Robust Recognition of Speaker Emotion With Difference Feature Extraction Using a Few Enrollment Utterances.
2023 IEEE Automatic Speech Recognition and Understanding Workshop (ASRU)(2023)
摘要
This paper presents a novel approach to derive robust representations for speech emotion recognition by extracting speaker-independent features with the help of a speaker-dependent Gaussian mixture model (GMM). Since emotions are subjective and can vary greatly due to speaker behavior, incorporating speaker representations used for speaker verification tasks, such as x-vectors in past studies, have shown to improve the performance of speech emotion recognition, though they are not extracting speaker independent features. In this paper, we propose to derive embeddings that normalize speaker influence in the form of I-vectors, derived using a universal background model (UBM) trained only using the neutral emotion utterances from a single target speaker and an utterance-wise GMM also trained from the same speaker. We show through experiments on three datasets, that the proposed representations outperform methods that employ conventional x-vectors (which are not speaker-independent features) by approx. $3 \%$ absolute on average using as little as 4 enrollment utterances from the target speaker.
更多查看译文
关键词
Speech emotion recognition,Speech enrollment,GMM
AI 理解论文
溯源树
样例
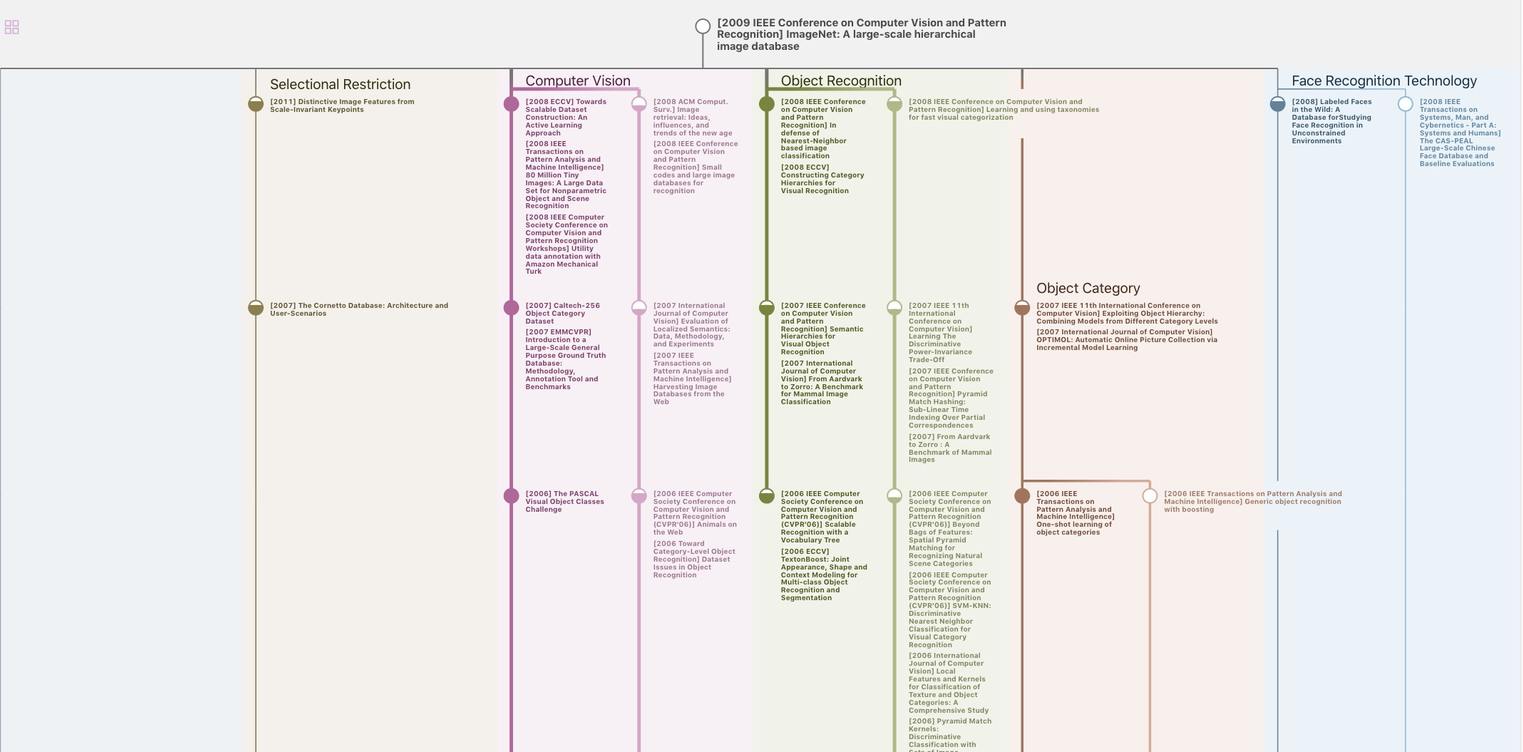
生成溯源树,研究论文发展脉络
Chat Paper
正在生成论文摘要