Predicting Protein-Ligand Binding Affinity with Multi-Scale Structural Features.
2023 IEEE International Conference on Bioinformatics and Biomedicine (BIBM)(2023)
摘要
Predicting protein-ligand binding affinity is important in areas such as drug discovery, gene regulation and signal transduction. The DTA(Drug-Target Affinity) method based on protein structure can not only effectively compensates for the lack of binding information, but also more in line with real biological processes. Although the structure-based DTA methods have achieved good performance, the existing methods still have the problem of only considering single-scale structural features and ignoring multi-scale structural features. In order to solve this problem, we propose the MSSDTA (Multi-Scale Structural Representation Drug-Target Affinity Prediction), which extracts multi-scale protein features by integrating the surface node features and structural node features of proteins. At the same time, the drug representation network is used to fuse the 2D molecular structure characteristics and chemical characteristics of the drug to effectively distinguish the drug molecules with similar planar structures. Finally, the affinity prediction network is used to generate protein-ligand binding affinity scores. We verify the performance of this model on the PDBbind v.2019 dataset. The experimental results show that the proposed method achieves excellent performance.
更多查看译文
关键词
Protein-Ligand Binding Affinity,Multi-Scale Protein Features,Drug Representation Network,Affinity Prediction Network
AI 理解论文
溯源树
样例
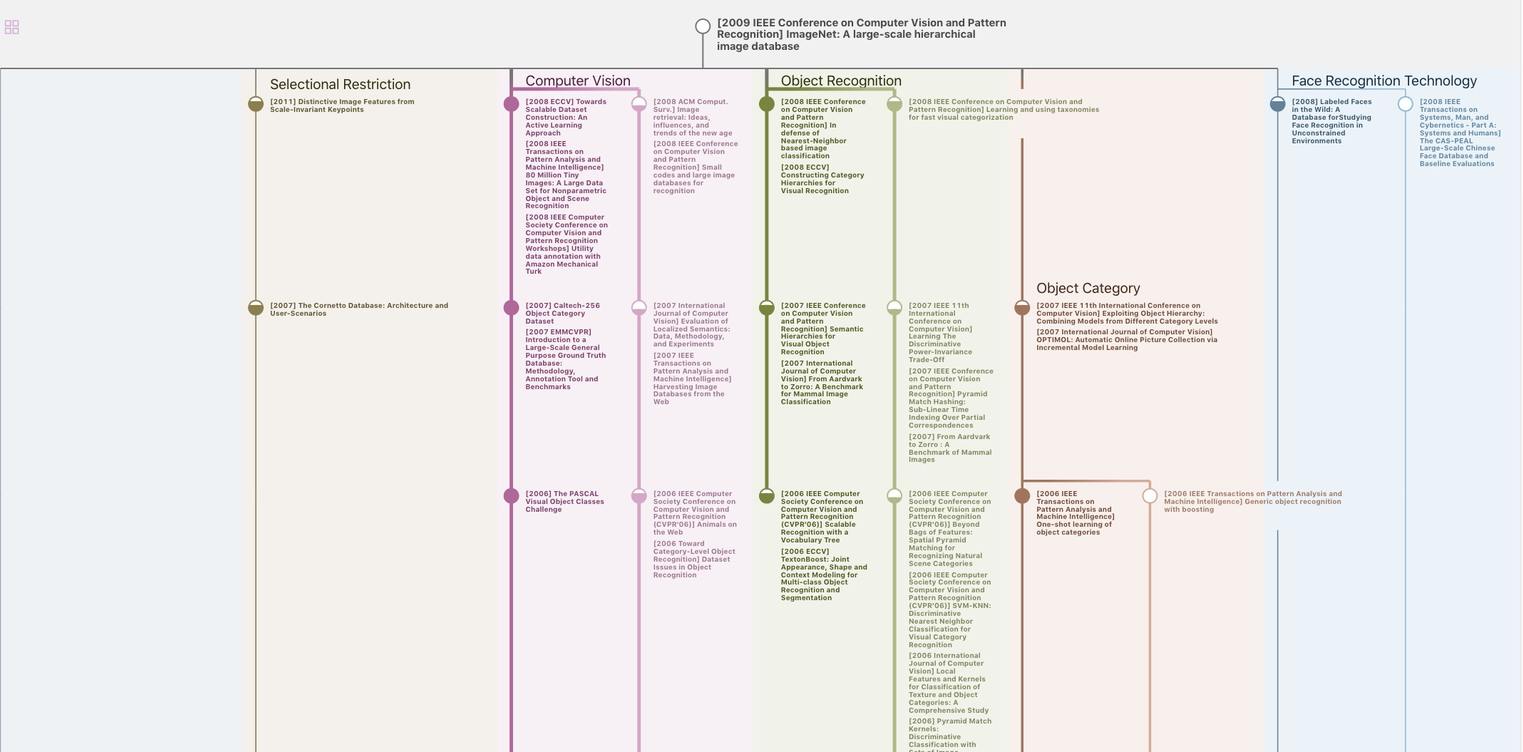
生成溯源树,研究论文发展脉络
Chat Paper
正在生成论文摘要