Machine learning-based optimization of catalytic hydrodeoxygenation of biomass pyrolysis oil
JOURNAL OF CLEANER PRODUCTION(2024)
摘要
Bio-oil derived from biomass pyrolysis contains various oxygenated compounds, compromising its quality. Catalytic hydrodeoxygenation (HDO) holds promise for upgrading low-quality bio-oil into valuable fuels and chemicals. However, developing stable and efficient catalysts and selecting optimum operating conditions is challenging. This study employs machine learning (ML) technology to navigate complex data relationships and optimize process parameters during bio-oil catalytic HDO. The goal is to establish an ML-based framework for modeling and fine-tuning catalytic HDO of guaiacol as a bio-oil model compound. Operating conditions and catalyst textural attributes serve as independent variables. A comprehensive database, compiled via a thorough literature review, covers various catalyst characteristics and reaction conditions. Four ML models are developed, with gradient boosting regression demonstrating superior predictive performance (R = 0.73-0.95) for guaiacol conversion and product distribution. Feature importance analysis highlights the significant influence of catalyst surface area and temperature. Through multi-objective optimization, an optimum guaiacol conversion of 92.26% is achieved. These conditions are 365(degrees)C, hydrogen pressure of 2.72 MPa, catalyst crystallinity index of 37%, and surface area of 756.9 m(2)/g. The results of this study provide a robust framework for effectively enhancing catalytic HDO, thus enabling the production of high-value chemical compounds from low-quality biomass pyrolysis oil.
更多查看译文
关键词
Biomass pyrolysis,Catalytic hydrodeoxygenation,Bio-oil upgrading,Machine learning modeling,Guaiacol conversion,Feature importance analysis
AI 理解论文
溯源树
样例
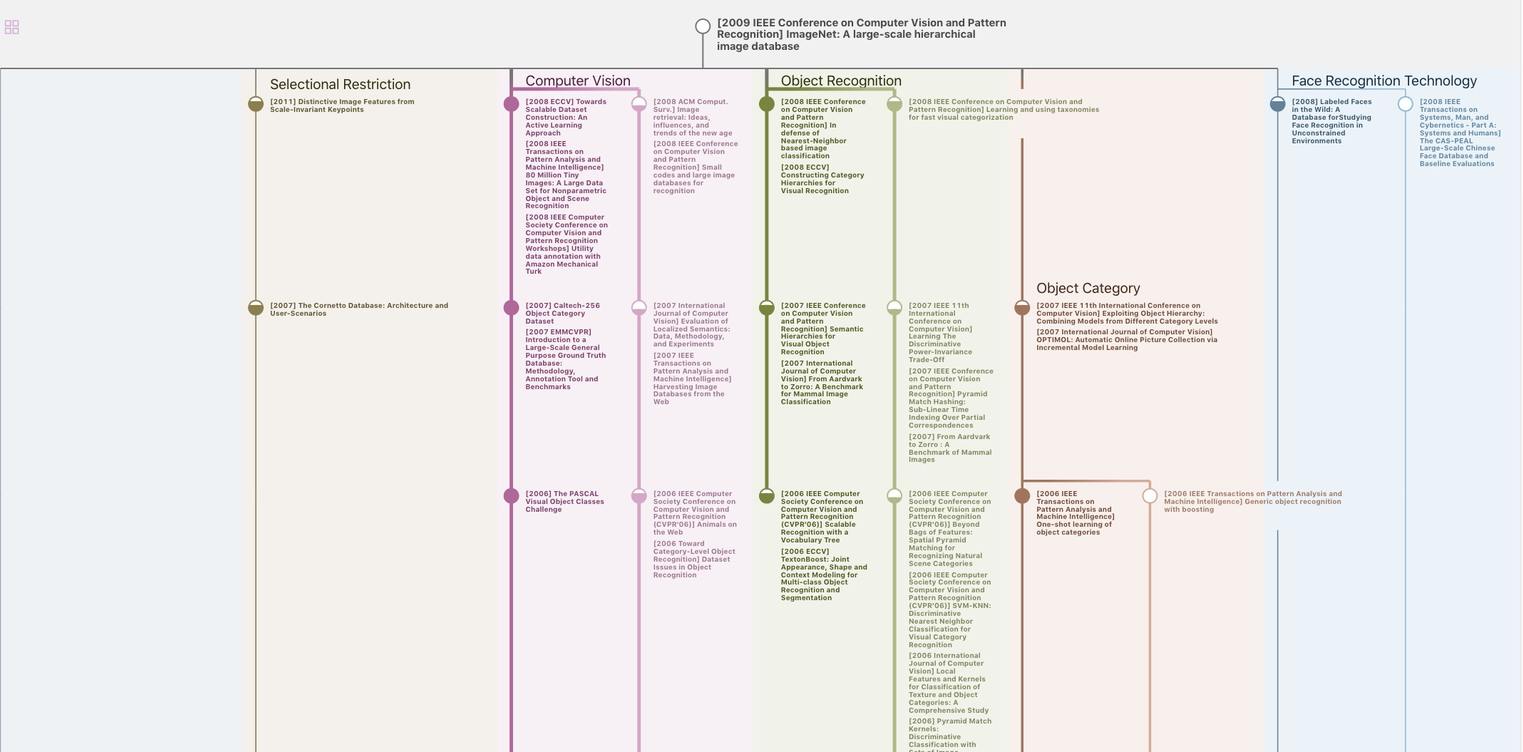
生成溯源树,研究论文发展脉络
Chat Paper
正在生成论文摘要