Transfer learning neural network for reconstructing temperature field in film cooling with scarce local measurements
ENERGY(2024)
摘要
Film cooling is widely adopted for thermal protection of gas turbine blades. Obtaining the surface temperature field is essential for film cooling design. However, it is generally challenging because temperature measurement points are scarce in practice. Although machine learning models can be trained to reconstruct the temperature field, their accuracy is limited when the measurement points are insufficient and the explicit physical constraints are lacking. To address the problem, a transfer learning neural network (TLNN) is proposed in present study. The main idea is to learn the general characteristics of temperature field from abundant data in other working conditions as implicit constraints, and to make fine-tuning of the model for a specific working condition with scarce measurement points. Numerical simulations were conducted under various working conditions to generate dataset for model training and testing. With the blowing ratios of 1 and 0.5 used as the source and target working conditions, the mean relative L2 -norm errors are 52 %, 41 %, and 32 % lower for 20, 10, and 5 measurement points, respectively, compared to the model without transfer learning. The results indicate that the proposed TLNN model can accurately reconstruct the temperature field in film cooling with scarce measurement points.
更多查看译文
关键词
Temperature field reconstruction,Film cooling,Neural network,Transfer learning,Scarce data
AI 理解论文
溯源树
样例
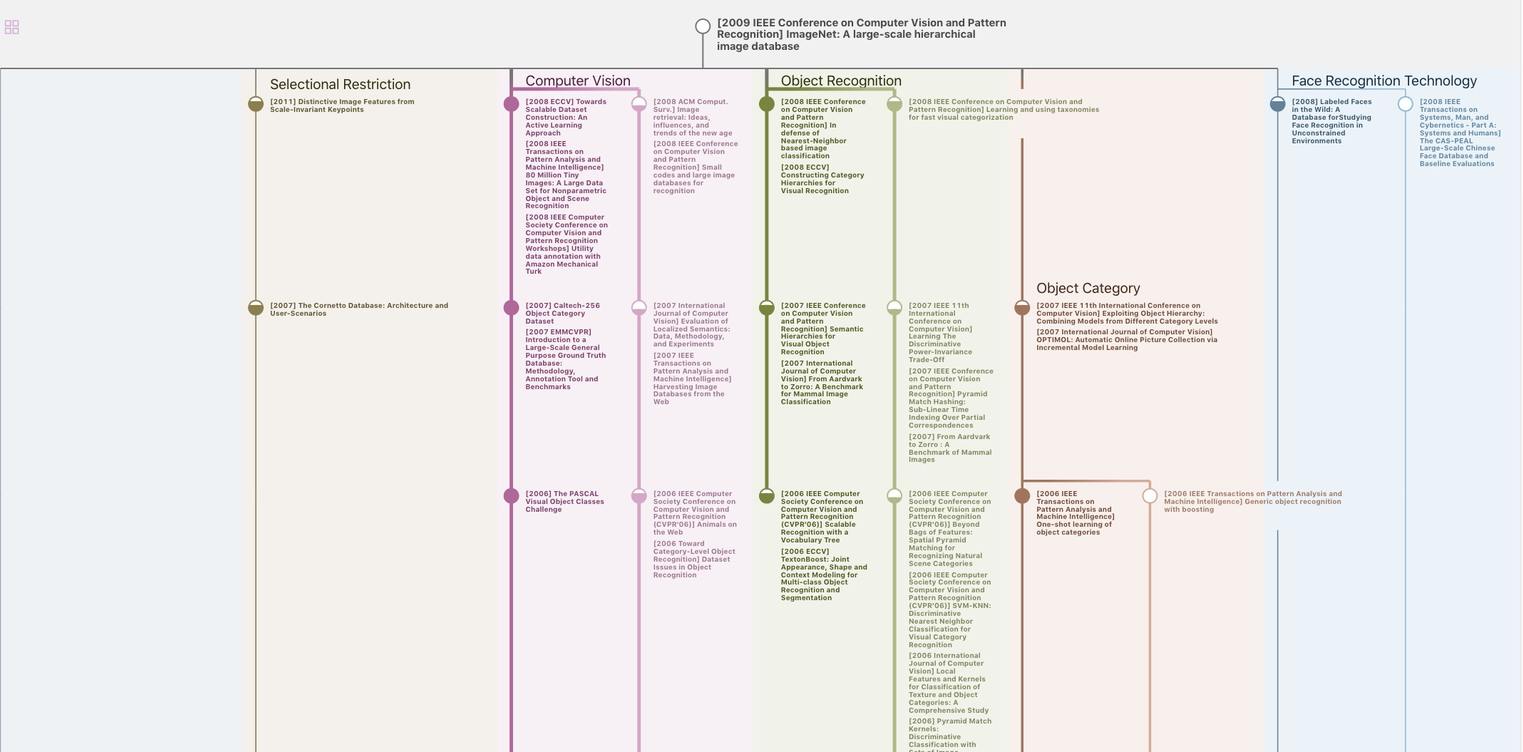
生成溯源树,研究论文发展脉络
Chat Paper
正在生成论文摘要