TS-DDD: A Two-Stage Training Strategy for Dialogue-based Disease Diagnosis.
2023 IEEE International Conference on Bioinformatics and Biomedicine (BIBM)(2023)
摘要
Dialogue-based automatic disease diagnosis has the potential to assist medical professionals in enhancing the accuracy and efficiency of diagnosis and treatment. Nonetheless, prior models grounded in reinforcement learning often exhibit a tendency to inquire about unrelated symptoms that hold limited relevance to the available symptom information within the constrained number of conversational exchanges. Consequently, these models may hastily assign a diagnosis to the patient. To address this issue, our paper draws inspiration from the diagnostic procedures employed by real-world physicians and introduces a novel two-stage training strategy termed TS-DDD for Dialogue-based Disease Diagnosis. In the initial stage, we pretrain two classifiers for symptom inquiry and disease prediction, separately. In the second stage, we devise a joint training framework that seamlessly integrates symptom inquiry, disease prediction, and decision-making processes. Extensive experiments are conducted and the results validate the effectiveness of our proposed TS-DDD model.
更多查看译文
关键词
Medical dialogue,Dialogue-based disease diagnosis,Pointer generation network,Two-stage training strategy
AI 理解论文
溯源树
样例
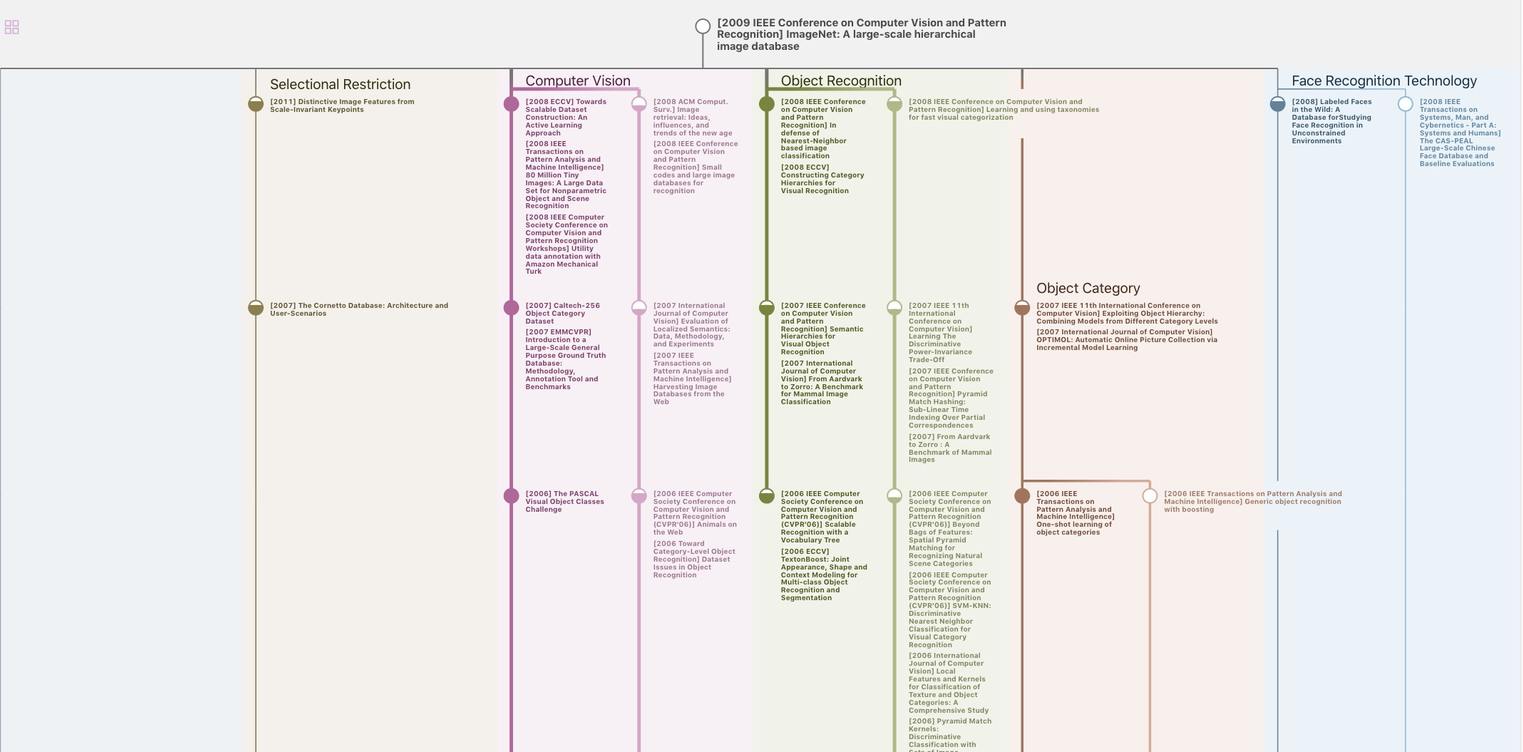
生成溯源树,研究论文发展脉络
Chat Paper
正在生成论文摘要