Radiology Report Generation via Structured Knowledge-Enhanced Multi-modal Attention and Contrastive Learning.
2023 IEEE International Conference on Bioinformatics and Biomedicine (BIBM)(2023)
摘要
The automated generation of radiology reports has attracted significant attention in the field of bioinformatics. Currently, the main limitations of this task include insufficient utilization of prior medical knowledge, lack of efficient knowledge fusion algorithms, and less distinctiveness between different generated reports. To address these issues, we propose a novel algorithm for radiology report generation, which includes Structured Knowledge-Enhanced Multi-modal Attention (SKEMA) and Dual-Branch Contrastive Learning (DBCL) for the first time. SKEMA aims to effectively bridge the gap between visual and prior knowledge by leveraging the high-order adjacency matrix of the knowledge graph to weightedly fuse image features and knowledge features. We enhance both features through masking, and use the original features and augmented features as positive and negative samples in the dual-branch contrastive learning (DBCL). DBCL increases the differences between positive and negative samples to avoid generating templated results, and enhances the robustness of the model. Finally, we conducted experiments to demonstrate the effectiveness of our model on two public radiology datasets, IU-Xray and MIMIC-CXR. Our model outperformed previous baseline methods on both datasets and achieved excellent evaluation scores.
更多查看译文
关键词
Radiology Report Generation,Knowledge Graph,Contrastive Learning,Multi-modal Fusion
AI 理解论文
溯源树
样例
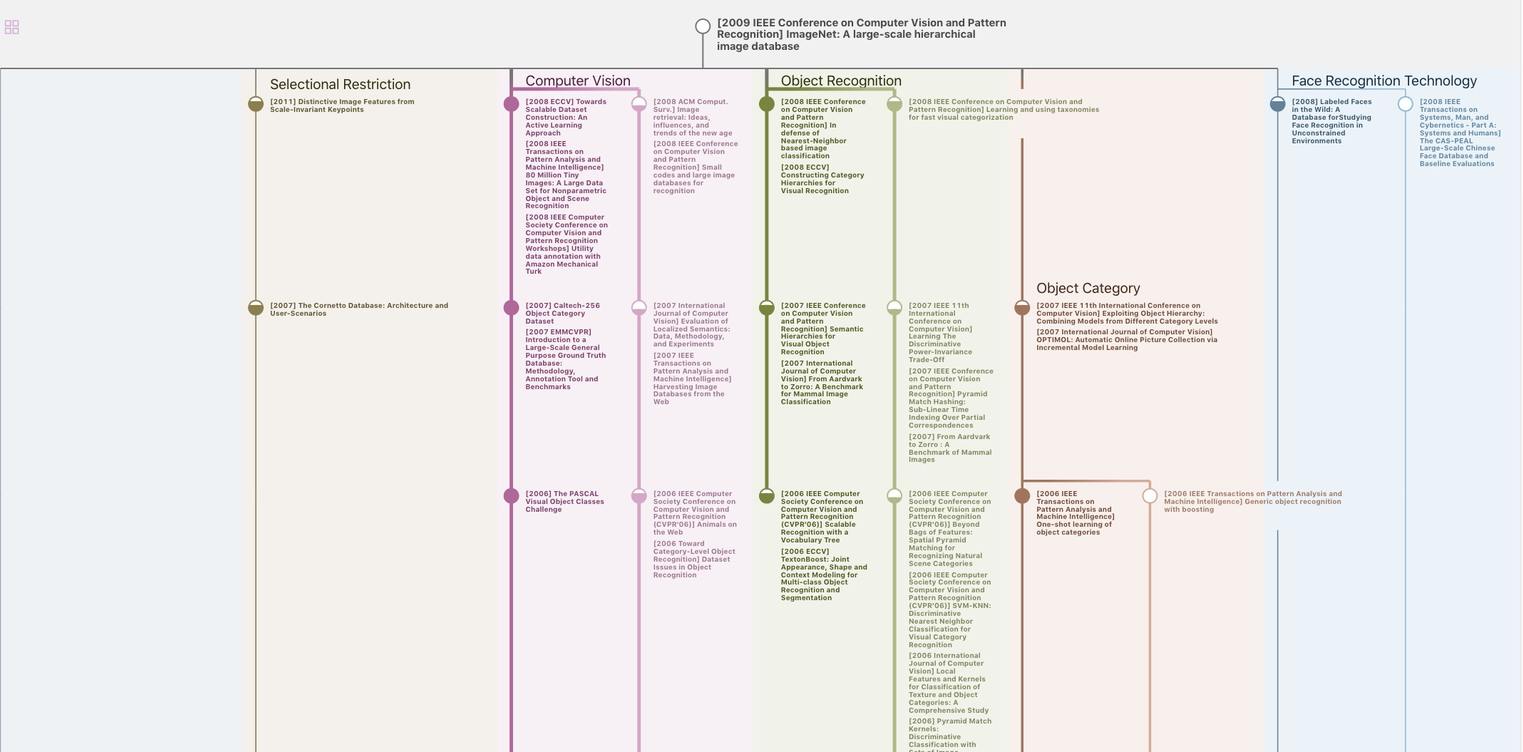
生成溯源树,研究论文发展脉络
Chat Paper
正在生成论文摘要