Protein function prediction using graph neural network with multi-type biological knowledge.
2023 IEEE International Conference on Bioinformatics and Biomedicine (BIBM)(2023)
摘要
Proteins play crucial roles in diverse biological functions, and accurately annotating their functions is essential for understanding cellular mechanisms and developing therapies for complex diseases. Computational methods have been proposed as alternatives to laborious experimental approaches. However, existing network-based methods focus on the protein-protein interaction (PPI) networks, while the proteins without interactions are ignored. To address this limitation, we propose a novel deep learning framework for protein function prediction, named PFP-GMB, which incorporates multi-type biological knowledge to consider the proteins not present in the PPI networks. PFP-GMB leverages a pre-trained protein language model to extract sequence representations. Moreover, PPIs and orthology relationships are used to generate functional related features via graph neural networks and attention mechanisms. Finally, these multi-type features are fused for protein function prediction. Compared to eight state-of-the-art methods, PFP-GMB outperforms all of them in terms of F-max and AUPR. The ablation studies further confirm the relevance and significance of the multi-type biological knowledge incorporated into PFP-GMB for protein function prediction.
更多查看译文
关键词
protein function,orthology network,PPI network,protein sequence,graph neural network
AI 理解论文
溯源树
样例
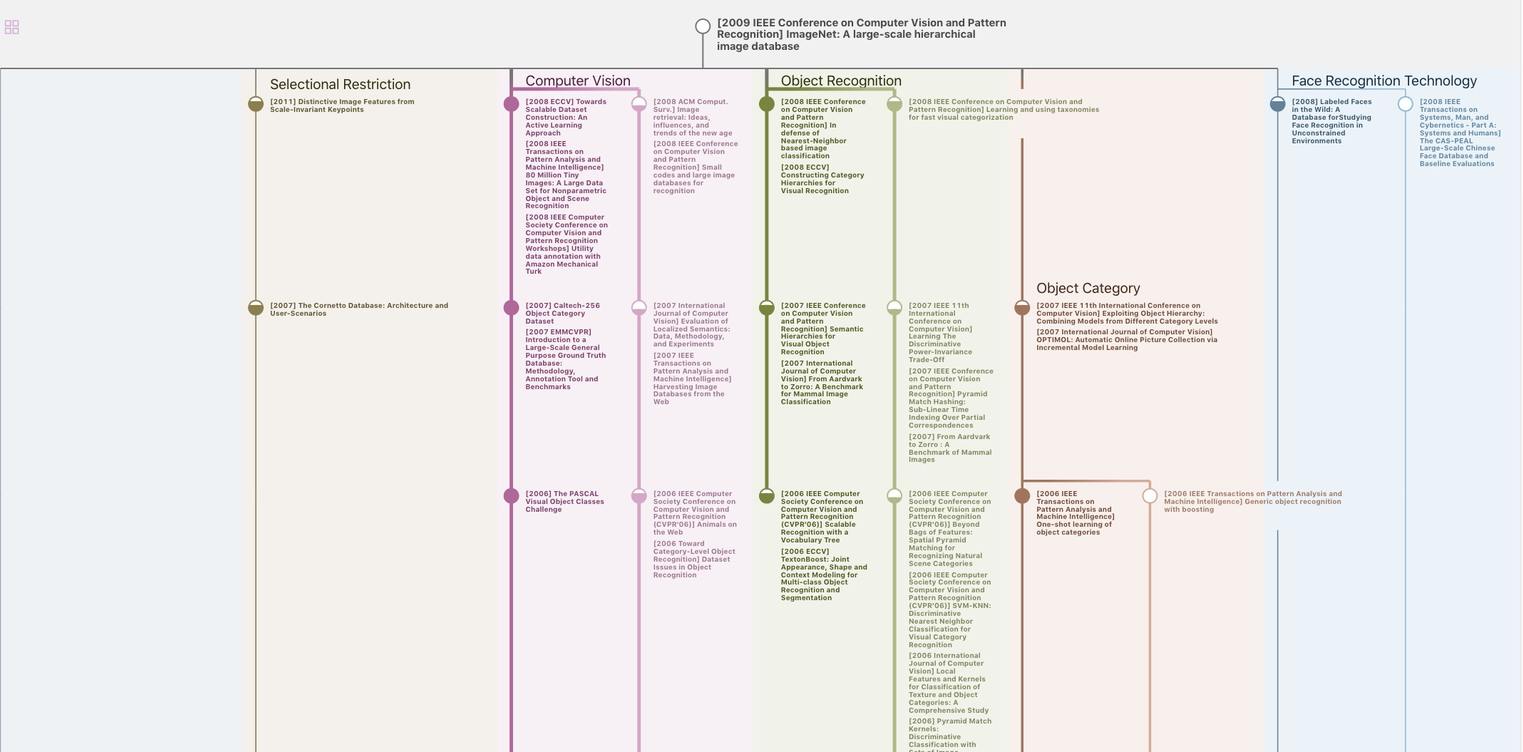
生成溯源树,研究论文发展脉络
Chat Paper
正在生成论文摘要