Robust augmented space recursive least-constrained-squares algorithms
SIGNAL PROCESSING(2024)
摘要
This paper proposes a novel augmented space robust adaptive filter by reusing the errors for online applications. First, a batched augmented space constrained model (ASCM) is constructed to combat nonGaussian noise. In ASCM, the errors are reused by k nearest neighbors (k -NN) estimation. Then, an augmented space recursive least -constrained -squares algorithm integrating with distance -based k -NN method (ARLCS-dk) is developed within the framework of ASCM for adaptive filtering. Finally, to curb the size of ever-growing error network, a sliding window ARLCS-dk (SW-ARLCS-dk) is proposed to reduce the computational burden. Theoretical analyses of excess mean square error (EMSE) and testing mean square error (TMSE) are carried out for performance evaluation. Examples on time -series prediction of simulated and real -world data are used to illustrate the advantages of proposed algorithms on robustness and prediction accuracy.
更多查看译文
关键词
Adaptive filters,Augmented space constrained model,Robustness,k nearest neighbors method,Sliding window
AI 理解论文
溯源树
样例
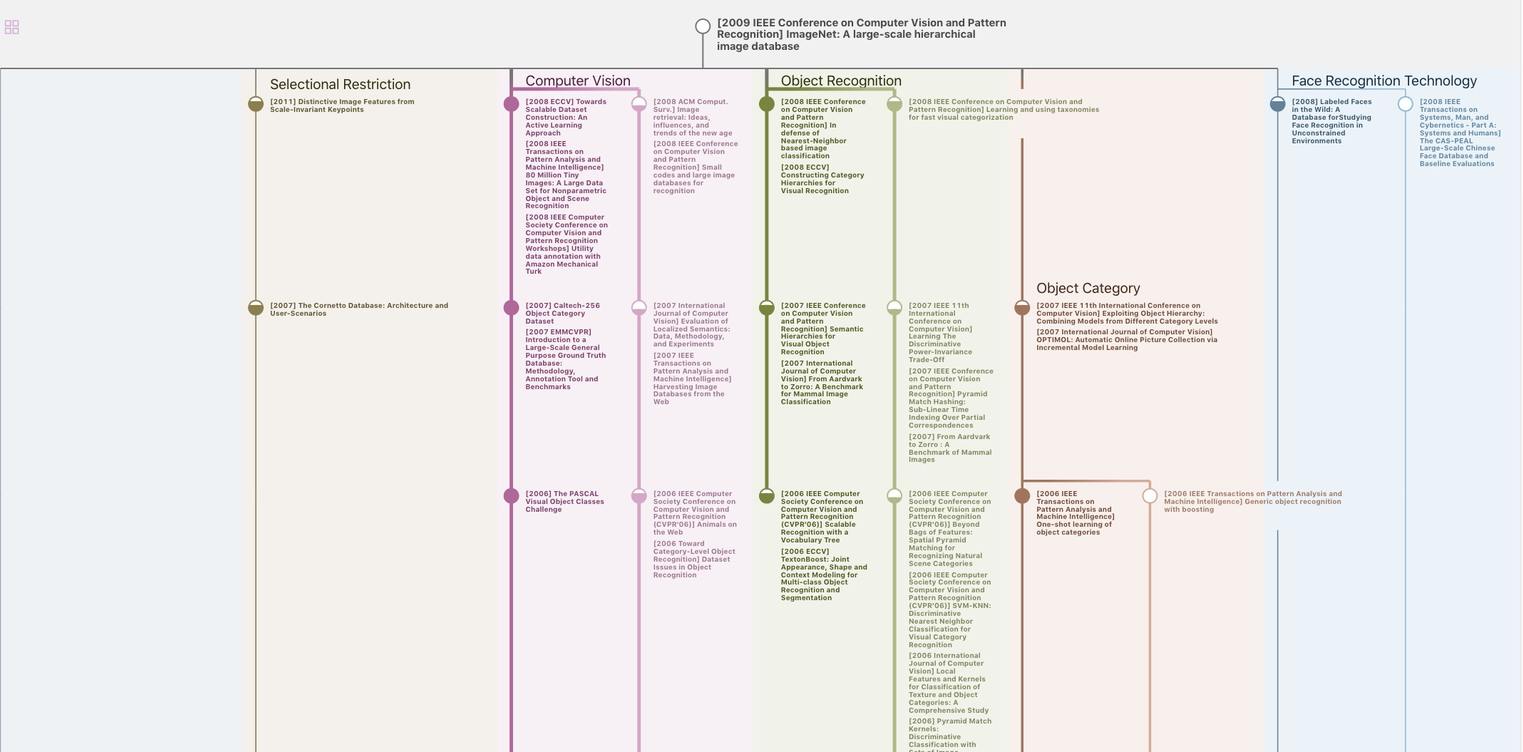
生成溯源树,研究论文发展脉络
Chat Paper
正在生成论文摘要