A model-free method based on RDPG for fiber diameter steady control
OPTICAL FIBER TECHNOLOGY(2024)
摘要
Optical fibers have made outstanding contributions to optical instruments such as micro-channel plates (MCPs) and micro-pore optics (MPOs). As the stricter performance requirements for the MCP and MPO, traditional diameter control methods are incapable. Additionally, the partial observation issue of fiber drawing process further limits the outcome of the existing control approaches. Innovative control algorithms are vital for the diameter precision enhancement. We investigate the significance of recurrent deterministic policy gradient (RDPG) on diameter control. RDPG consists of deep deterministic policy gradient (DDPG) and recurrent neural network (RNN) where DDPG is a model-free reinforcement learning algorithm for continuous control and RNN for addressing partial observation. The impact of RNN variants selection on control performance is further discussed. Results illustrate that the application of RNN substantially solves the partial observation and varying RNN variants play a positive role in diameter control, but bidirectional gated recurrent unit (BiGRU) reaches the best accuracy. Comparison experiment results indicate that RDPG is able to learn reasonable control strategies and achieves significantly more accurate and robust diameter control in contrast with the existing methods.
更多查看译文
关键词
Optical fiber,Diameter control,Recurrent deterministic policy gradient,Deep reinforcement learning
AI 理解论文
溯源树
样例
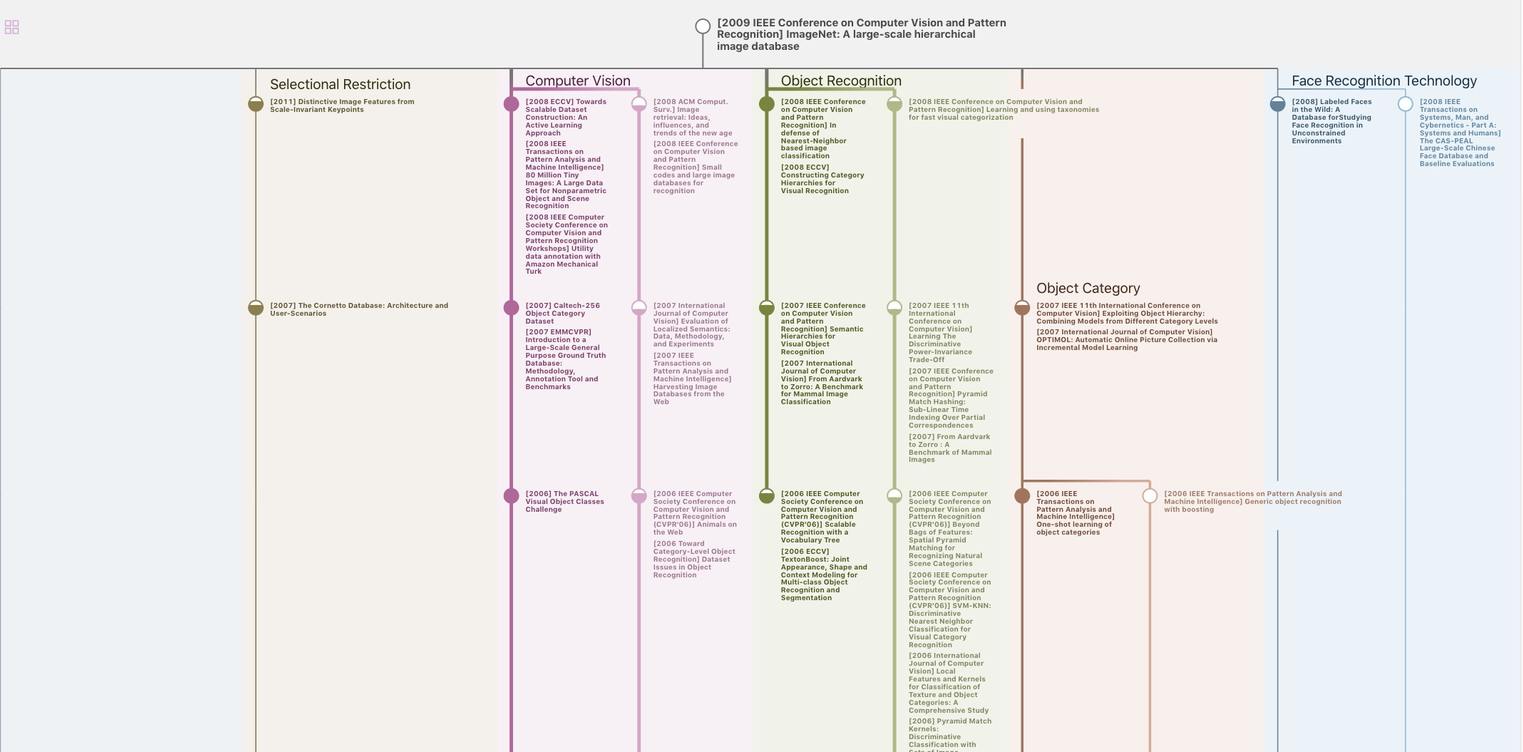
生成溯源树,研究论文发展脉络
Chat Paper
正在生成论文摘要