Accurately Identifying Muscle-Invasive Bladder Cancer from MRI via Weakly Supervised Learning.
2023 IEEE International Conference on Bioinformatics and Biomedicine (BIBM)(2023)
Abstract
Bladder cancer (BCa) is one of the most common malignancies in the world, which can be categorized into muscleinvasive (MIBC) and non-muscle-invasive (NMIBC). These two types of BCa must be treated differently, and thus it is essential to correctly distinguish MIBC and NMIBC patients preoperatively for adopting different treatment methods accordingly. Currently, the two types can be distinguished through MRI images by radiologists, but manual inspection is time and labor-consuming. Existing machine learning based methods attempt to free radiologists from manual inspection. However, they fail to take full advantage of image features and always require extra laborious refined manual labeling in addition to the classification labels. In this study, we propose a Tumor Staging and Localization Network (TSLNet) to perform preoperative non-invasive assessment of muscle invasion of BCa, which can automatically distinguish MIBC patients from NMIBC patients based on MRI T2-weighted images of BCa. The model adopts the weakly supervised learning method. Specifically, self-produced guidance is used as pixellevel segmentation pseudo labels for auxiliary supervision to extract basic features, and location-recognition based fine-grained image classification technology and inexact consistency labels are used for auxiliary supervision to extract fine-grained features. Moreover, the model can visualize the critical regions of the lesions, which can provide practical reference and a basis for clinicians’ clinical diagnosis. Experimental results show that the model achieves high AUC, accuracy, specificity, sensitivity, and F1-score, which is comparable to experienced clinicians.
MoreTranslated text
Key words
Bladder Cancer (BCa),Muscle-invasive,MRI T2-weighted image,Weakly Supervised Learning,Fine-grained Image ClassificationI
AI Read Science
Must-Reading Tree
Example
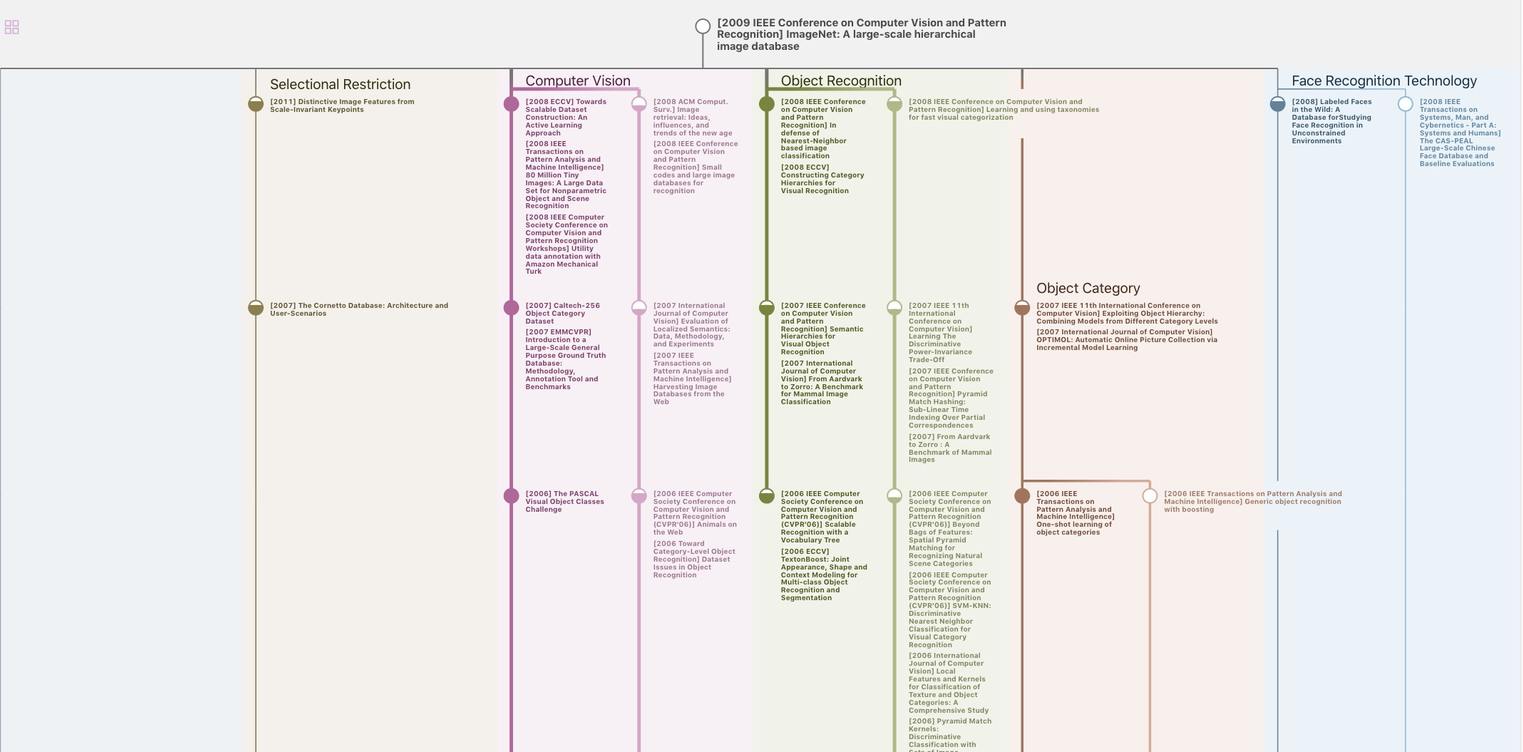
Generate MRT to find the research sequence of this paper
Chat Paper
Summary is being generated by the instructions you defined