SegmA: Residue Segmentation of cryo-EM density maps.
2023 IEEE International Conference on Bioinformatics and Biomedicine (BIBM)(2023)
摘要
We introduce SegmA, a novel method for cryo-EM map visualization. By coloring voxels based on their amino acids, SegmA enhances both manual and automated modeling. This coloring approach also serves as a scoring function, extending its utility to de-novo structure modeling.SegmA’s algorithm is a cascade of group rotational equivariant convolutional neural networks (G-CNNs), enabling it to better handle the orientation variability of cryo-EM maps. During the training procedure of SegmA, an iterative co-training algorithm is utilized to filter out poorly labeled samples from the training dataset. SegmA also detects regions of low-confidence labeling, with amino acid detection accuracy increasing to 80% after removing these regions.Beyond 3D visualization, SegmA also has additional applications such as prediction of amino acid centers of mass, scoring of the fitting of a protein structural template into a cryo-EM map, and de-novo modeling of a protein complex.
更多查看译文
关键词
Protein structure prediction,cryo-EM,Machine Learning,Docking
AI 理解论文
溯源树
样例
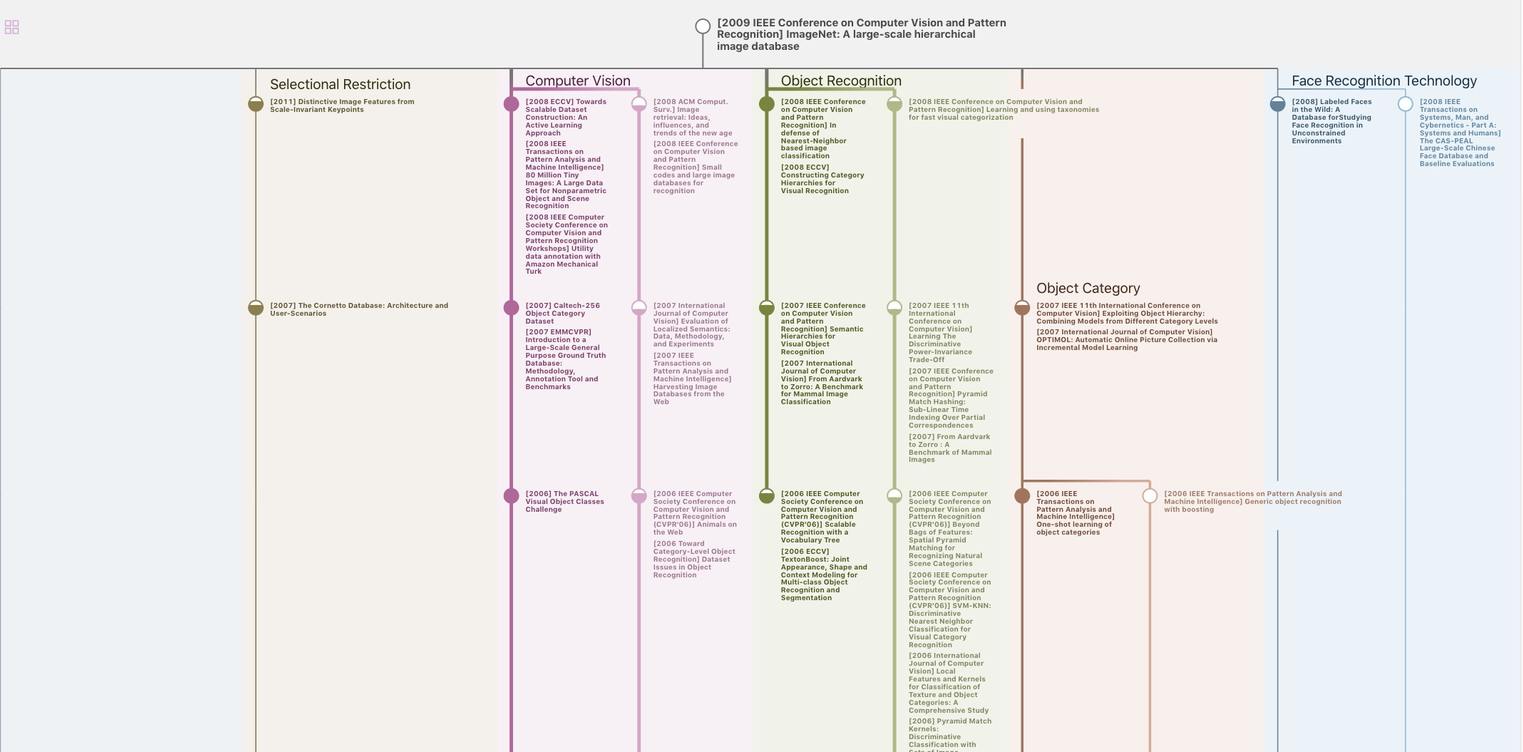
生成溯源树,研究论文发展脉络
Chat Paper
正在生成论文摘要