Accuracy Analysis of Physics-Informed Neural Networks for Approximating the Critical SQG Equation
CoRR(2024)
摘要
We systematically analyze the accuracy of Physics-Informed Neural Networks (PINNs) in approximating solutions to the critical Surface Quasi-Geostrophic (SQG) equation on two-dimensional periodic boxes. The critical SQG equation involves advection and diffusion described by nonlocal periodic operators, posing challenges for neural network-based methods that do not commonly exhibit periodic boundary conditions. In this paper, we present a novel approximation of these operators using their nonperiodic analogs based on singular integral representation formulas and use it to perform error estimates. This idea can be generalized to a larger class of nonlocal partial differential equations whose solutions satisfy prescribed boundary conditions, thereby initiating a new PINNs theory for equations with nonlocalities.
更多查看译文
AI 理解论文
溯源树
样例
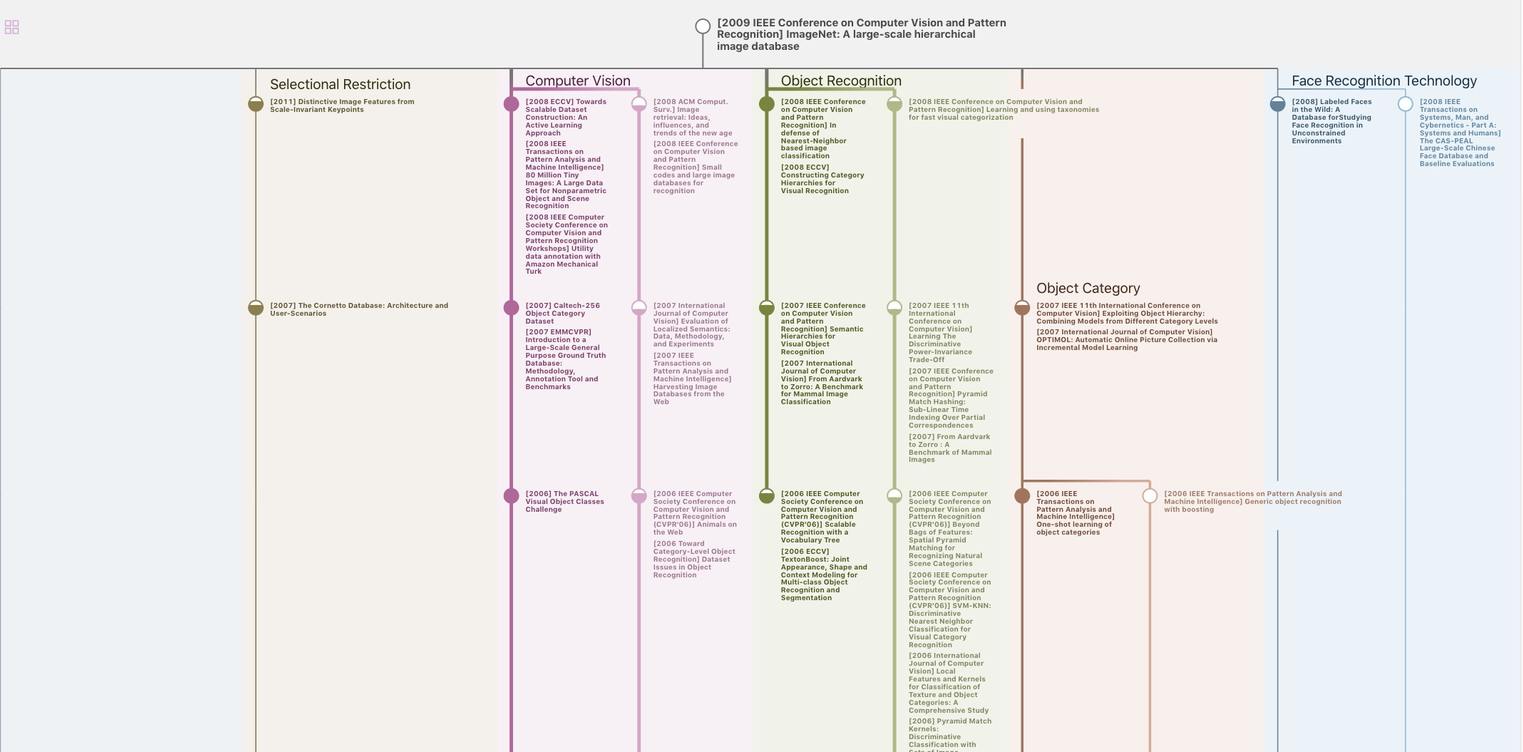
生成溯源树,研究论文发展脉络
Chat Paper
正在生成论文摘要