Benchmarking Robustness of Deep Neural Networks in Semantic Segmentation of Fluorescence Microscopy Images
crossref(2024)
摘要
Abstract Fluorescence microscopy (FM) is an important and widely adopted biological imaging technique. Segmentation is generally the first step in quantitative analysis of FM images. Although deep neural networks (DNNs) have become the state-of-the-art tools for image segmentation, their performance on natural images may collapse under certain image corruptions or adversarial attacks. This poses real risks to their deployment in real-world applications. Although the robustness of DNN models in segmenting natural images has been studied extensively, their robustness in segmenting FM images remains poorly understood. To address this deficiency, we have developed an assay that benchmarks robustness of DNN segmentation models using both realistic synthetic and real FM images with precisely controlled corruptions or adversarial attacks. Specifically, we have benchmarked robustness of ten representative models such as DeepLab and Vision Transformer in semantic segmentation of FM images. We find that models with good robustness on natural images may perform poorly on FM images. We also find new robustness properties of DNN models and new connections between their corruption robustness and adversarial robustness. Based on comprehensive benchmarking of robustness of the selected models, we make specific recommendations on selection and design of robust models for segmentation of FM images.
更多查看译文
AI 理解论文
溯源树
样例
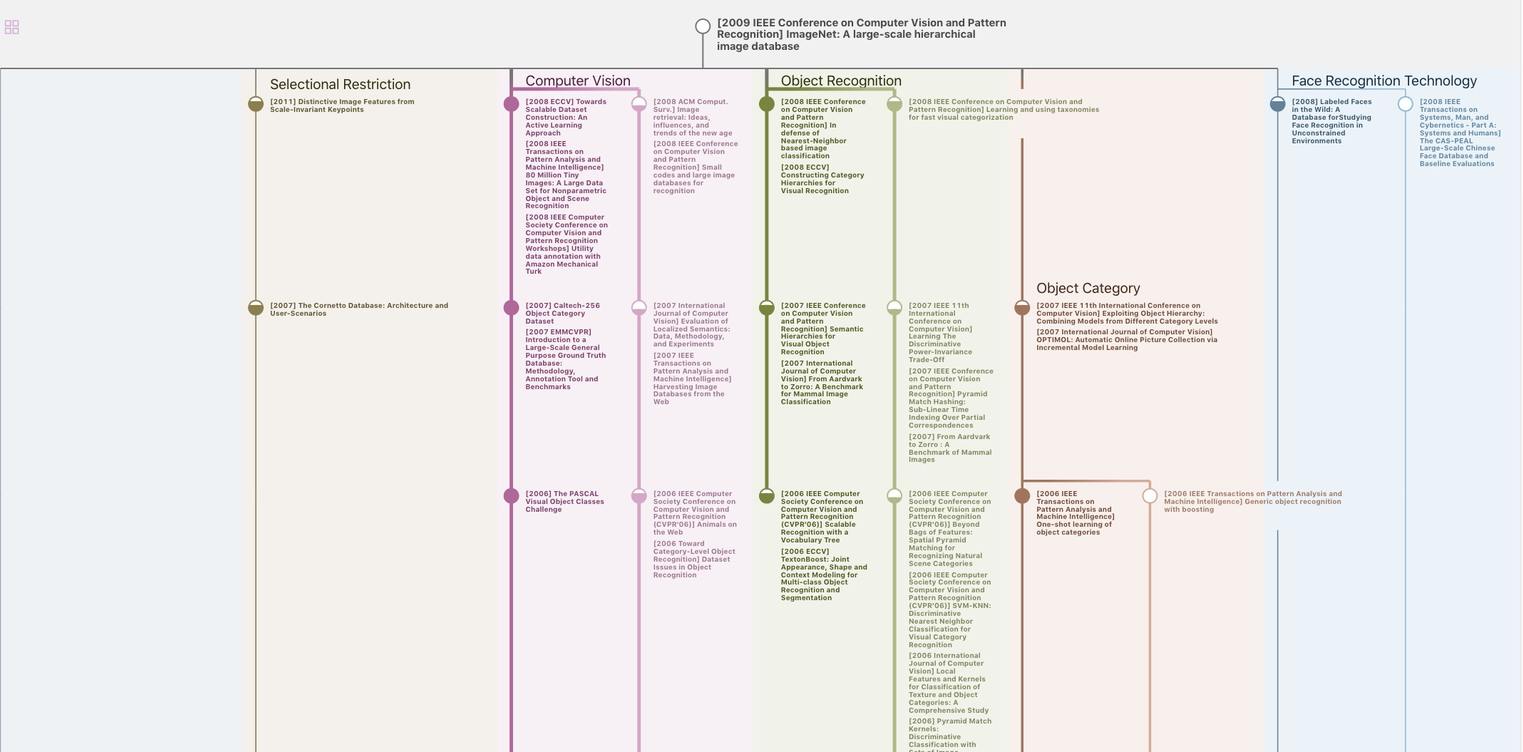
生成溯源树,研究论文发展脉络
Chat Paper
正在生成论文摘要