Exploring Parameter-Efficient Fine-Tuning of a Large-Scale Pre-Trained Model for scRNA-seq Cell Type Annotation.
2023 IEEE International Conference on Bioinformatics and Biomedicine (BIBM)(2023)
摘要
Accurate identification of cell types is a pivotal and intricate task in scRNA-seq data analysis. Recently, significant strides have been made in cell type annotation of scRNA-seq data using pre-trained language models (PLMs). This method has surmounted the constraints of conventional approaches regarding precision, robustness, and generalization. However, the fine-tuning process of large-scale pre-trained models incurs substantial computational expenses. To tackle this issue, a promising avenue of research has emerged, proposing parameter-efficient fine-tuning techniques for PLMs. These techniques concentrate on fine-tuning only a small portion of the model parameters while attaining comparable performance. In this study, we extensively research parameter-efficient fine-tuning methods for scRNA-seq cell type annotation, employing scBERT as the backbone. We scrutinize the performance and compatibility of various parameter-efficient fine-tuning methodologies across multiple datasets. Through comprehensive analysis, we demonstrate the remarkable performance of parameter-efficient fine-tuning methods in cell type annotation. Hopefully, this study can inspire new thinking in analyzing scRNA-seq data.
更多查看译文
关键词
Single-cell RNA-seq,Cell type annotation,Parameter-efficient fine-tuning,Pre-trained language model
AI 理解论文
溯源树
样例
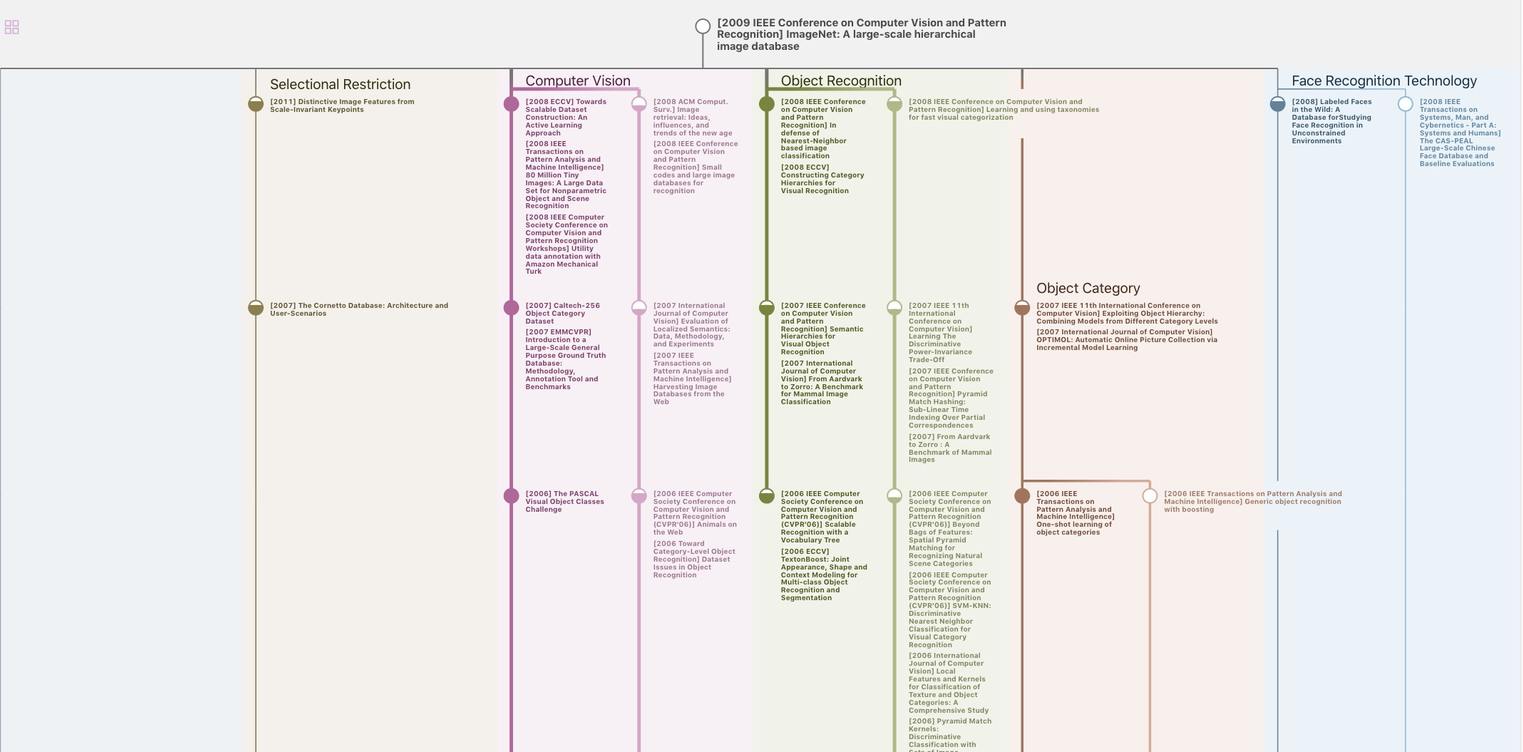
生成溯源树,研究论文发展脉络
Chat Paper
正在生成论文摘要