CAMSAT: Augmentation Mix and Self-Augmented Training Clustering for Self-Supervised Speaker Recognition.
2023 IEEE Automatic Speech Recognition and Understanding Workshop (ASRU)(2023)
摘要
Clustering (CL)-based pseudo-labels (PLs) are widely used to optimize speaker embedding (SE) networks and train self-supervised (SS) speaker verification (SV) systems. However, PL-based SS training depends on high-quality PLs. In this paper, we propose a general-purpose CL algorithm called CAMSAT that outperforms all other baselines used to cluster SEs. Moreover, using the generated PLs to train our SE system allows us to further improve SV performance. CAMSAT is based on two principles: (1) mixing predictions of augmented samples to provide a complementary supervisory signal for CL and enforce symmetry within augmentations (2) Self-Augmented Training to enforce representation invariance and maximize the information-theoretic dependency between samples and their predicted PLs. We provide a thorough comparative analysis of the performance of our CL method vs. all baselines using a variety of CL metrics and perform an ablation study to analyze the contribution of each component.
更多查看译文
关键词
Speaker Verification,Speaker Embeddings,Clustering Algorithm,Pseudo-Labels
AI 理解论文
溯源树
样例
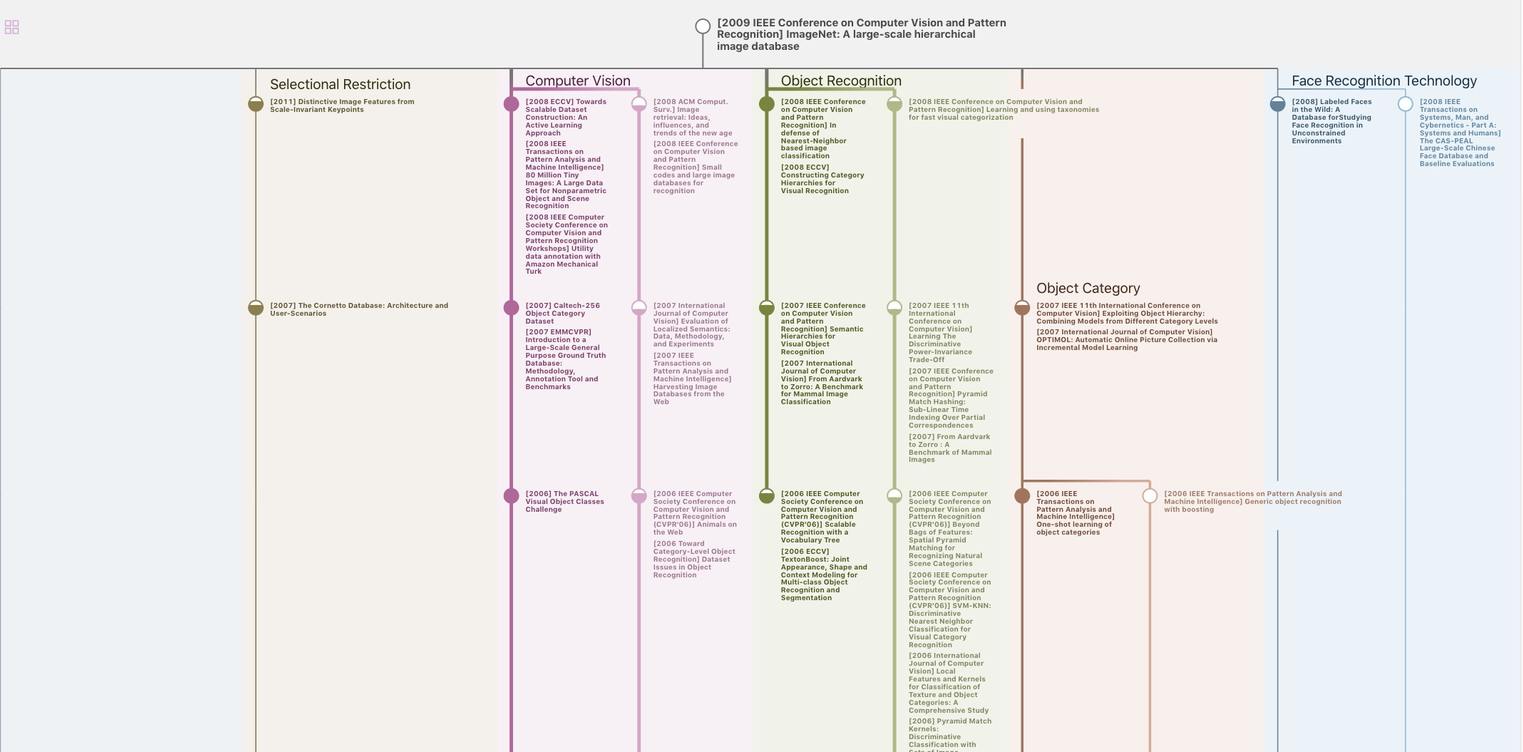
生成溯源树,研究论文发展脉络
Chat Paper
正在生成论文摘要