Non-rigid Medical Image Registration Based on Unsupervised Self-driven Prior Fusion.
2023 IEEE International Conference on Bioinformatics and Biomedicine (BIBM)(2023)
摘要
Deformable image registration is a basic building block in intelligent bioinformatical analysis and biomedicine systems. With the rapid development of deep learning, the community has witnessed a great leap via this effective data-driven technique. Recently, the Vision Transformer, famous by its long-range modeling ability, has been successfully used in the field of medical image registration. However, the existing ViT based techniques are deemed to have certain limitations. Firstly, these methods often transplanted the transformer module directly into the networks, while did not dive deeper to explore its compatibility to practical registration tasks. Moreover, self-attention’s relatively rigid all-to-all patching strategy may cause undesirable discontinuity effect to the spatial calculation. To address these issues, we propose a novel medical image registration framework based on an efficient image prior learning and fusion mechanism. Unlike the existing prior-based registration methods, our model is capable of learning task-specific saliency priors, without the need of hand-crafted features, or heavy-loaded auxiliary tasks, or pre-acquired expensive annotations. Then, followed by a multi-scale patch embedding module, the self-driven saliency prior is integrated into a ViT block with an active feature fusion mechanism, to further expand our network’s structural learning abilities. Extensive experiments on multiple data sets have demonstrated the superior quality of the proposed framework. We believe this plug-and-play model will bring about more application potentials to the community (Project webpage: https://github.com/raincity212/SPF-Net).
更多查看译文
关键词
Medical image registration,Self-driven Prior,Fusion transformer
AI 理解论文
溯源树
样例
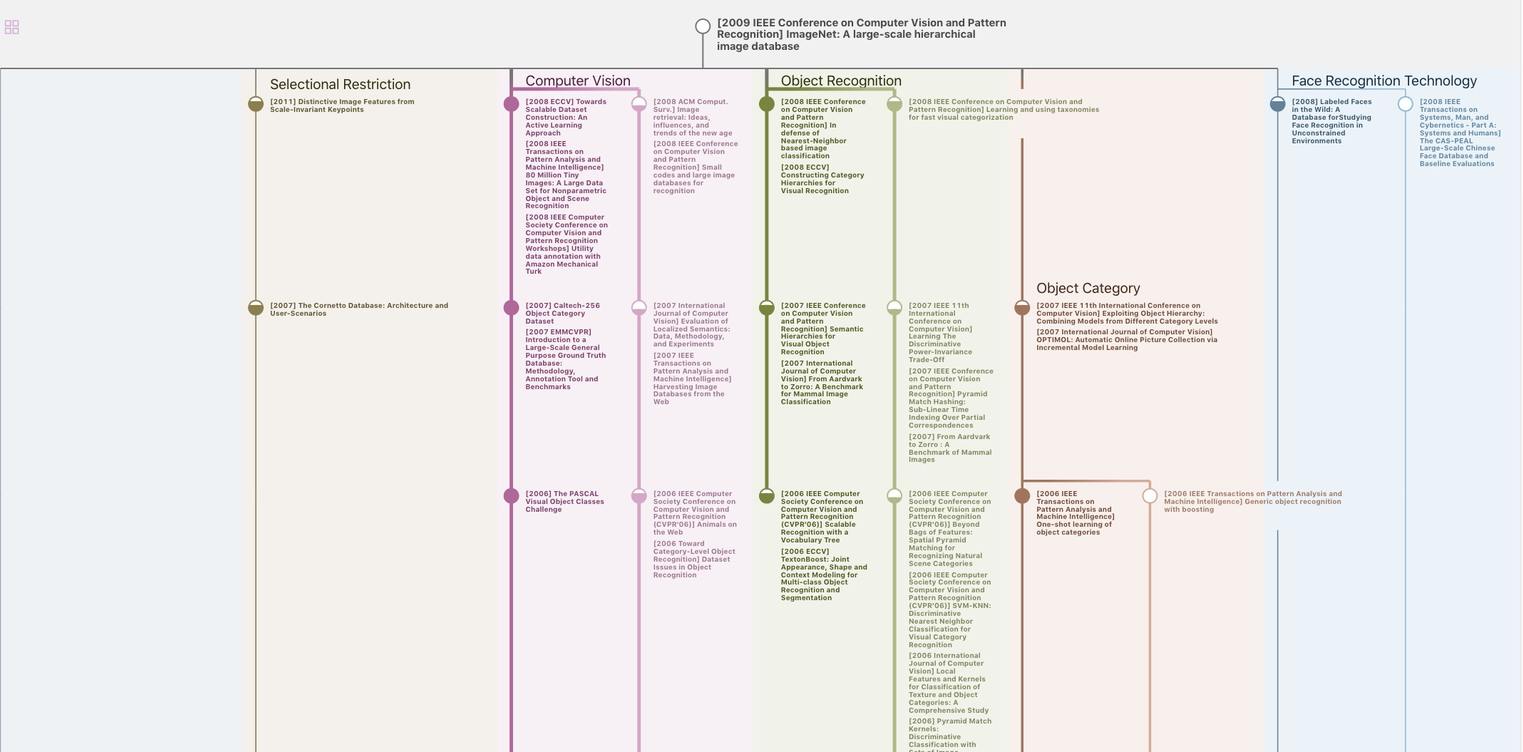
生成溯源树,研究论文发展脉络
Chat Paper
正在生成论文摘要