Attack and Defense Analysis of Learned Image Compression
arXiv (Cornell University)(2024)
摘要
Learned image compression (LIC) is becoming more and more popular these yearswith its high efficiency and outstanding compression quality. Still, thepracticality against modified inputs added with specific noise could not beignored. White-box attacks such as FGSM and PGD use only gradient to computeadversarial images that mislead LIC models to output unexpected results. Ourexperiments compare the effects of different dimensions such as attack methods,models, qualities, and targets, concluding that in the worst case, there is a61.55attack. To improve their robustness, we conduct adversarial training by addingadversarial images into the training datasets, which obtains a 95.52in the R-D cost of the most vulnerable LIC model. We further test therobustness of H.266, whose better performance on reconstruction quality extendsits possibility to defend one-step or iterative adversarial attacks.
更多查看译文
关键词
Image Forgery Detection,JPEG Compression,Adversarial Examples
AI 理解论文
溯源树
样例
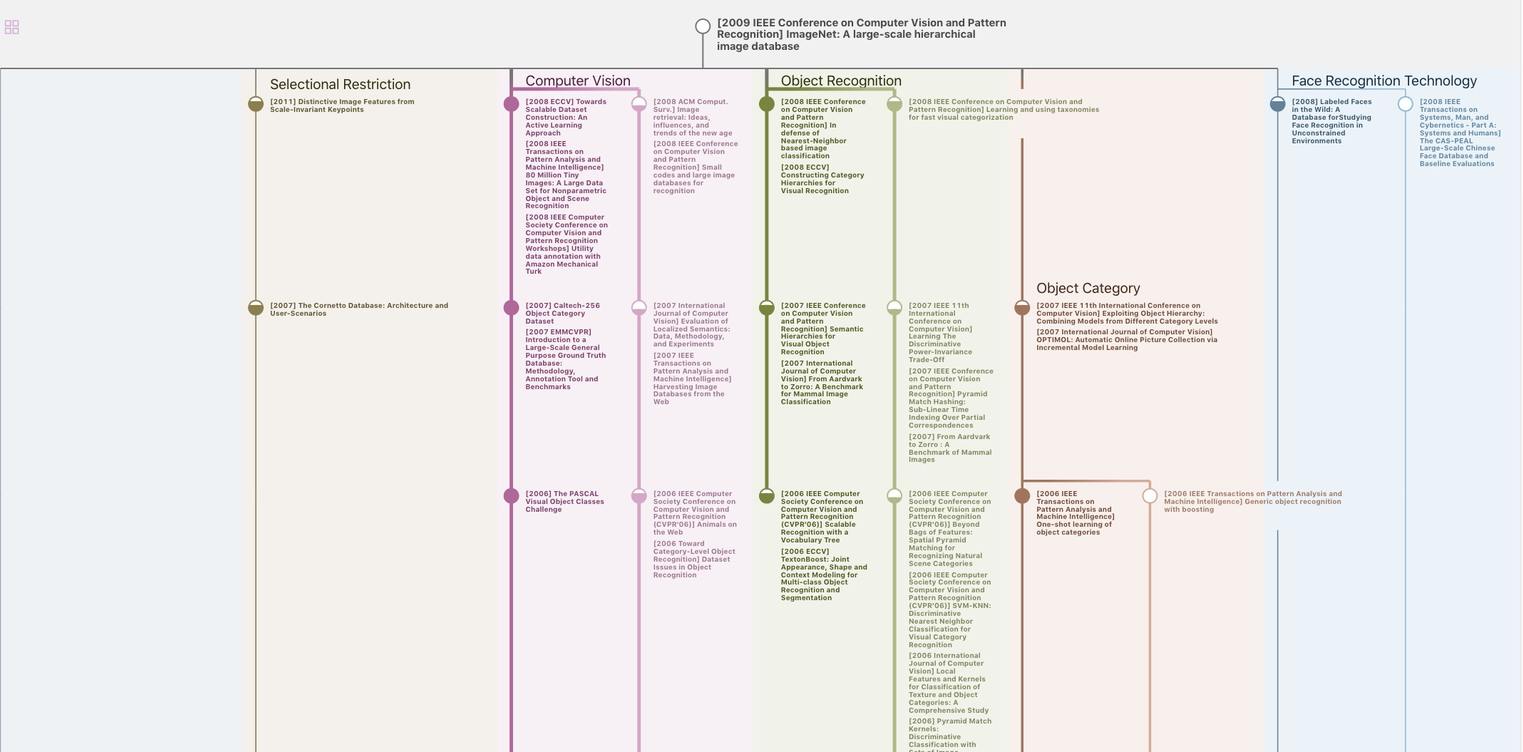
生成溯源树,研究论文发展脉络
Chat Paper
正在生成论文摘要