Investigating Training Strategies and Model Robustness of Low-Rank Adaptation for Language Modeling in Speech Recognition
CoRR(2024)
摘要
The use of low-rank adaptation (LoRA) with frozen pretrained language models (PLMs) has become increasing popular as a mainstream, resource-efficient modeling approach for memory-constrained hardware. In this study, we first explore how to enhance model performance by introducing various LoRA training strategies, achieving relative word error rate reductions of 3.50\% on the public Librispeech dataset and of 3.67\% on an internal dataset in the messaging domain. To further characterize the stability of LoRA-based second-pass speech recognition models, we examine robustness against input perturbations. These perturbations are rooted in homophone replacements and a novel metric called N-best Perturbation-based Rescoring Robustness (NPRR), both designed to measure the relative degradation in the performance of rescoring models. Our experimental results indicate that while advanced variants of LoRA, such as dynamic rank-allocated LoRA, lead to performance degradation in $1$-best perturbation, they alleviate the degradation in $N$-best perturbation. This finding is in comparison to fully-tuned models and vanilla LoRA tuning baselines, suggesting that a comprehensive selection is needed when using LoRA-based adaptation for compute-cost savings and robust language modeling.
更多查看译文
AI 理解论文
溯源树
样例
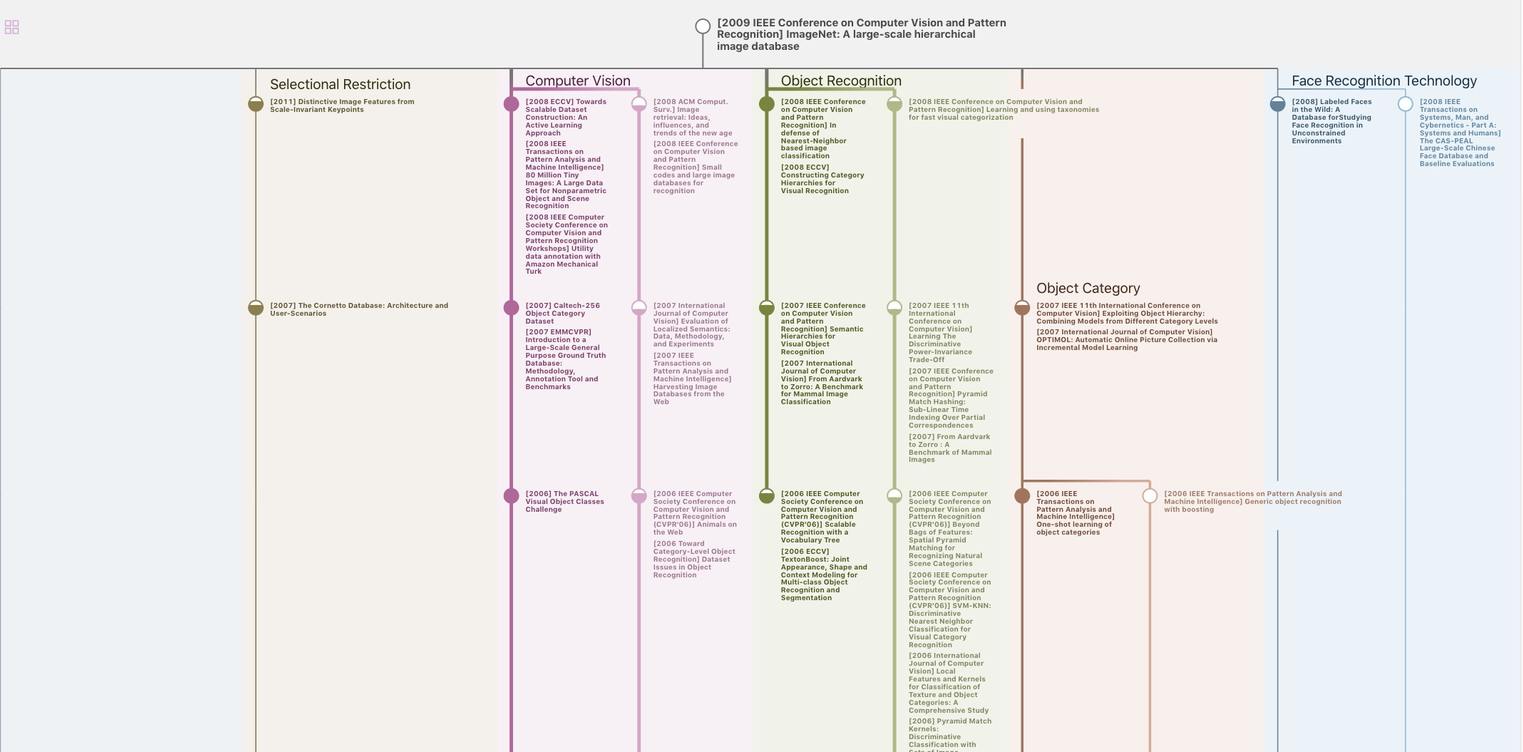
生成溯源树,研究论文发展脉络
Chat Paper
正在生成论文摘要