Multimodal-AIR-BERT: A Multimodal Pre-trained Model for Antigen Specificity Prediction in Adaptive Immune Receptors.
2023 IEEE International Conference on Bioinformatics and Biomedicine (BIBM)(2023)
摘要
The in silico prediction of antigen specificity in adaptive immune receptors (AIRs), such as T-cell receptors (TCRs), is essential for understanding immunological processes and developing targeted therapies. The V(D)J gene rearrangement is a critical biological process that generates diversity in amino acid (AA) sequences in antigen-binding regions, enabling AIRs to recognize a wide range of antigens from various pathogens and "altered self cells" observed in cancers. The huge diversity of AIRs presents a significant challenge to existing computational methods for antigen specificity prediction. To address these complexities, we introduce Multimodal-AIR-BERT, a novel multimodal pre-trained model aimed at enhancing the prediction of antigen-binding specificity in TCRs. It comprises a pre-trained sequence encoder, a gene encoder, and a multimodal fusion module with gating-based attention and tensor fusion to calibrate and integrate the V(D)J gene and AA sequence features of TCRs, thereby generating more informative representations. The integration of V(D)J gene information, which provides insights often unobtainable from sequences alone, benefits Multimodal-AIR-BERT in performance enhancement compared to its sequence-modality-only counterpart. Collectively, our work provides an advancement in the accurate prediction of antigen-binding specificity. As the precision of this specificity prediction improves, it can potentially pave the way for targeted immune therapies and deeper insights into the interactions within the immune system.
更多查看译文
关键词
antigen-binding specificity,T-cell receptors,deep learning,multi-modal learning,Computational immunology
AI 理解论文
溯源树
样例
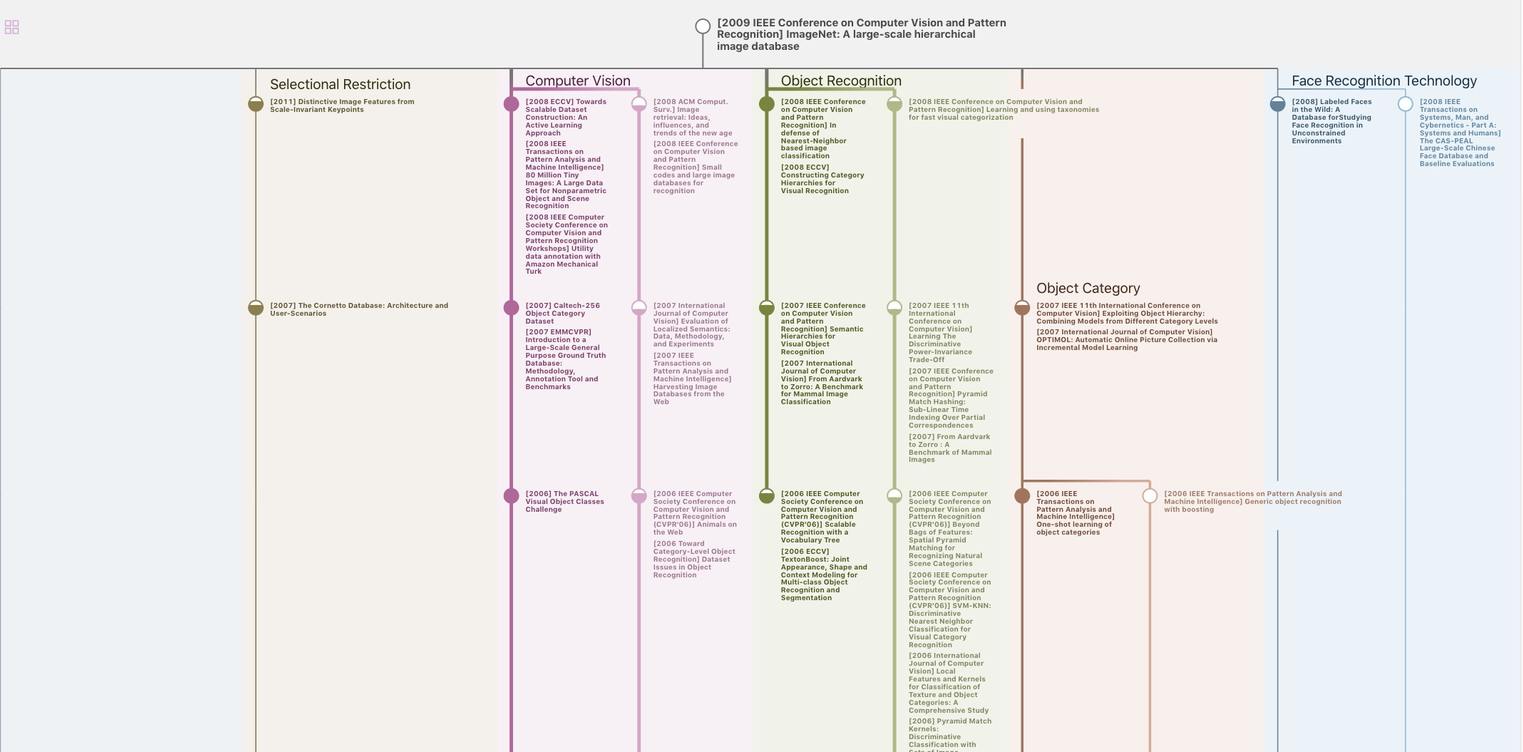
生成溯源树,研究论文发展脉络
Chat Paper
正在生成论文摘要