A digital twin for smart manufacturing of structural composites by liquid moulding
The International Journal of Advanced Manufacturing Technology(2024)
摘要
In this work, the authors present a digital twin (DT) to analyse the manufacturing process of structural composites by using resin transfer moulding (RTM). During RTM, a dry textile preform is impregnated with a polymer resin injected in a closed mould. RTM is one of the most used production methods for high-performance structural composites. The DT is focused on detecting in-homogeneous resin flow produced by race-tracking channels that divert resin flow to the outlet gates of the mould, producing dry spots and lack of impregnation. The DT core contains two surrogate models based on encoder/decoder deep learning architectures, providing the fast/accurate response necessary for interrogation during manufacturing. The first surrogate acts as the disturbance detector, providing the on-the-fly representation of the fabric permeability with the only information gathered by a set of five pressure sensors distributed over the mould surface. The second offers real-time representation of a set of quantities of interests (QoI): namely, the flow progress and the pressure field inside the mould. Training of both surrogates was performed with synthetic data generated by high-fidelity multi-physics simulations of the flow progress in a porous preform by following Darcy’s law. Errors in the pressure field predictions of the surrogates are lower than 1 % with consultation time <50 ms, enabling encapsulation in the digital twin. The DT performance was evaluated by comparing the response against a set of RTM experiments for different race-tracking scenarios. The two most relevant novelties of the work are the use of the concept of the instantiated DT, which provides information on the current state of the process from data provided by a network of distributed sensors, and that this DT has been trained exclusively with synthetic data from multiphysics simulations while evaluated against experimental data from injection tests.
更多查看译文
关键词
Composite materials,Digital twins,Liquid moulding,Surrogate models,Deep learning
AI 理解论文
溯源树
样例
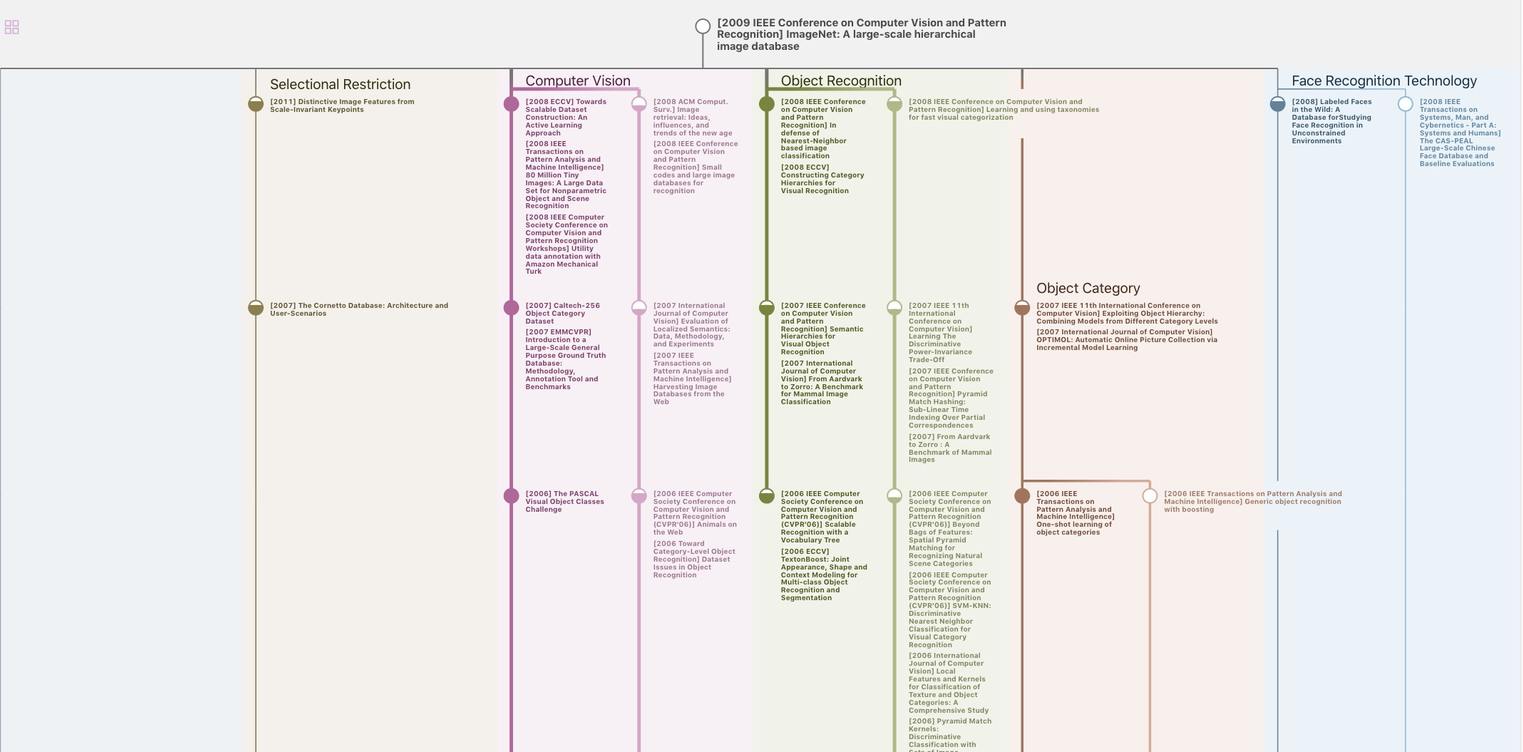
生成溯源树,研究论文发展脉络
Chat Paper
正在生成论文摘要