Reducing vulnerable internal feature correlations to enhance efficient topological structure parsing
EXPERT SYSTEMS WITH APPLICATIONS(2024)
摘要
Most cropping-and-segmenting pattern parsers typically establish a single metric/scheme to reason diverse inner correlations, resulting in over-general and redundant representations. To make pattern parsing procedure more streamlined and concise, a part-relation scissor network (PRSN) with multi-constrained attention shifters (MCASs) and multi-head attention expectation-maximum routing agreement (MhAEMRA) is proposed on the basic of matrix-based capsule network (CapsNet). MCASs detect and prune fragile part-to-whole correlations from the perspectives of inter-part diversity and intra-object cohesion. They stipulate that only those primary entities fulfilling the criteria of inter-part diversity and intra-object cohesiveness can update senior entities. MhAEMRA is defined to shield the redundant capsule voting signals. PRSN gradually parses objective semantic patterns by clustering highly associated secondary entities in a bottom-up "part backtracking" manner. Quantitative and ablation experiments surrounding face and human parsing tasks demonstrate the superiority of PRSN over the state-of-the-arts, especially for the definition of fine-grained semantic boundaries.
更多查看译文
关键词
Face parsing,Human parsing,Graph attention,Capsule network,Expectation -maximum routing agreement
AI 理解论文
溯源树
样例
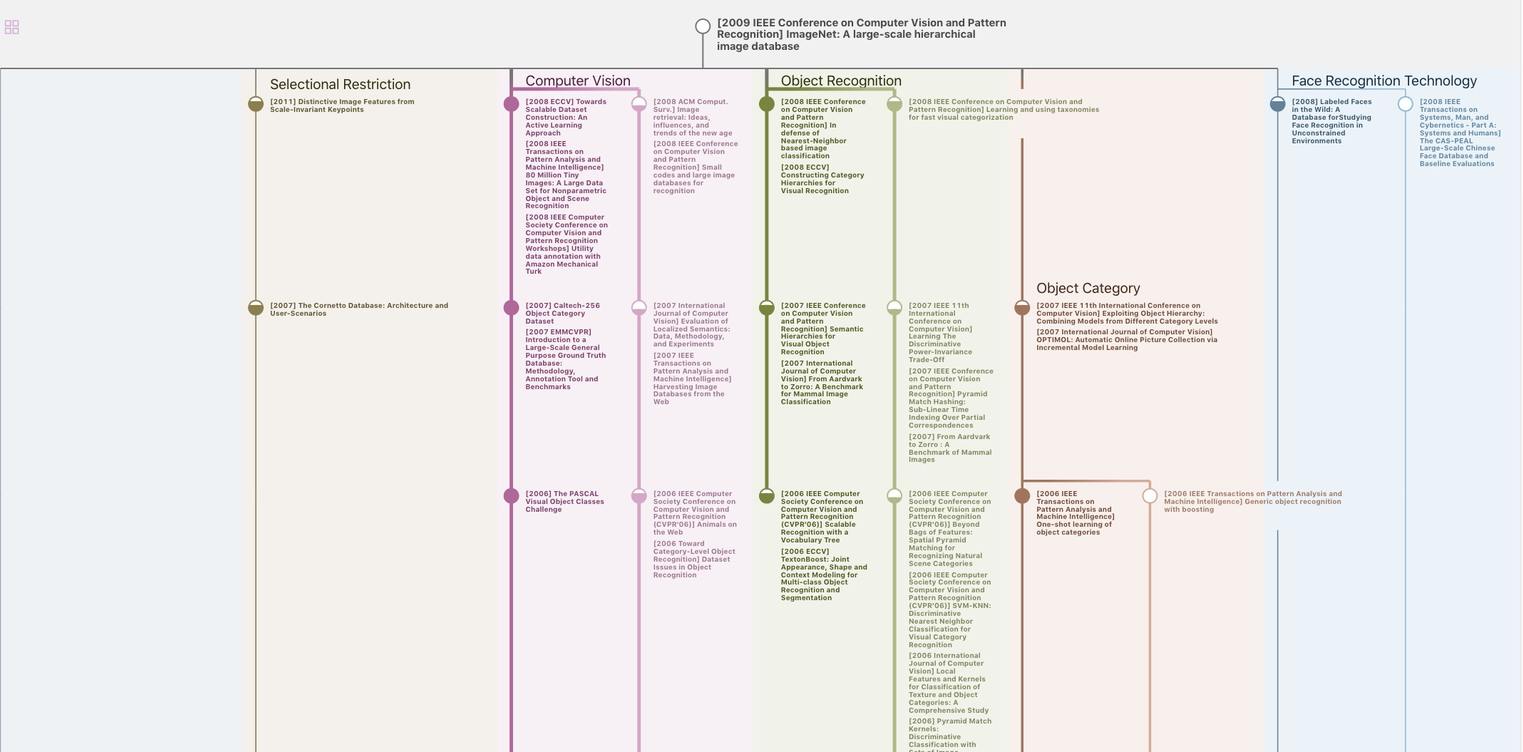
生成溯源树,研究论文发展脉络
Chat Paper
正在生成论文摘要