Application of Machine Learning and Deep EfficientNets in Distinguishing Neonatal Adrenal Hematomas From Neuroblastoma in Enhanced Computed Tomography Images
WORLD JOURNAL OF ONCOLOGY(2024)
摘要
Background: The aim of the study was to employ a combination of radiomic indicators based on computed tomography (CT) imaging and machine learning (ML), along with deep learning (DL), to differentiate between adrenal hematoma and adrenal neuroblastoma in neonates. Methods: A total of 76 neonates were included in this retrospective study (40 with neuroblastomas and 36 with adrenal hematomas) who underwent CT and divided into a training group (n=38) and a testing group (n=38). The regions of interest (ROIs) were segmented by two radiologists to extract radiomics features using Pyradiomics package. ML classifications were done using support vector machine (SVM), AdaBoost, Extra Trees, gradient boosting, multi-layer perceptron (MLP), and random forest (RF). EfficientNets was employed and classified, based on radiometrics. The area under curve (AUC) of the receiver operating characteristic (ROC) was calculated to assess the performance of each model. Results: Among all features, the least absolute shrinkage and selection operator (LASSO) logistic regression selected nine features. These radiomics features were used to construct radiomics model. In the training cohort, the AUCs of SVM, MLP and Extra Trees models were 0.967, 0.969 and 1.000, respectively. The corresponding AUCs of the test cohort were 0.985, 0.971 and 0.958, respectively. In the classification task, the AUC of the DL framework was 0.987. Conclusion: ML decision classifiers and DL framework constructed from CT-based radiomics features offered a non-invasive method to differentiate neonatal adrenal hematoma from neuroblastoma and performed better than the clinical experts.
更多查看译文
关键词
Neuroblastoma,Adrenal hematomas,Machine learning,Radiomics,Neonate
AI 理解论文
溯源树
样例
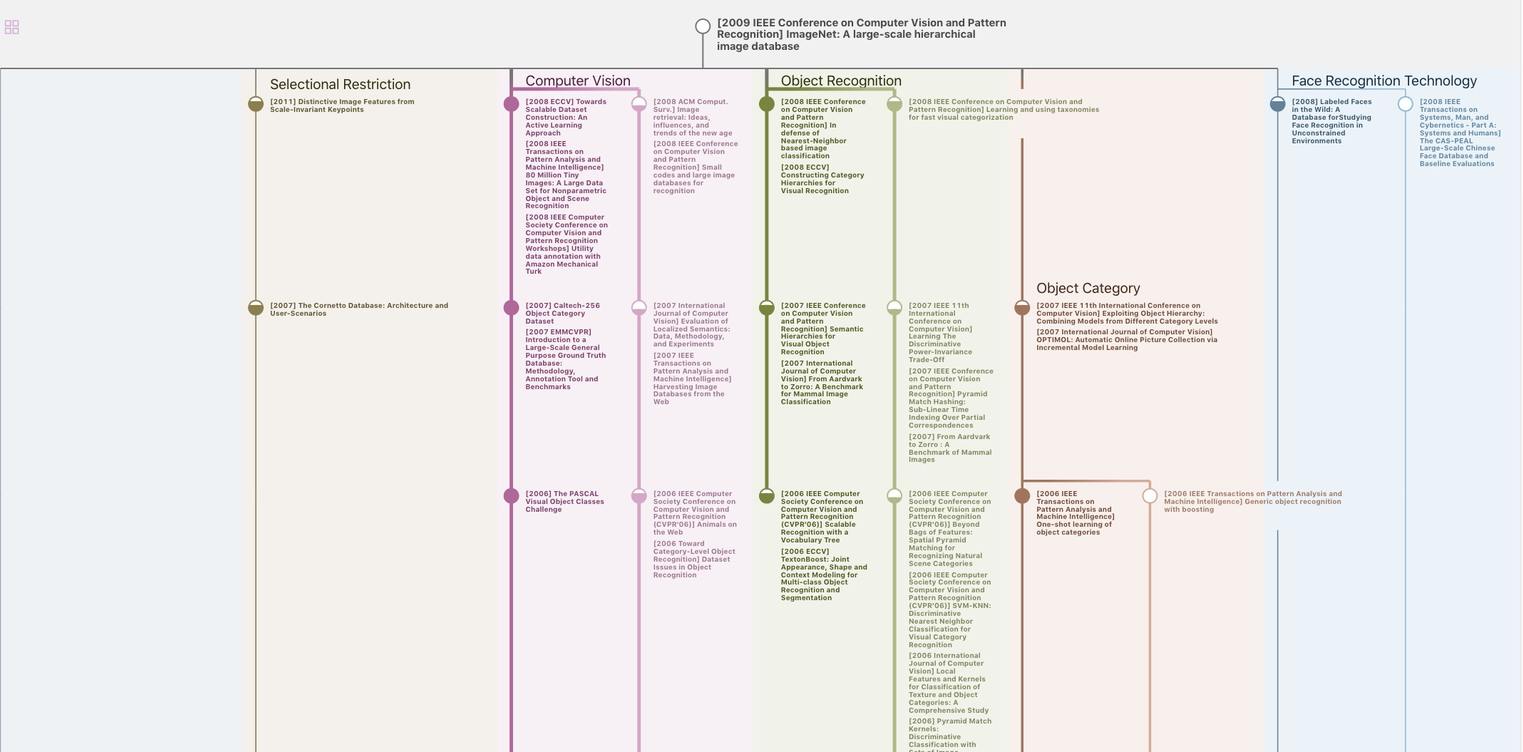
生成溯源树,研究论文发展脉络
Chat Paper
正在生成论文摘要