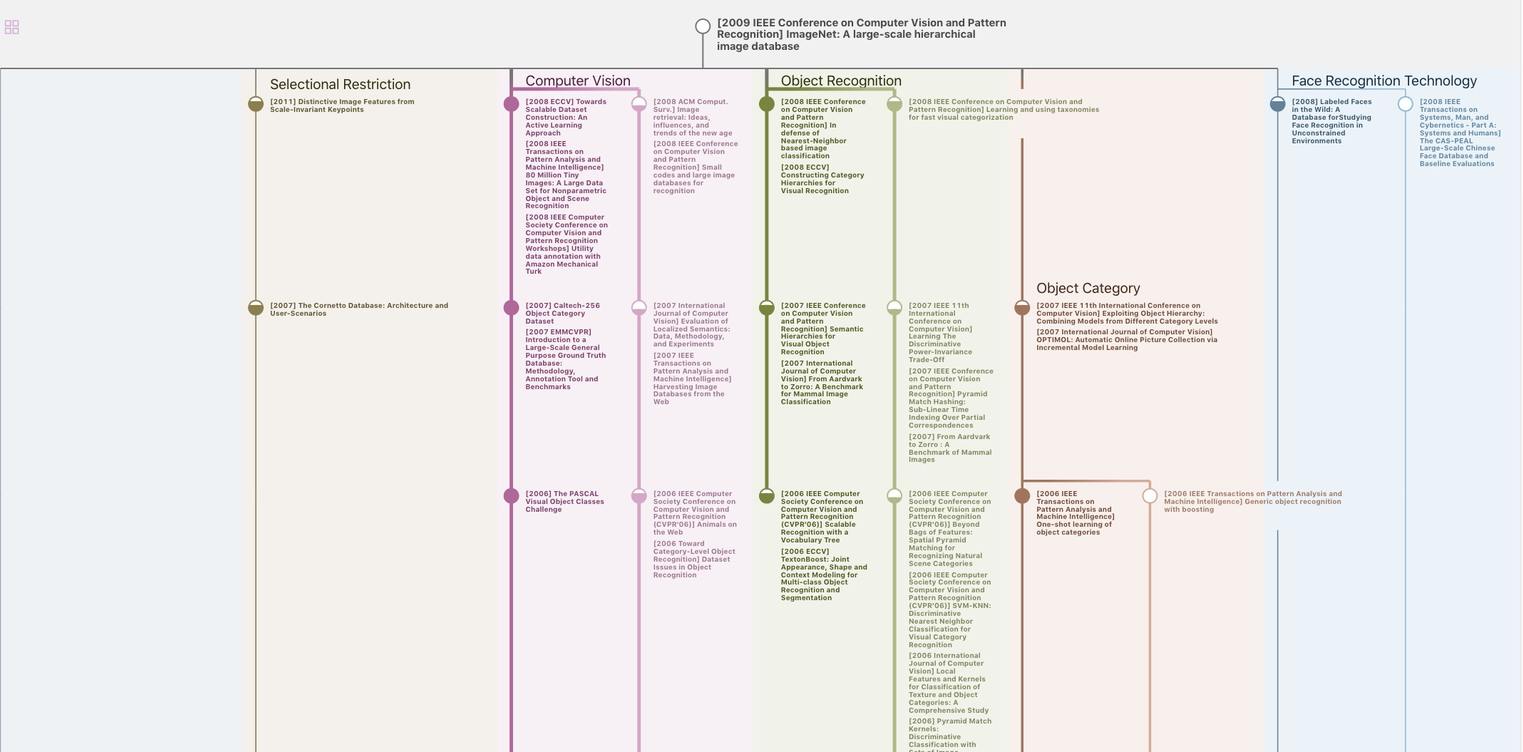
生成溯源树,研究论文发展脉络
Chat Paper
正在生成论文摘要