Tensor recovery using the tensor nuclear norm based on nonconvex and nonlinear transformations
SIGNAL PROCESSING(2024)
摘要
Recently, the tensor completion problem has witnessed significant advancements with the introduction of the tensor nuclear norm based on self -supervised nonlinear transformations (S2NTNN). However, the tensor nuclear norm serves as a convex substitute for the tubal rank of tensors, often resulting in suboptimal solutions to the original problem. In this work, we propose a novel method, called Nonconvex Nonlinear transformation -based Tensor Nuclear Norm (N2TNN), which builds upon nonlinear transformations and introduces a generalized nonconvex function to better exploit the prior information of underlying tensors. Additionally, we utilize regularization techniques, namely spatial total variation (TV) and spatial spectral total variation (SSTV), to enhance both the details and edge information. Extensive numerical experiments unequivocally validate the superior performance of our proposed method when compared to other state-of-the-art techniques in terms of both numerical accuracy and visual quality.
更多查看译文
关键词
Nonlinear transformation,Nonconvex function,Tensor nuclear norm,Self-supervised learning
AI 理解论文
溯源树
样例
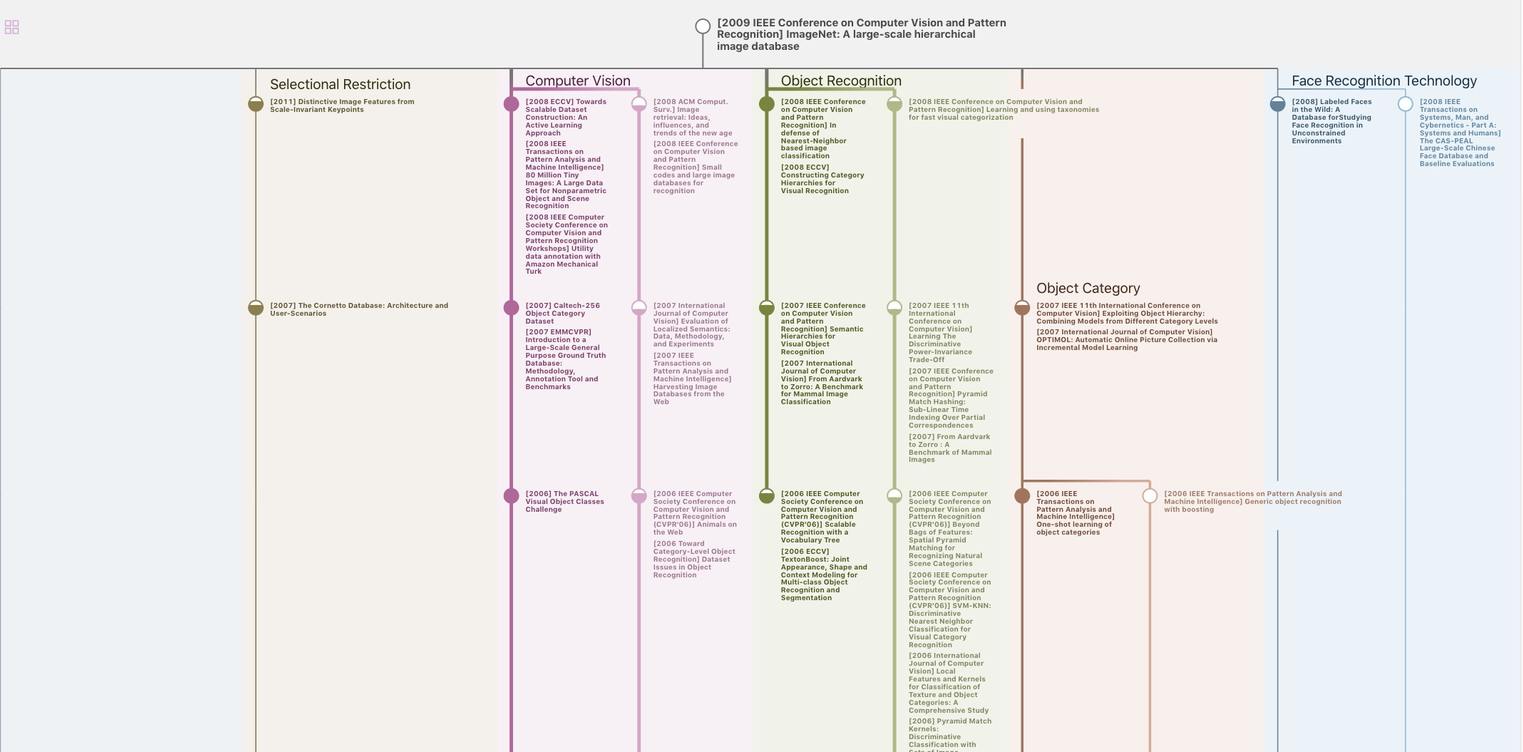
生成溯源树,研究论文发展脉络
Chat Paper
正在生成论文摘要